Characterising surface roughness of Ti-6Al-4V alloy machined using coated and uncoated carbide tools with variable nose radius by machine learning
Engineering Applications of Artificial Intelligence(2023)
摘要
A rich literature explores how cutting parameters influence surface roughness in machining different materials using two- or three-dimensional visualisations. Research on Ti-6Al-4V alloy, an important but difficult-to-machine material, is relatively nascent. This research conducts real-life turning operations for Ti-6Al-4V alloy using coated and uncoated carbide inserts with different nose radii and subsequently applies ML techniques to simulate and characterise surface roughness (Ra) for varying cutting parameters — cutting speed (v), feed rate (f), depth of cut (d). Additionally, it presents an innovative visualisation of all three machining parameters’ influences on the surface roughness for Ti-6Al-4V alloy. The results identify Support Vector Machine (SVM) with RBF kernel as a highly potential ML approach to characterise the impact compared to SVM with linear kernel, Random Forest, Deep Learning, and ensemble approaches. The results also demonstrate, for a low feed rate and cutting speed, the surface roughness is better irrespective of the depth of cut and cutting tool materials. For coated carbide with a 0.40 mm nose radius and feed rate of 0.10 mm/rev, for example, Ra values stay within the range of 0.68 to 1.16 mμ for all experimented cutting speeds ranging from 50 m/min to 250 m/min. A similar outcome occurs for other cutting tools. The surface roughness tends to be higher for a higher feed rate. However, the Ra values can decrease even for a high feed rate if the cutting speed exceeds 200 m/min. Overall, the visualisation indicates a nonlinear relationship between machining parameters and surface roughness.
更多查看译文
关键词
uncoated carbide tools,surface roughness,alloy,variable nose radius
AI 理解论文
溯源树
样例
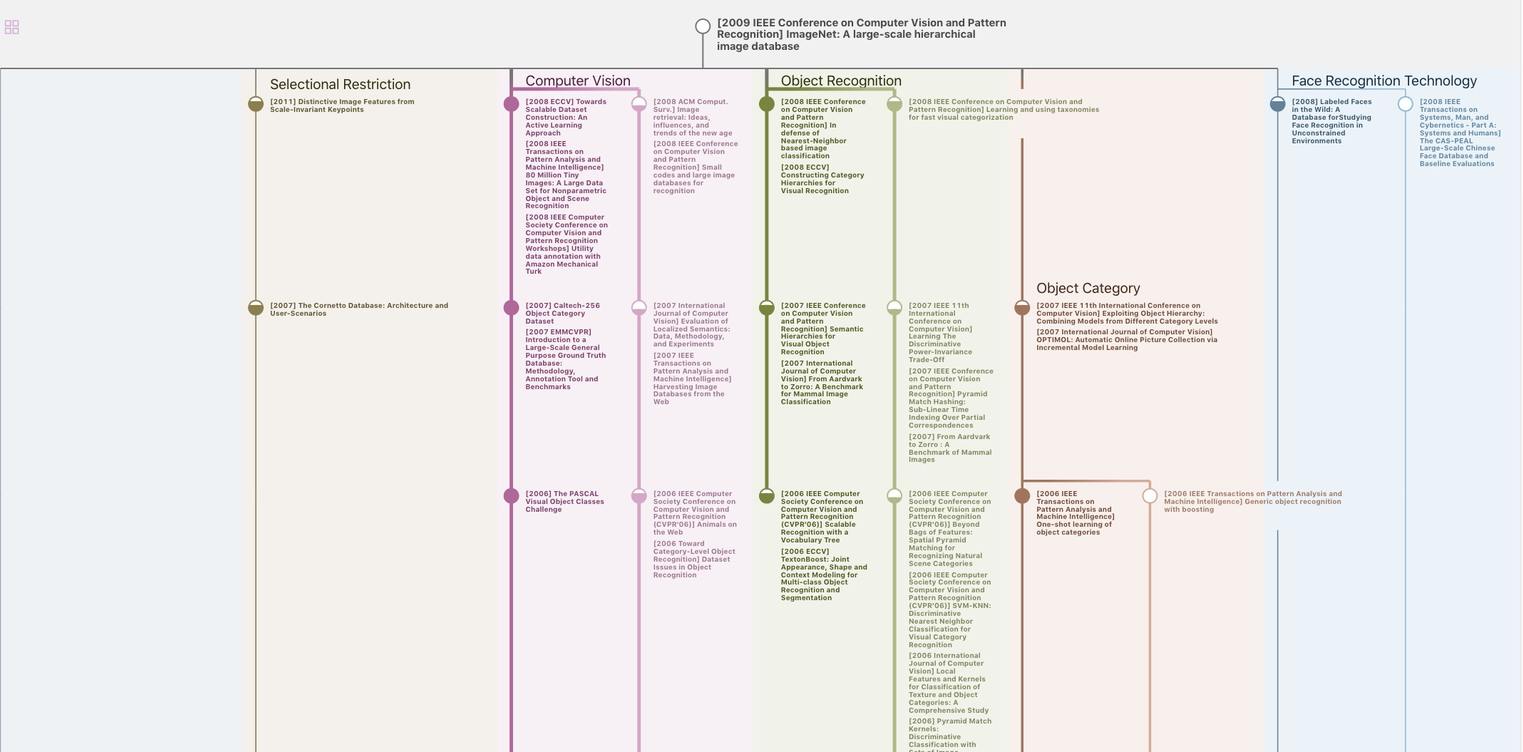
生成溯源树,研究论文发展脉络
Chat Paper
正在生成论文摘要