Machine Learning for Realised Volatility Forecasting
SSRN Electronic Journal(2020)
Abstract
This paper compares machine learning and HAR models for forecasting realised volatility of 23 NASDAQ stocks using 146 variables extracted from limit order book (LOBSTER) and stock-specific news (Dow Jones Newswires) from 27 July 2007 to 18 November 2016. We find simpler ML to outperform HARs on normal volatility days. With SHAP, an Explainable AI technique, we find simple mid prices at all limit order book levels and mean bid/ask prices drive RV forecasts for many stocks. An ML model with a larger number of units and complex idiosyncratic LOB variables are needed for forecasting volatility jumps.
MoreTranslated text
Key words
realised volatility forecasting,machine learning
AI Read Science
Must-Reading Tree
Example
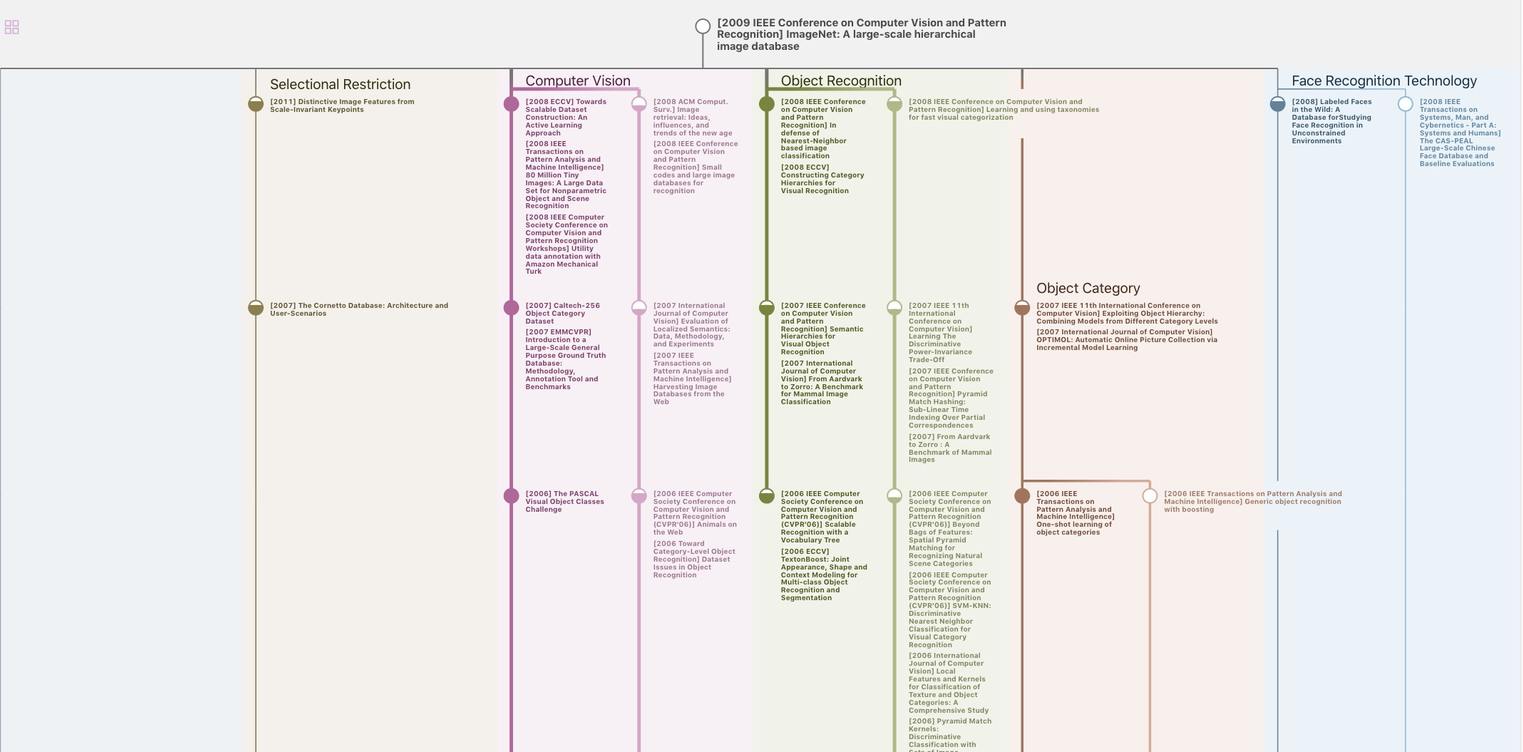
Generate MRT to find the research sequence of this paper
Chat Paper
Summary is being generated by the instructions you defined