An autonomous and heuristic approach for extracting bridge frequencies from passing vehicles
Engineering Structures(2023)
摘要
The Federal Highway Administration (FHWA) recognizes a need for cost-effective and practical structural health monitoring (SHM) techniques to manage the bridge network. System identification (SI) techniques are the first step in developing SHM frameworks to extract bridge dynamic properties (natural frequencies, damping ratios, and mode shapes). One promising indirect SI technique uses accelerometer-instrumented vehicles to measure the dynamic properties of bridges, such that a few instrumented vehicles could economically monitor a large network of bridges. This study proposes an autonomous framework called autonomous peak picking variational mode decomposition (APPVMD), which leverages an ensemble of signal processing techniques and heuristic models to autonomously extract bridge frequencies from instrumented vehicles. The proposed framework does not require prior information or model-informed training. The framework is tested on four vehicles and 39 unique bridge classes using finite element (FE) models to assess the feasibility of deploying it into practice. The results of this study indicate that the algorithm can make successful bridge frequency extractions in more than 69.2% of the bridge cases using any vehicle and a maximum value of 97.4% using an SUV, while considering the effects of road surface roughness. The influence of bridge stiffness, mass, and span length on the algorithm’s success rate is discussed. It is concluded that flexural rigidity and span length play a significant role in the accuracy of APPVMD. The combined effects of bridge inertia and damping cause heavier bridges to have a higher amplitude signal for a longer duration, increasing the algorithm’s successful detection rate of bridge frequency. Next, the authors examine the effect of reducing the tire stiffness in the truck model on the success of the APPVMD algorithm. The customized truck outperformed the original truck and the SUV by successfully extracting the bridge frequencies of 97.4% of the bridge cases and by having more bridge frequencies predicted in a higher confidence region than the SUV. In addition, the customized truck model is used to verify the APPVMD’s robustness to large levels of sensor noise. Even at significant levels of white noise (1% SNR), as the success rate for bridge frequency extraction using the customized truck only drops to 84.6%. Finally, the influence of vehicle speed on the APPVMD algorithm’s effectiveness is studied. Generally, slower speeds resulted in more successful predictions of bridge frequency, but minor improvements in the accuracy of the frequency predictions were observed at slightly increased velocities, 13.4 m/s (30 mph) and 17.9 m/s (40 mph), but a reduction in accuracy was observed at higher velocities, 22.3 m/s (50 mph).
更多查看译文
关键词
bridge frequencies,vehicles,heuristic approach
AI 理解论文
溯源树
样例
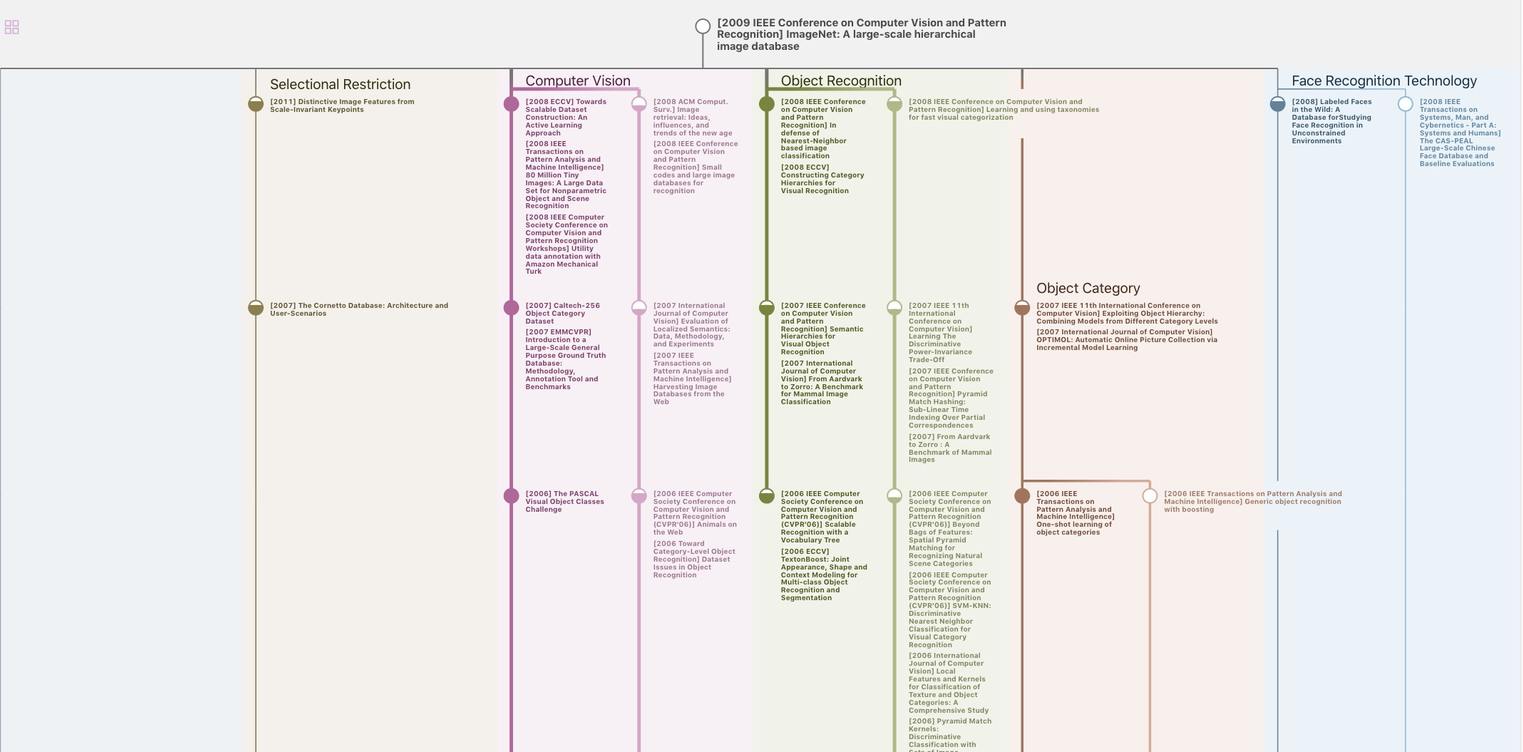
生成溯源树,研究论文发展脉络
Chat Paper
正在生成论文摘要