Machine learning-based detection of frost events in wheat plants from infrared thermography
European Journal of Agronomy(2023)
摘要
Frost is an extreme temperature event that significantly impacts crops, particularly in Mediterranean-type climates. Current frost damage assessment techniques are heavily dependent on traditional temperature logger data and manual inspection of the crops after a suspected frost event, an approach that can be erroneous, labour-intensive and can lead to delayed management decisions. This study investigates a new technique to automatically detect two crucial stages of frost in on-field plants, i.e., exposure to freezing temperatures with and without ice formation (crystallisation and supercooling), using machine learning (ML) models trained on infrared thermal (IRT) images. Our dataset consists of IRT images of on-field wheat plants collected during the winter growing season. We demonstrate that our approach based on classification accuracy curves, can detect ice nucleation and freezing point temperatures with four ML models, extreme gradient boosting (XGBoost), random forest (RF), convolutional neural networks (CNN) and ResNet-50. We find that RF detects frost events, i.e., crystallisation for frost and supercooling for non-frost night from the accuracy curves with fastest classification time (approx. 17 ms per image). Our study provides important insights into a primary building block for the future development of automatic and real-time on-field plant frost monitoring systems.
更多查看译文
关键词
infrared thermography,frost events,wheat plants,learning-based
AI 理解论文
溯源树
样例
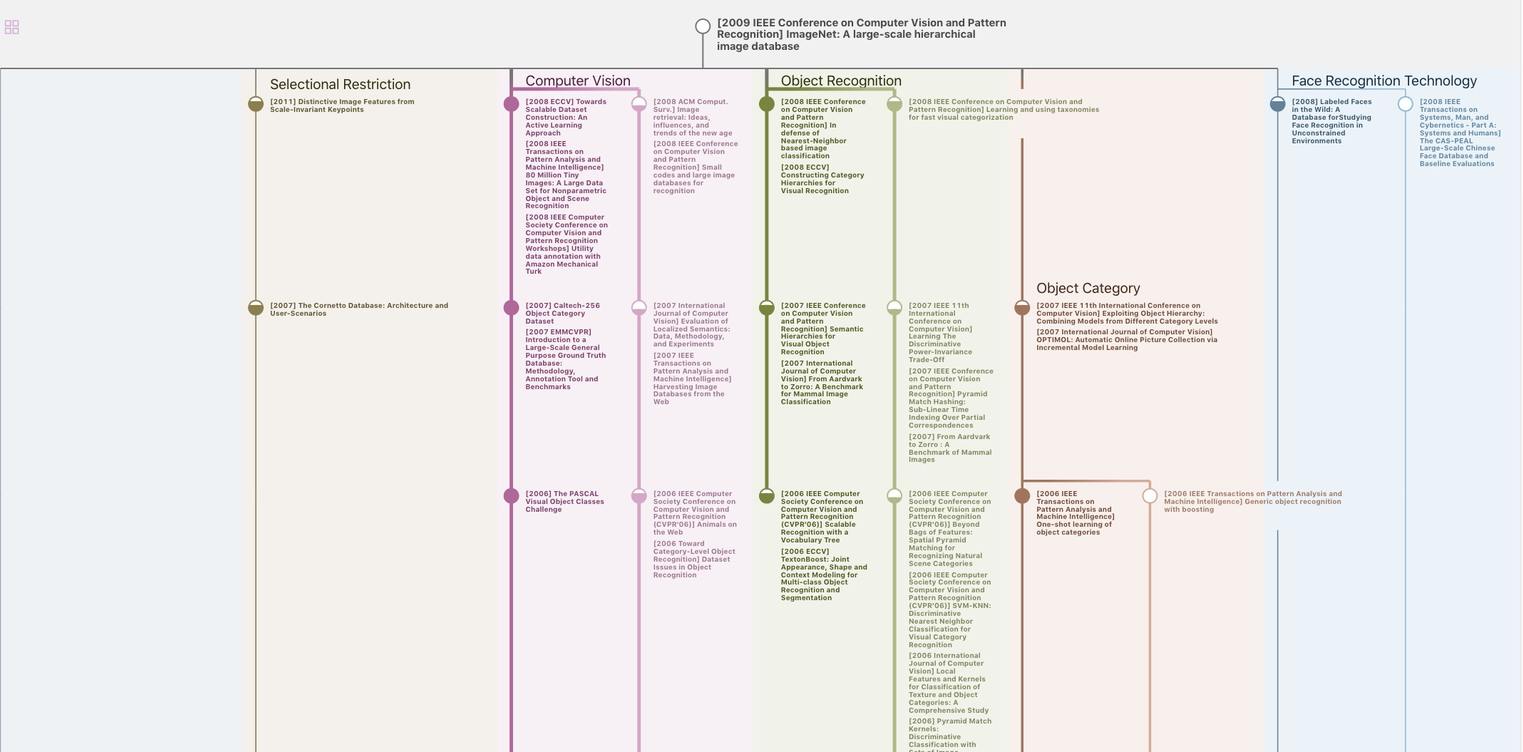
生成溯源树,研究论文发展脉络
Chat Paper
正在生成论文摘要