Stochastic Day-ahead operation of cascaded hydropower systems with Bayesian neural network-based scenario generation: A Portland general electric system study
International Journal of Electrical Power & Energy Systems(2023)
摘要
Coordinating water usage of cascaded dams along a river to serve electric power generation and other non-power needs is challenging, due to the volatile and time-variate streamflow, the tight hydrologic couplings between the dams, and the strict regulation of individual dams. With this, cascaded hydropower system (CHS) operators, such as Portland General Electric (PGE), usually adopt empirical strategies to sidestep these difficulties, but at the expense of water usage inefficiency and economic losses. Motived by this, a stochastic optimization-based dayahead operation model of CHSs is developed to determine scenario-independent hourly forebay levels of individual dams, which could assist operators in effectively utilizing available water resources against inflow uncertainties and maximizing the profit of hydropower. A common penstock effect model is also integrated to accurately capture the physical operating characteristics of CHSs. Moreover, a data-driven Bayesian neural network (BNN)-based scenario generation model is seamlessly connected to the stochastic day-ahead operation model for preparing water inflow scenarios of the impending operating day. Leveraging actual operation data of the PGE's Round Butte-Pelton CHS, numerical simulations demonstrate that the day-ahead operation schedules out of the proposed approach could deliver more efficient water usage and higher economic benefits than the PGE's current practice.
更多查看译文
关键词
Hydropower,Bayesian neural network,Stochastic optimization,Scenario generation
AI 理解论文
溯源树
样例
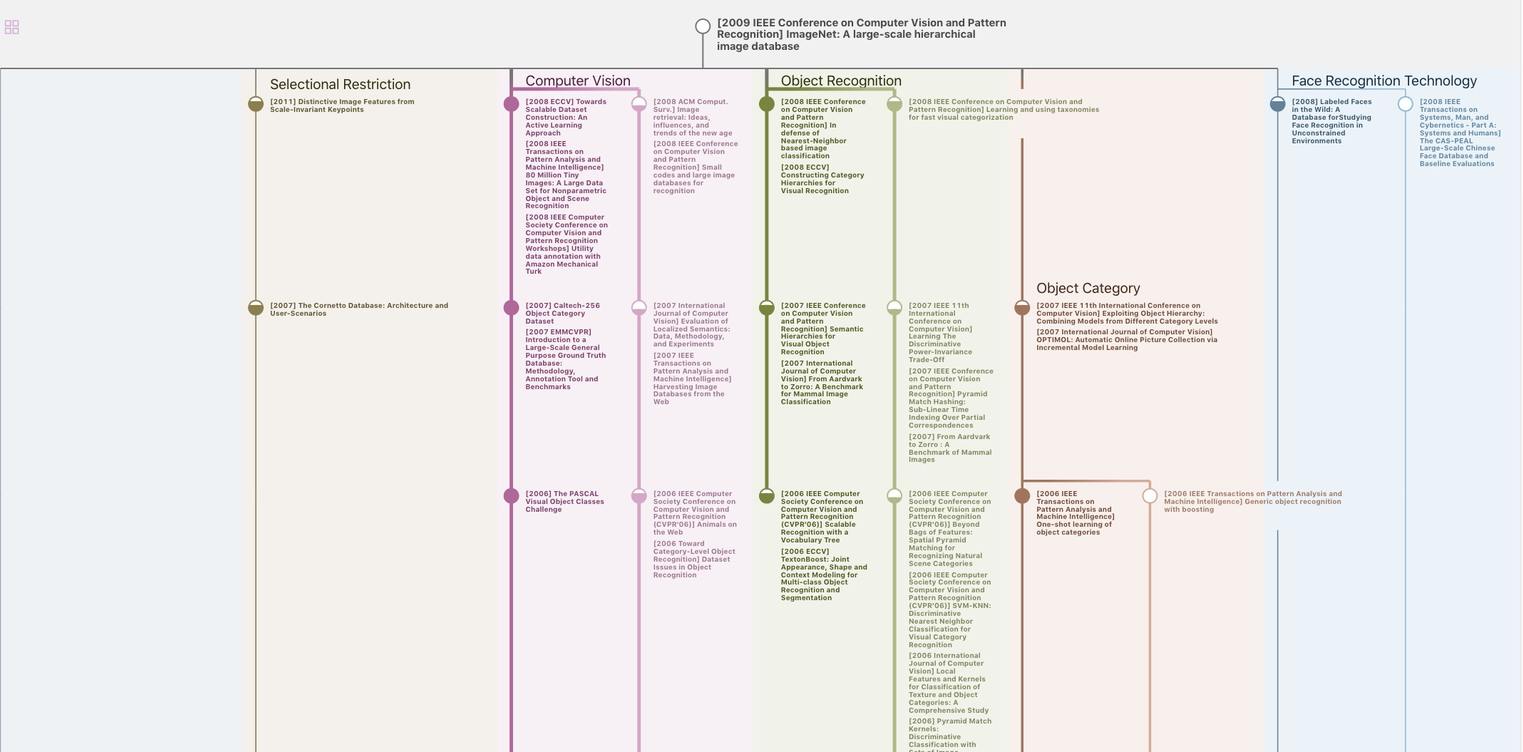
生成溯源树,研究论文发展脉络
Chat Paper
正在生成论文摘要