Characteristic Time Scale As Optimal Input in Machine Learning Algorithms: Homogeneous Autoignition
ENERGY AND AI(2023)
Key words
Machine Learning,Feature selection,Autoignition
AI Read Science
Must-Reading Tree
Example
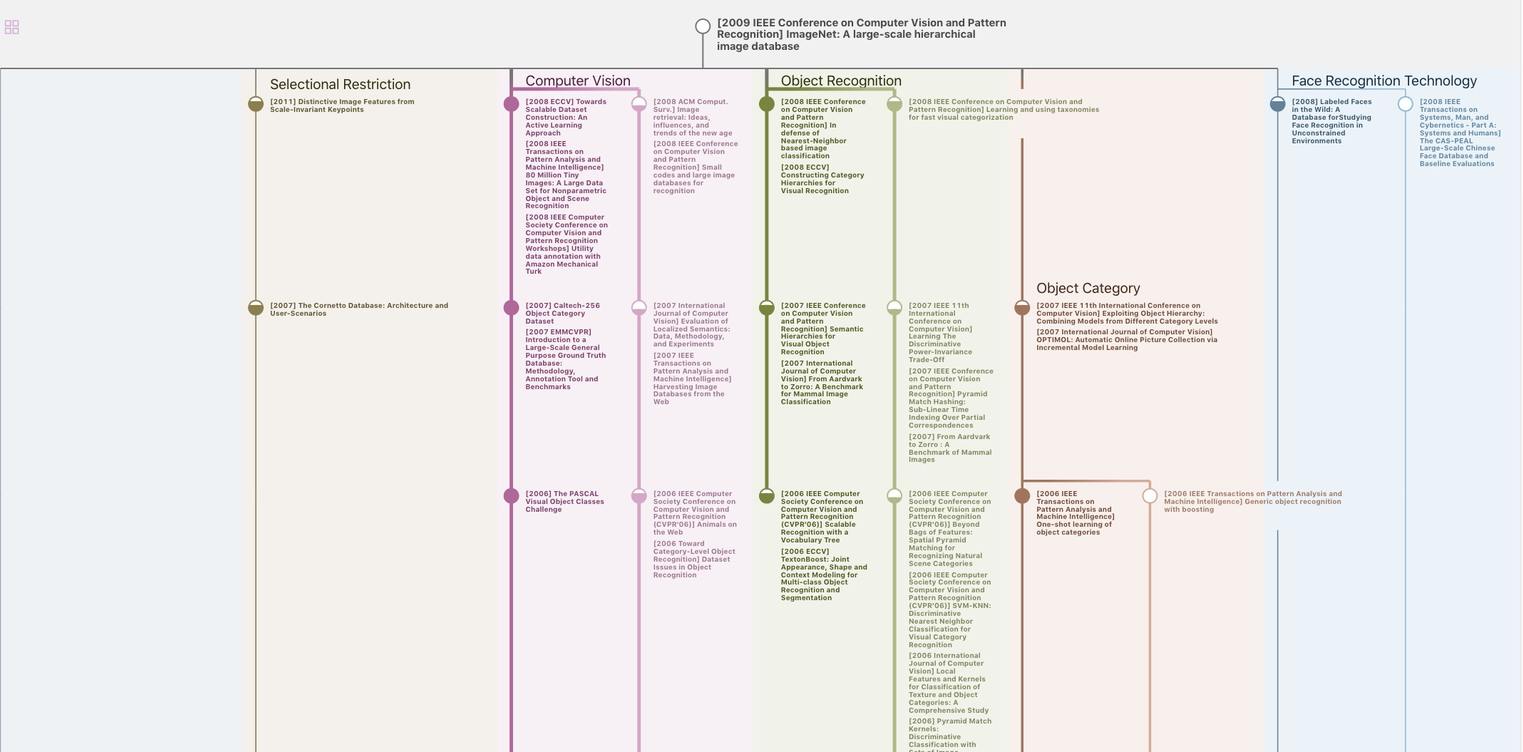
Generate MRT to find the research sequence of this paper
Chat Paper
Summary is being generated by the instructions you defined