DGFaceNet: Lightweight and efficient face recognition
Engineering Applications of Artificial Intelligence(2023)
Abstract
Face recognition has achieved great success due to the development of deep convolutional neural networks (DCNNs). However, complex DCNNs bring a large number of parameters as well as computational effort, which poses a significant challenge to resource-constrained embedded devices. Meanwhile, the commonly popular loss functions and lightweight networks are not so effective for face recognition. In this paper, we first investigate the impact of the number of similar features generated by inexpensive operations on model performance. It is shown that DCNNs can tolerate more similar features generated by cheap operations in the early stage of the network. We construct Dynamic Ghost Bottleneck based on this idea, and DGFaceNet is composed of stacking Dynamic Ghost Bottleneck. In addition, we propose a new class-margin-linear softmax loss function (CML-softmax) for lightweight networks. CML-softmax designs a quadratic function to replace the cosine function as the target logit, which allows better performance and convergence in low-dimensional output for face recognition. Meanwhile, CML-softmax introduces two margin functions to alleviate class imbalance and softmax early saturation problems, respectively. Our method demonstrates competitive results in many validation datasets and large-scale popular benchmark tests. Speed tests on embedded devices show that the actual inference time of DGFaceNet is 11.08 times, 8.57 times, 2.75 times, and 2.82 times faster than ResNet-50, EfficientNet, MobileNetV2, and MobileFaceNet, respectively. DGFaceNet can significantly improve the running efficiency of the model in resource-constrained embedded devices while ensuring the model's performance.
MoreTranslated text
Key words
Face recognition,Lightweight networks,Loss function,Embedded devices
AI Read Science
Must-Reading Tree
Example
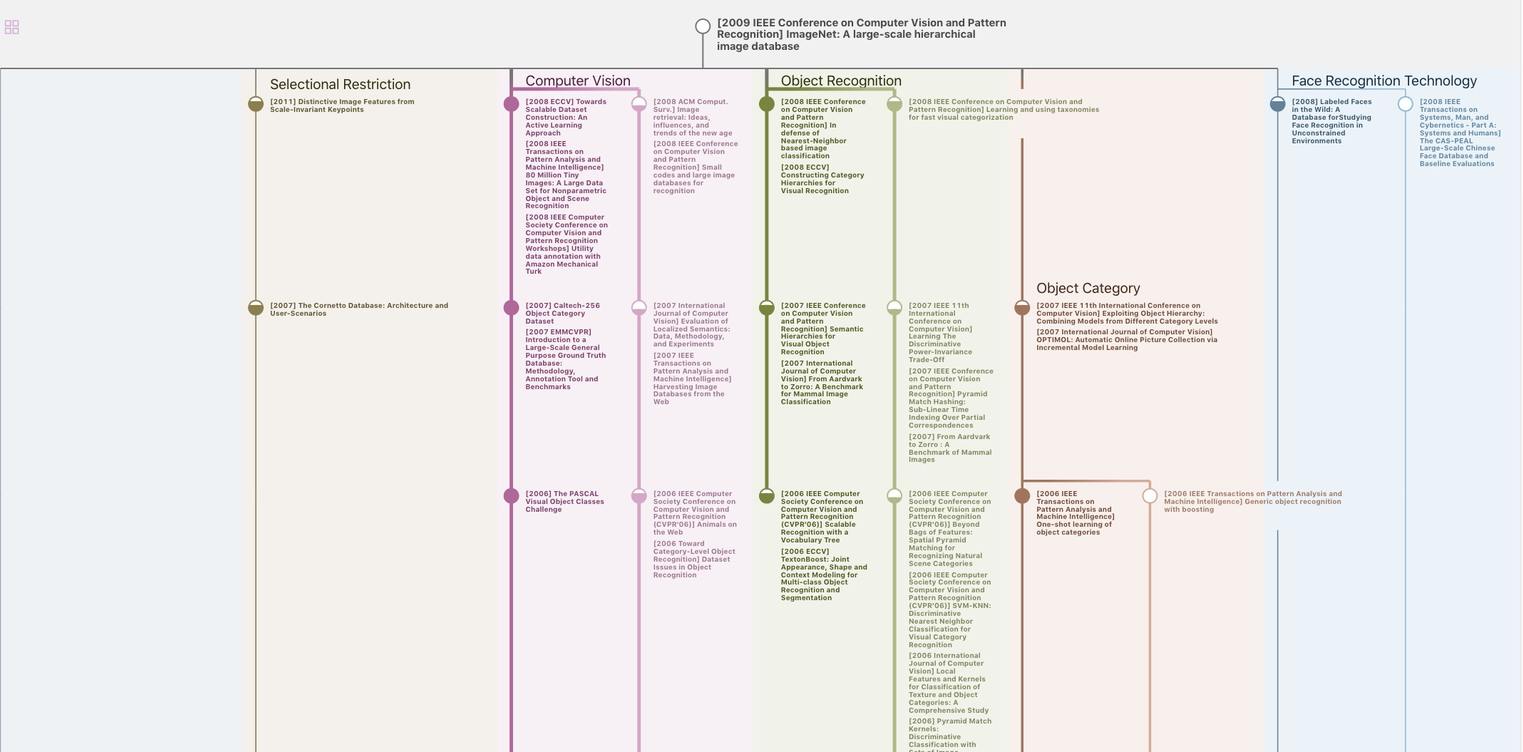
Generate MRT to find the research sequence of this paper
Chat Paper
Summary is being generated by the instructions you defined