Modelling fatigue life prediction of additively manufactured Ti-6Al-4V samples using machine learning approach
International Journal of Fatigue(2023)
摘要
•ML framework for fatigue life prediction of AM Ti-6Al-4V samples is proposed.•ANN, RFR and SVR models are used for fatigue life prediction.•Spearman’s rank correlation test is applied to identify insensitive features.•The LOOCV technique is employed in the optimization of the ML models.
更多查看译文
关键词
Selective laser melting (SLM), Ti-6Al-4V, Micro-computed tomography (mu CT), Fatigue life prediction, Machine learning (ML)
AI 理解论文
溯源树
样例
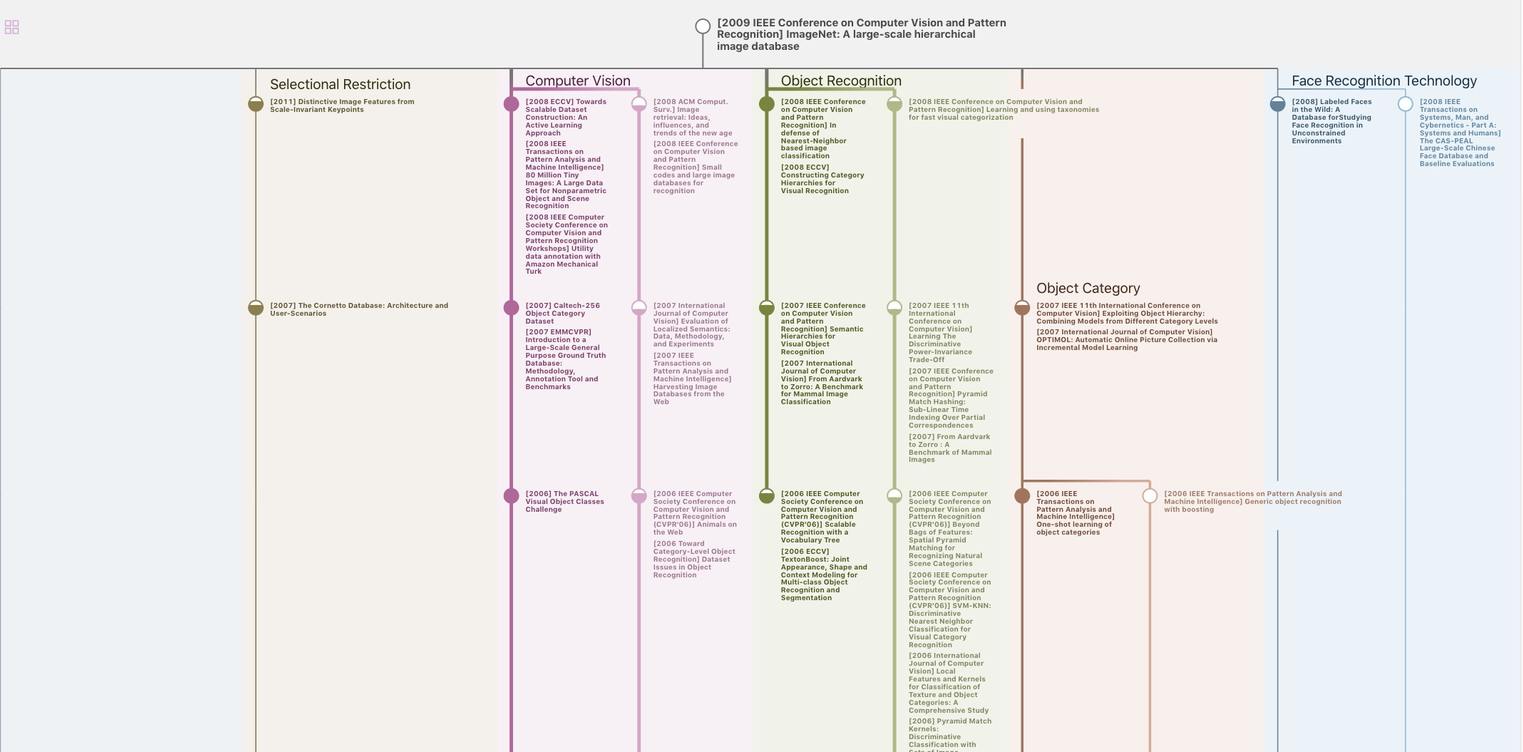
生成溯源树,研究论文发展脉络
Chat Paper
正在生成论文摘要