SEA-net: Sequence attention network for seismic event detection and phase arrival picking
Engineering Applications of Artificial Intelligence(2023)
摘要
Automatic seismic phase detection and phase arrival time picking are always fundamental tasks in seismology. With the emergence of machine learning, and deep learning in particular, a number of neural network architectures are being used to explore the correlations between seismic waveforms and hidden information of interest. In this paper, we present a sequential attention (SEA) module that can learn both local and long-distance corrections to effectively extract sequential features. This module is lightweight and plug-in-play. Based on the SEA module, a fully convolutional neural network with sequential attention mechanism was constructed to detect seismic events and determine the arrival time of phases. Further experiments on STEAD dataset, in comparison with existing methods, show that our proposed model outperforms other algorithms with a higher F1-score (100% for seismic detection, 98.8% for P arrival picking, and 89.0% for S arrival picking) and fewer residual errors. Comparatively, our model has a quarter of the model parameters and computational complexity as well as a 36.7% reduction in processing time compared to the current state-of-the-art method. This demonstrates that the proposed architecture is effective for seismic sequential learning tasks and, as a result, can serve as an improved alternative backbone.
更多查看译文
关键词
Seismic detection, Sequence attention, Phase picking, Convolution network, Deep learning
AI 理解论文
溯源树
样例
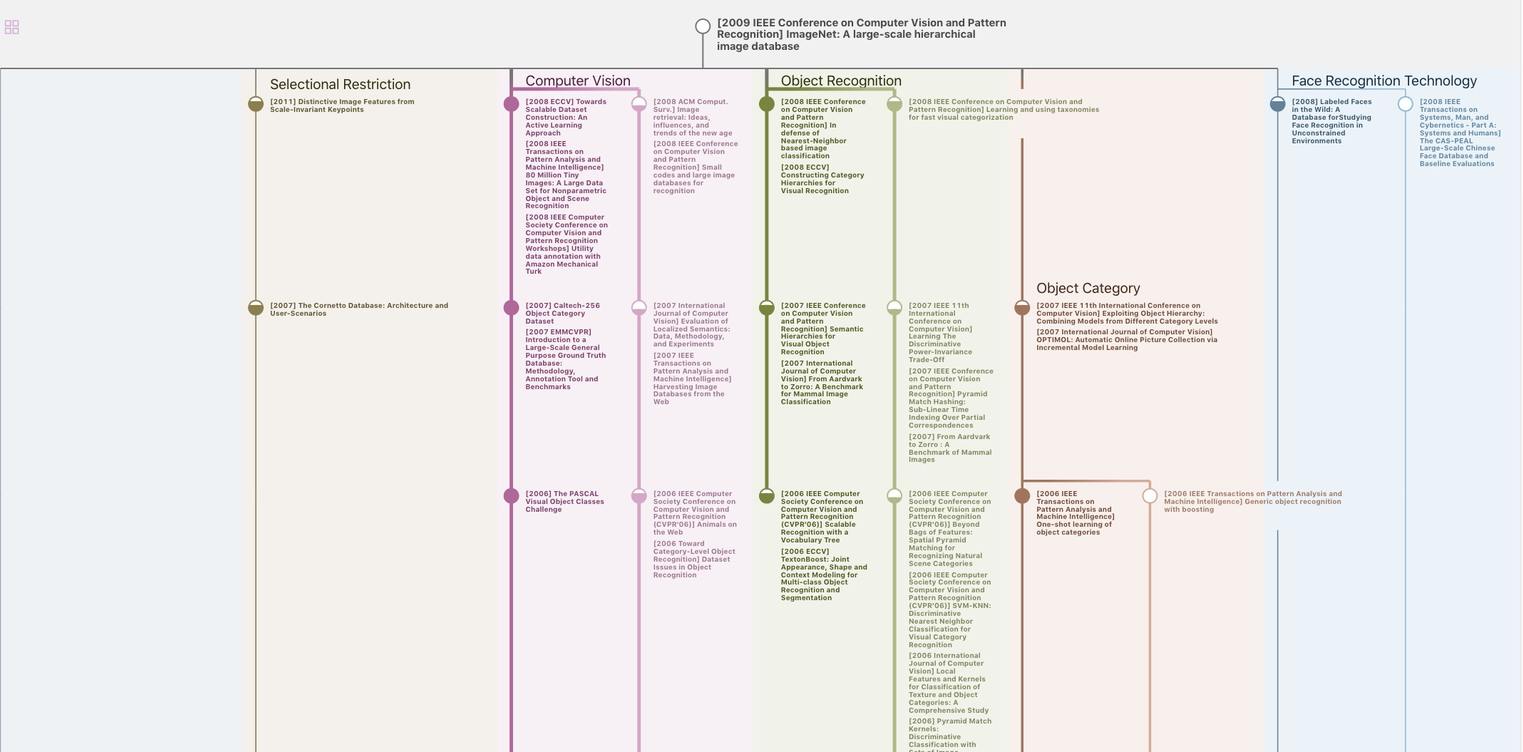
生成溯源树,研究论文发展脉络
Chat Paper
正在生成论文摘要