Elasticity-mechanics-informed generative adversarial networks for predicting the thermal strain of thermal barrier coatings penetrated by CaO–MgO–Al2O3–SiO2
European Journal of Mechanics - A/Solids(2023)
摘要
Air-plasma-sprayed thermal barrier coatings have complex and variable microstructures. As a result, they require repeated numerical or experimental analyses in each case, which increases the computation time and limits the optimization of the coating structure and processing parameters. In this work, a neural network-based framework for rapidly evaluating the thermal strain of thermal barrier coatings is proposed. The deformation compatibility condition and the generalized Hooke's law considering thermal expansion are applied to constrain the network. The training database is established using one high-resolution scanning electron microscopy structural image. The proposed model is validated using another image database with different microstructure characteristics and cooling temperature ranges. The model strains generated by the proposed model, the general pixel-to-pixel network, and finite-element models are compared. The results show that the constraint equations improve the generalization ability of the neural networks, and the proposed model exhibits higher prediction accuracy and confidence than general networks.
更多查看译文
关键词
Elasticity–mechanics-informed GAN,Image-based strain prediction,Thermal barrier coatings,Thermoelasticity
AI 理解论文
溯源树
样例
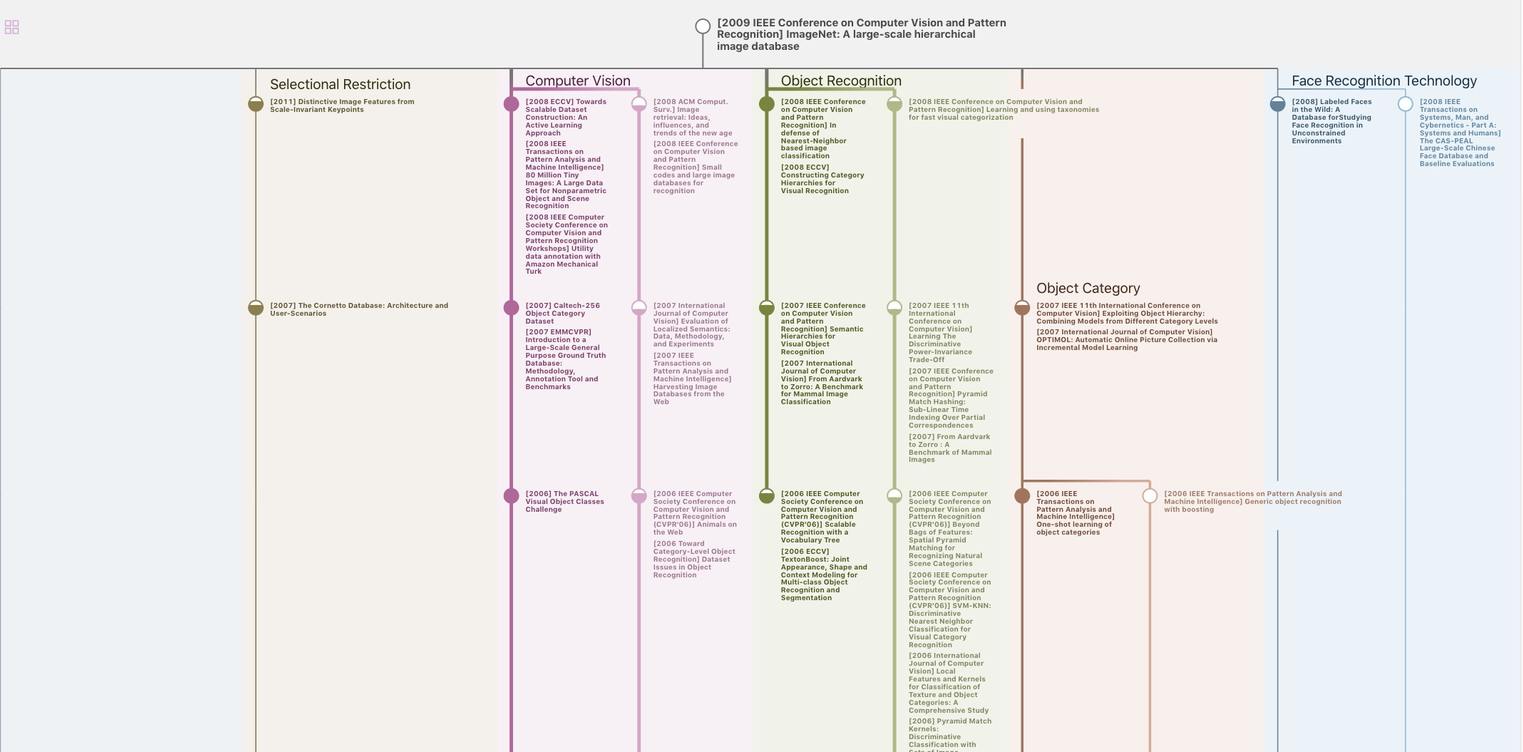
生成溯源树,研究论文发展脉络
Chat Paper
正在生成论文摘要