Physics-informed Student’s t mixture regression model applied to predict mixed oil length
Journal of Pipeline Science and Engineering(2023)
Abstract
Real-time estimation of thelength of mixed oil in a multi-product pipeline is a critical task during batch transportation. In previous studies, various predictive models have been built while they merely depended on a single predictive model to fulfill the regression work, and model performance severely deteriorated with the presence of outliers. The Student’s t mixture regression (SMR) model can identify multimode characteristics and reduce the impact of outliers. However, ignorance of physics knowledge and the simplistic assumption of a linear relationship between variables in the SMR may lead to unsatisfactory performance. In addition, the possible singularity problem can make the SMR fails to work. Motivated by resolving these issues, this paper proposes a physics-informed SMR modeling method by integrating the physics knowledge and the SMR to develop a robust hybrid predictive model for predicting the mixed oil length in a multi-product pipeline. Case studies are carried out on the measured dataset to demonstrate the effectiveness and advantages of the proposed new modeling method compared to the model entirely based on the SMR method and two state-of-the-art predictive models.
MoreTranslated text
Key words
Mixed oil length, Multi-product pipeline, Student's t mixture regression model, Physics-data, Robustness
AI Read Science
Must-Reading Tree
Example
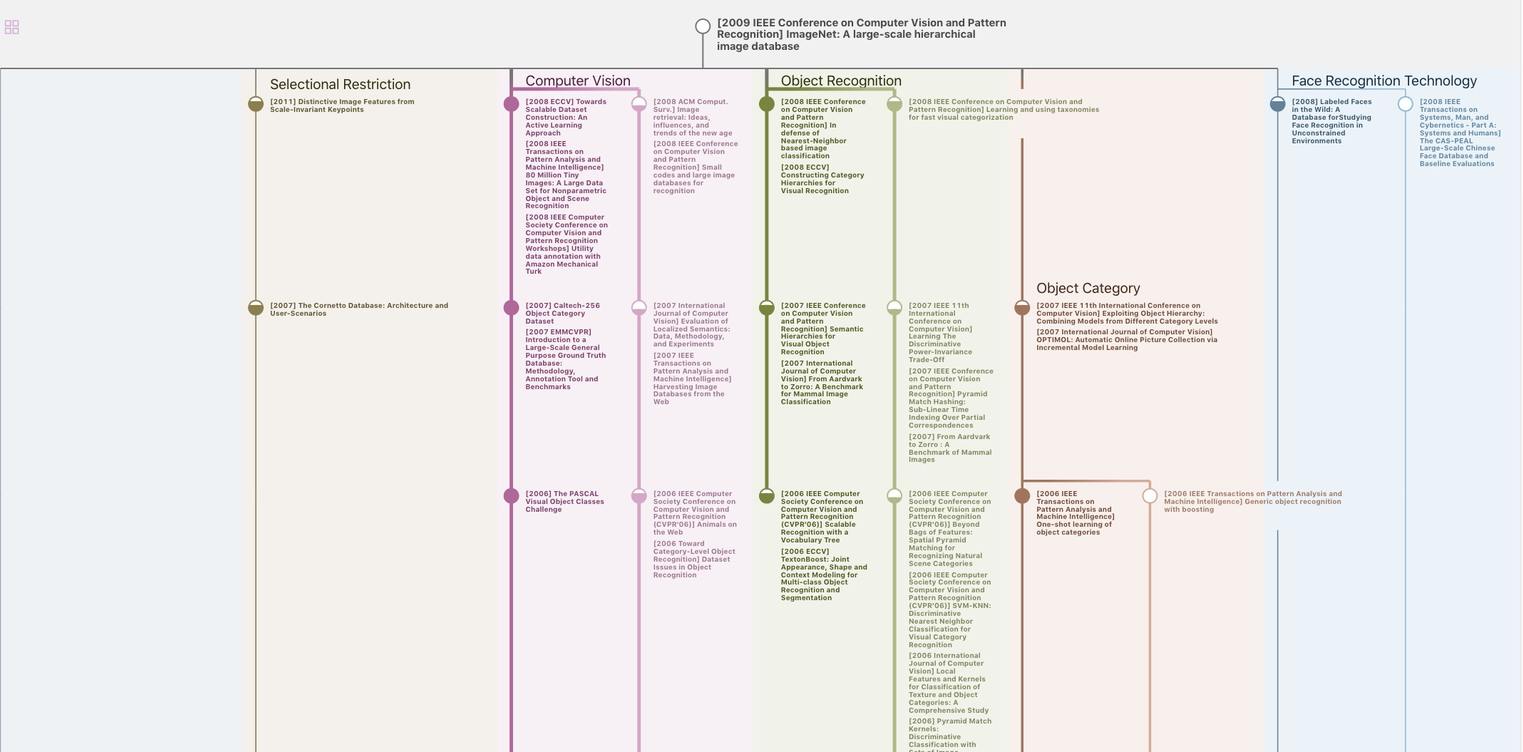
Generate MRT to find the research sequence of this paper
Chat Paper
Summary is being generated by the instructions you defined