Comparative data-driven enhanced geothermal systems forecasting models: A case study of Qiabuqia field in China
Energy(2023)
摘要
Geothermal energy is gaining global attractiveness owing to its abundance and sustainable nature. An in-depth understanding of potential geothermal production provides the energy industry a possibility to diversify the supply portfolio. With the development of artificial intelligence, machine learning offers an efficient alternative to the conventional numerical simulation method in forecasting energy harvesting. However, a comprehensive comparison and an effective algorithm selection are absent from the machine learning applications in forecasting geothermal energy recovery. In this study, four machine learning algorithms based data-driven models are created to determine the optimal choice in predicting geothermal production, including K-Nearest Neighbors (KNN), Support Vector Machine (SVM), Extreme Gradient Boosting (XGBoost) and Artificial Neural Network (ANN). To investigate their application range, two different sizes of data groups are involved to train and test these models, and their performance is comprehensively compared. As the results show, the highest coefficient of determination R2 of 0.998 is demonstrated in the ANN models showing its promising predictive ability. Besides, the ANN is the most stable with the lowest performance variances between a training set and a validation set. In addition, the ANN is the most adaptable due to its minimal performance differences between different sizes of data groups. By jointly considering the prediction accuracy, stability and adaptability, the ANN is the best choice to substitute numerical simulation for predicting geothermal development. Importantly, the successful imple-mentation of the proposed data-driven model requires 2700 times less computational time compared to nu-merical simulation, demonstrating a considerable improvement in the prediction efficiency. The results provide a beneficial reference for operators in conducting machine learning to simulate the development of the geothermal system studied, and can be effectively applied in other energy systems.
更多查看译文
关键词
Geothermal energy,Machine learning,K -nearest neighbors,Support vector machine,Extreme gradient boosting,Artificial neural network
AI 理解论文
溯源树
样例
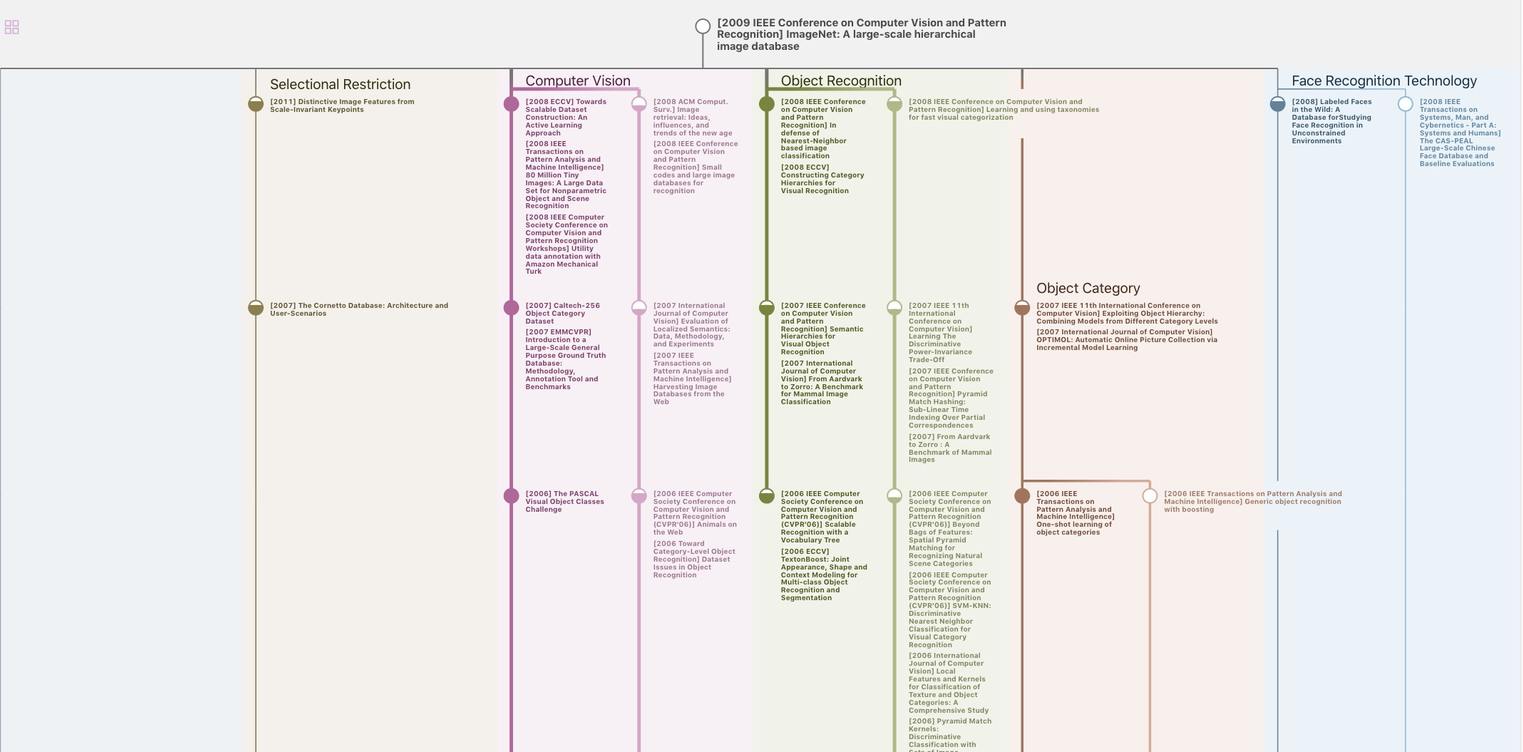
生成溯源树,研究论文发展脉络
Chat Paper
正在生成论文摘要