Comparative evaluation of LSTM, CNN, and ConvLSTM for hourly short-term streamflow forecasting using deep learning approaches
Ecological Informatics(2023)
摘要
This study investigates the effectiveness of three deep learning methods, Long Short-Term Memory (LSTM), Convolutional Neural Network (CNN), and Convolutional Long Short-Term Memory (ConvLSTM), in short-term streamflow forecasting in the Kelantan and Muda River basins in Malaysia. The ability to forecast streamflow in the short-term is crucial for water resource management to prevent disasters such as floods. Hourly streamflow and rainfall data from the two river basins were used to evaluate the accuracy of the methods at one-hour, threehour, and six-hour prediction intervals. The results showed that all three deep learning methods performed with high accuracy in predicting streamflow. The study also found that LSTM performed better in small basin with well spatial distributed rainfall stations, while CNN and ConvLSTM were more effective in moderate to high streamflow, and large river basin. Moreover, early prediction intervals had better accuracy compared to later ones. The findings of this study demonstrate the potential of using deep learning methods for short-term streamflow forecasting in water resource management. The study highlights the importance of accurate shortterm streamflow forecasting and emphasizes the potential of using deep learning algorithms to achieve this goal.
更多查看译文
关键词
Streamflow,Forecasting,Deep learning methods,CNN,ConvLSTM
AI 理解论文
溯源树
样例
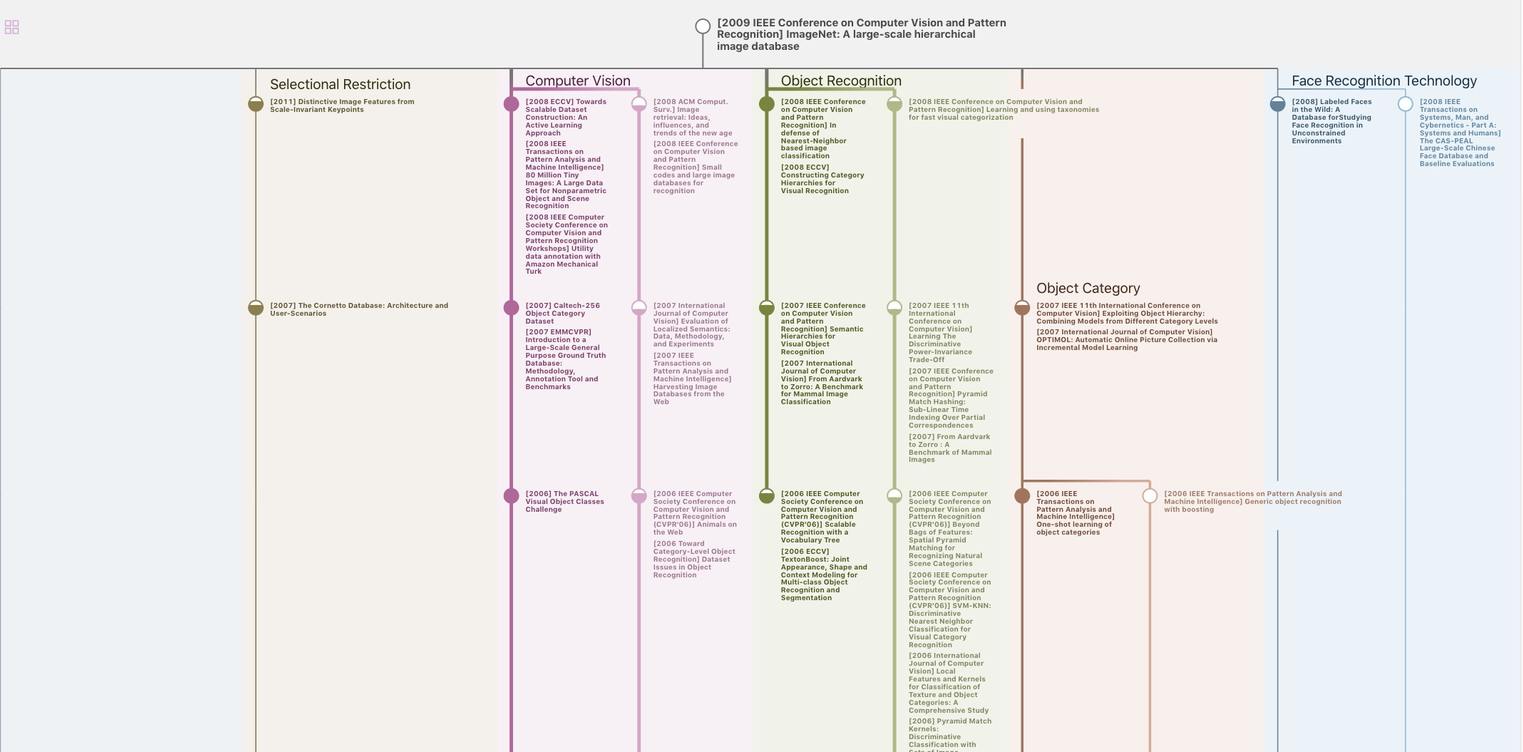
生成溯源树,研究论文发展脉络
Chat Paper
正在生成论文摘要