Speaker identification from emotional and noisy speech using learned voice segregation and speech VGG
Expert Systems with Applications(2023)
摘要
Speech signals are more susceptible to emotional influences and acoustic interference than other communications. Applications for real-time speech processing face difficulties when dealing with noisy, emotion-filled speech data. Finding a reliable method to separate the dominating signal from outside influences. An ideal system should be capable of precisely identifying necessary auditory events from a complex scene captured in an undesirable circumstance. In this work, we proposed and evaluated an end-to-end framework for voice recognition in adverse talking conditions using a pre-trained Deep Neural Network mask and voice VGG. This research suggests a unique method for speaker recognition under challenging circumstances, including emotion and interference. Using the Ryerson audio–visual dataset, the presented model outperformed recent literature on emotional speech data in English and Arabic, reporting an average speaker identification rate of 85.2%, 87.0%, and 86.6% using the Ryerson audio–visual dataset (RAVDESS), speech under simulated and actual stress (SUSAS) dataset and Emirati-accented Speech dataset (ESD) respectively.
更多查看译文
关键词
Deep Neural Network,Emotional talking conditions,Feature extraction,Noise reduction,Speaker identification,Speech segregation
AI 理解论文
溯源树
样例
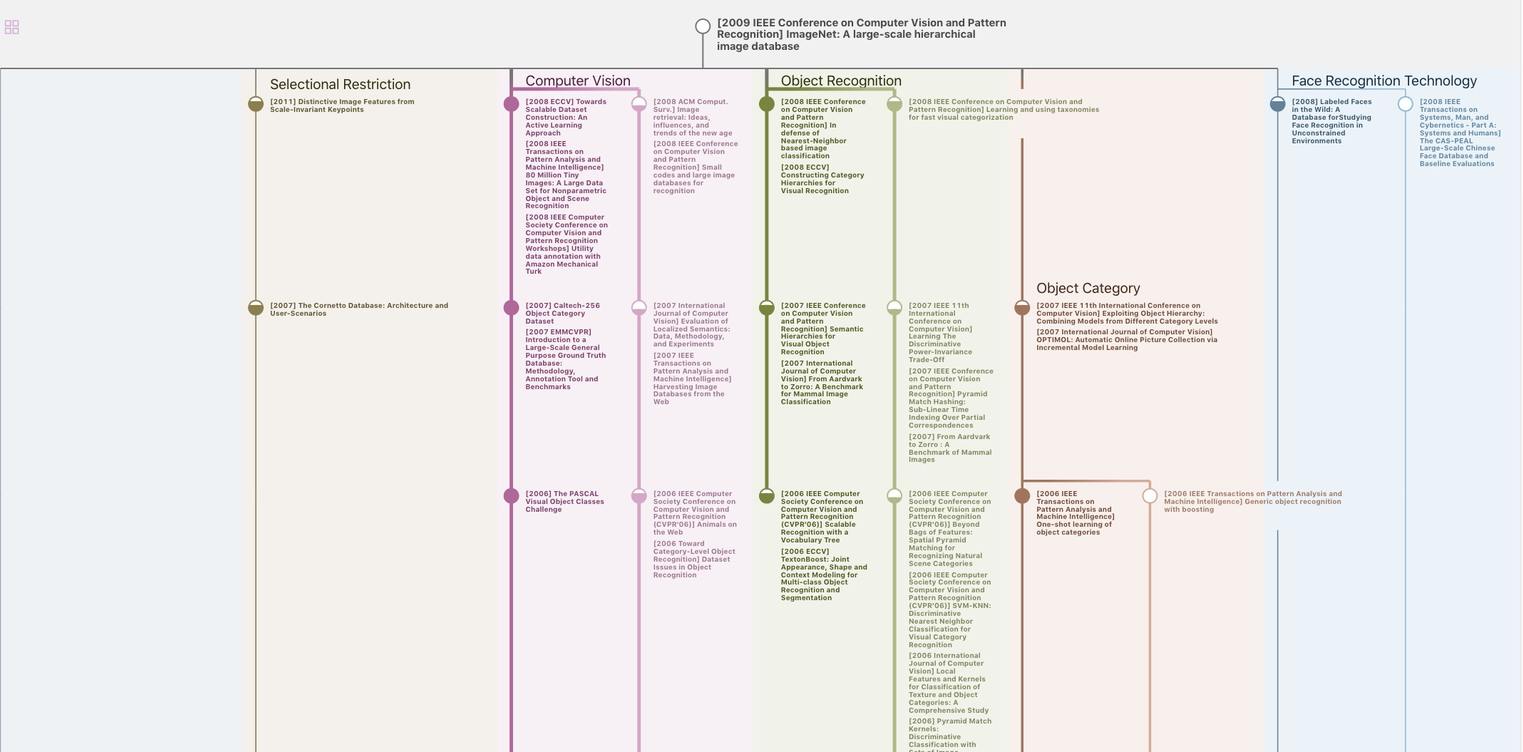
生成溯源树,研究论文发展脉络
Chat Paper
正在生成论文摘要