Meta-learning-based multi-objective PSO model for dynamic scheduling optimization
Energy Reports(2023)
摘要
The by-product gas is an important secondary energy in the iron and steel industry. It is important to make the by-product gas's utilization efficient and reasonable,which is the key to improve the economic efficiency and the level of energy conservation and emission reduction. Aiming at the problems of complex dynamic changes, difficult to accurately model and difficult to predict real-time traffic in gas energy system, this paper proposes an optimal scheduling method based on meta-learning multi-objective particle swarm optimization algorithm. The gas energy optimization scheduling problem is modeled as a multi-objective dynamic scheduling optimization problem. The difficulty of this problem is to comprehensively consider multiple indicators, continuously adapt to the change of the objective function over time, and track the changing Pareto optimal solution set. One of the promising solutions is to build prediction models with better performance under dynamic changes. The model we propose makes full use of the optimization results and the experience information of the optimization process in the historical optimization task. We propose a dynamic parameter initialization method based on meta-learning by starting multiple historical optimization tasks at the same time. Thus,the comprehensive parameters of different tasks are obtained, and a general and best base parameter is learned. Experiments show that the proposed model has better convergence and accuracy than the conventional algorithm. (c) 2023 Published by Elsevier Ltd. This is an open access article under the CCBY-NC-ND license (http://creativecommons.org/licenses/by-nc-nd/4.0/).
更多查看译文
关键词
Energy scheduling,Meta-learning,Multi-objective dynamic optimization,Pre-knowledge learning
AI 理解论文
溯源树
样例
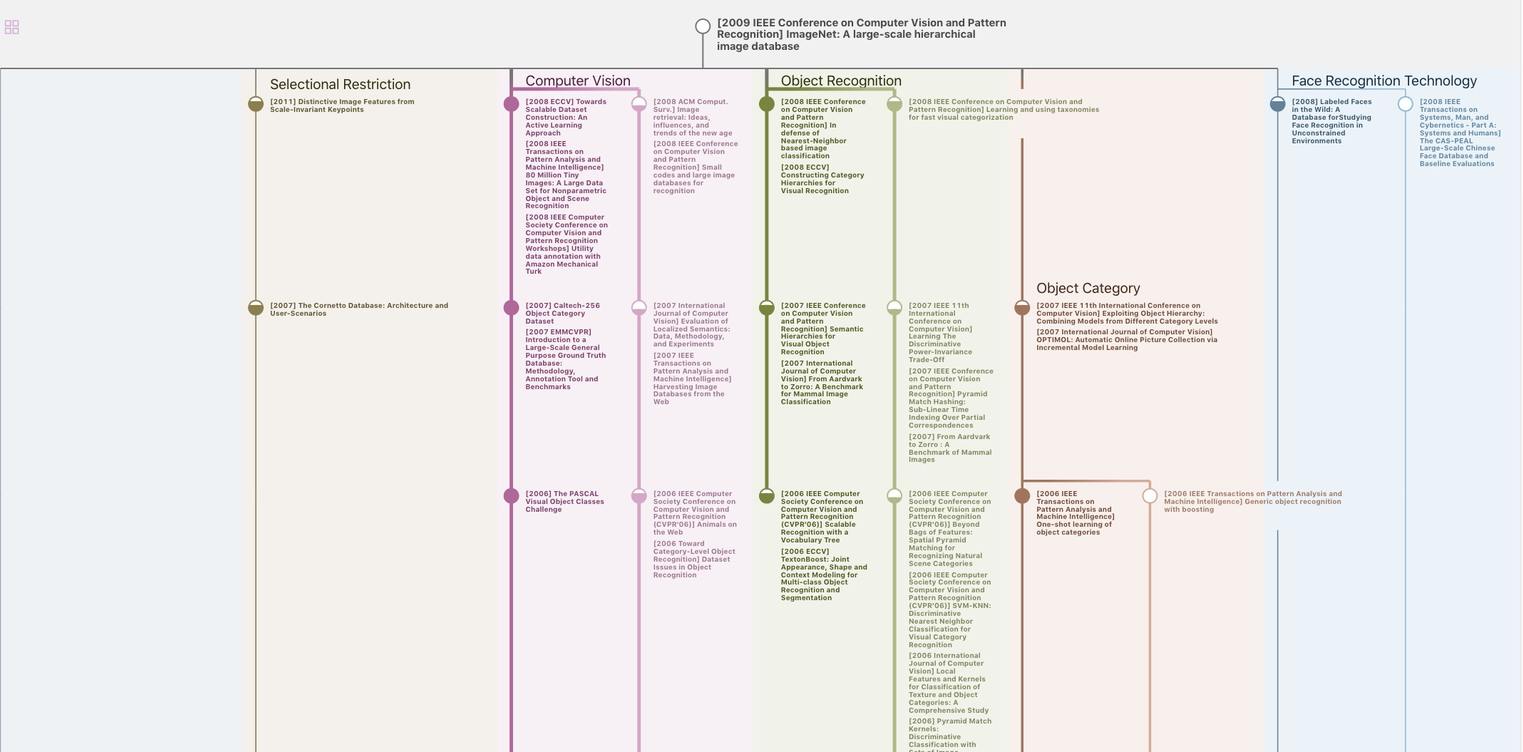
生成溯源树,研究论文发展脉络
Chat Paper
正在生成论文摘要