MVPNet: A multi-scale voxel-point adaptive fusion network for point cloud semantic segmentation in urban scenes
International Journal of Applied Earth Observation and Geoinformation(2023)
摘要
Point cloud semantic segmentation, which contributes to scene understanding at different scales, is crucial for three-dimensional reconstruction and digital twin cities. However, current semantic segmentation methods mostly extract multi-scale features by down-sampling operations, but the feature maps only have a single receptive field at the same scale, resulting in the misclassification of objects with spatial similarity. To effectively capture the geometric features and the semantic information of different receptive fields, a multi-scale voxelpoint adaptive fusion network (MVP-Net) is proposed for point cloud semantic segmentation in urban scenes. First, a multi-scale voxel fusion module with gating mechanism is designed to explore the semantic representation ability of different receptive fields. Then, a geometric self-attention module is constructed to deeply fuse fine-grained point features with coarse-grained voxel features. Finally, a pyramid decoder is introduced to aggregate context information at different scales for enhancing feature representation. The proposed MVP-Net was evaluated on three datasets, Toronto3D, WHU-MLS, and SensatUrban, and achieved superior performance in comparison to the state-of-the-art (SOTA) methods. For the public Toronto3D and SensatUrban datasets, our MVP-Net achieved a mIoU of 84.14% and 59.40%, and an overall accuracy of 98.12% and 93.30%, respectively.
更多查看译文
关键词
Point cloud,Multi-scale voxel,Semantic segmentation,Geometric self-attention,Pyramid decoder
AI 理解论文
溯源树
样例
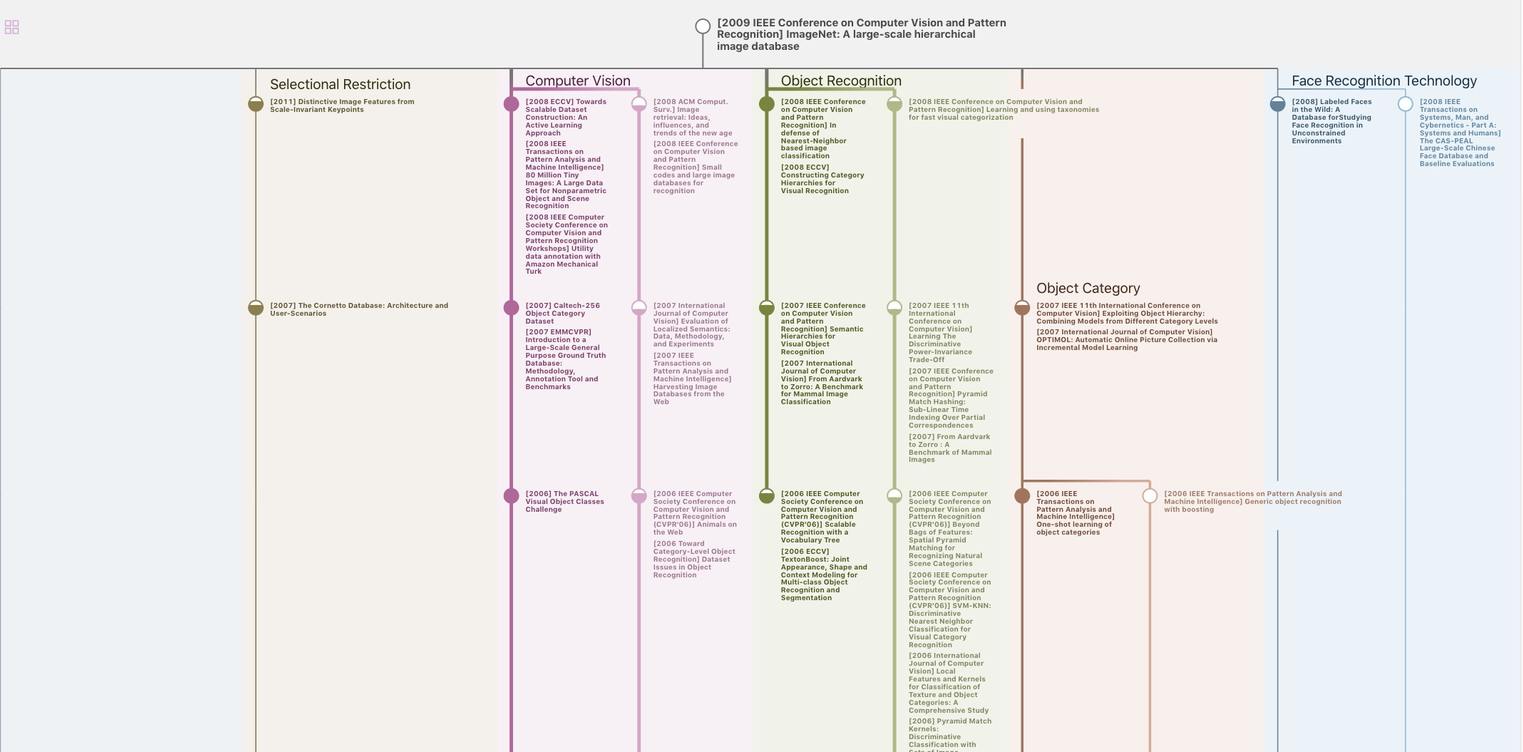
生成溯源树,研究论文发展脉络
Chat Paper
正在生成论文摘要