Privacy-preserving malware detection in Android-based IoT devices through federated Markov chains
Future Generation Computer Systems(2023)
摘要
The continuous emergence of new and sophisticated malware specifically targeting Android-based Internet of Things devices is causing significant security hazards and is consequently fostering the need for effective detection models and strategies able to work with these hardware-constrained devices. In addition, since such models are often trained on confidential application data, many involved subjects are reluctant to share their data for this purpose. Accordingly, several Federated Learning -based solutions are emerging, which rely on the capabilities of Machine Learning models in malware detection/classification without sharing user data. However, Federated Learning methods are often adversely affected by non-independent and identically distributed data in terms of both the required training time and classification results. Therefore, a promising solution could be to overcome the Federated Learning-related issues by preserving the privacy of end-user data. In this direction, the capabilities of Markov chains and associative rules are extended within a federated environment to face malware classification tasks in the IoT scenario. The presented approach, evaluated on several malware families, has achieved an average accuracy of 99% in the presence of centralized and decentralized unbalanced training/testing data by overcoming the most common state-of-the-art approaches. Also, its runtime performance is comparable with centralized ones by considering several non-independent and identically distributed dataset partitions, splitting criteria, and clients, respectively.& COPY; 2023 Elsevier B.V. All rights reserved.
更多查看译文
关键词
Malware classification,Federated learning,Markov chains,Privacy
AI 理解论文
溯源树
样例
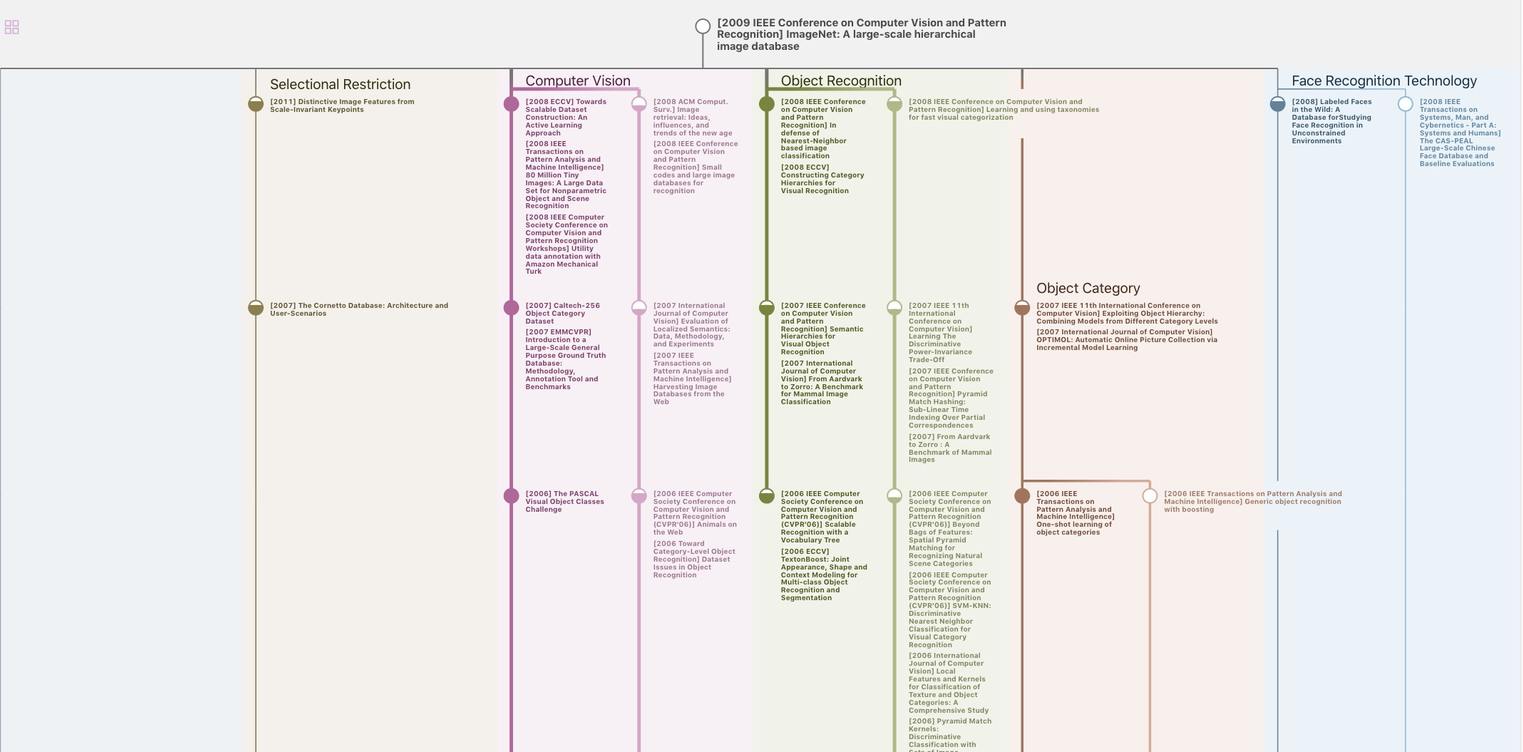
生成溯源树,研究论文发展脉络
Chat Paper
正在生成论文摘要