An enhanced data-driven constitutive model for predicting strain-rate and temperature dependent mechanical response of elastoplastic materials
European Journal of Mechanics - A/Solids(2023)
摘要
Data-driven and machine-learning based approaches provide a highly compatible and efficient fundamentals for the mechanical constitutive modeling of engineering materials. In this work, an enhanced data-driven constitutive model is developed to predict the stress–strain relationship of an elastoplastic material through the integration of a data-driven concept with fundamental plasticity theory. A novel strain reconfiguration strategy is proposed to improve the learning capability and predictability of the data-driven model, along with a two-step training method. A compatible numerical implementation algorithm is developed to incorporate the data-driven approach into a finite element calculation. This developed data-driven constitutive model is applied to learn and predict the mechanical response of Ti-6Al-4V titanium alloy under multiple loading conditions, including five different loading rates, four different temperatures, and thirteen different stress states. The excellent correlation with the experimental results demonstrates the high accuracy and generality of the presented approach, especially its capability for predicting unknown nonlinear stress–strain response. The presented theory reveals the great potential of employing such a data-driven approach in computational mechanics.
更多查看译文
关键词
dependent mechanical response,constitutive model,data-driven,strain-rate
AI 理解论文
溯源树
样例
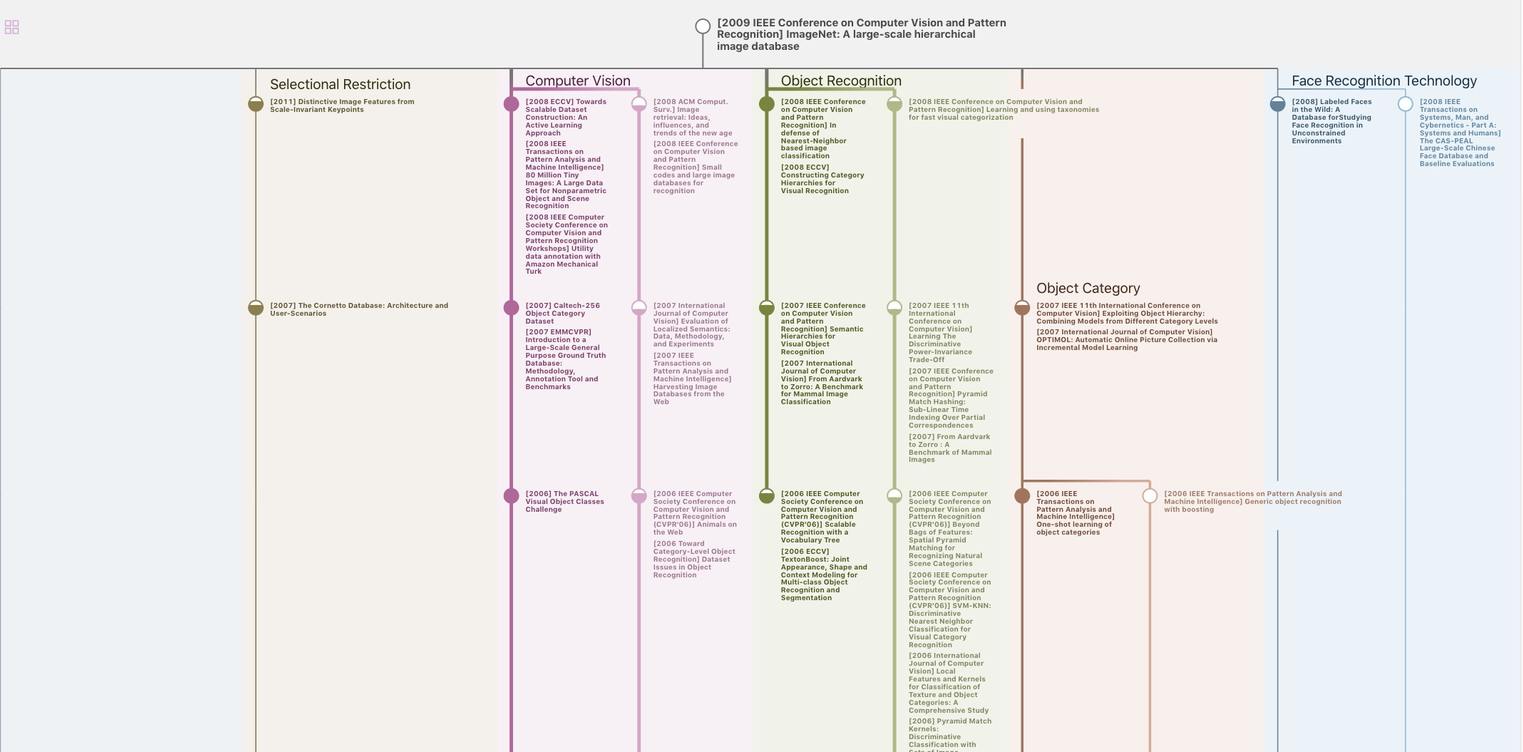
生成溯源树,研究论文发展脉络
Chat Paper
正在生成论文摘要