Interpretable data-driven constitutive modelling of soils with sparse data
Computers and Geotechnics(2023)
摘要
There is significant potential for machine learning (ML) to achieve step-change advances in the state-of-the-art for constitutive modelling of geomaterials. However, a common challenge and criticism of ML for geotechnical modelling is a lack of interpretability and onerous dependency on big data. This study proposes an interpretable data-driven constitutive modelling approach for application in the context of sparse geotechnical data. Improved interpretability is achieved by incorporating (a) prior known ‘tried-and-tested’ theoretical knowledge within the ML models and (b) uncertainty within the predictions. Three different theoretical descriptions of soil behaviour including incremental nonlinearity, hyperelasticity and elastoplasticity are considered and incorporated into the data-driven framework. To alleviate dependencies on big data, a ‘multi-fidelity’ modelling framework is adopted to maximise the impact of small ‘high-fidelity’ geotechnical datasets. The ability of pure and physics-constrained data-driven models to predict the response of both synthetic and real elemental data is subsequently compared. The results indicate that data-driven modelling with physical constraints shows more robust performance particularly for extrapolation outside the original calibration space of a small geotechnical dataset. The proposed data-driven modelling is generic and demonstrates exciting potential for modelling complex behaviours of various materials.
更多查看译文
关键词
Neural Networks,Constitutive relations,Uncertainty,Clays,Sands
AI 理解论文
溯源树
样例
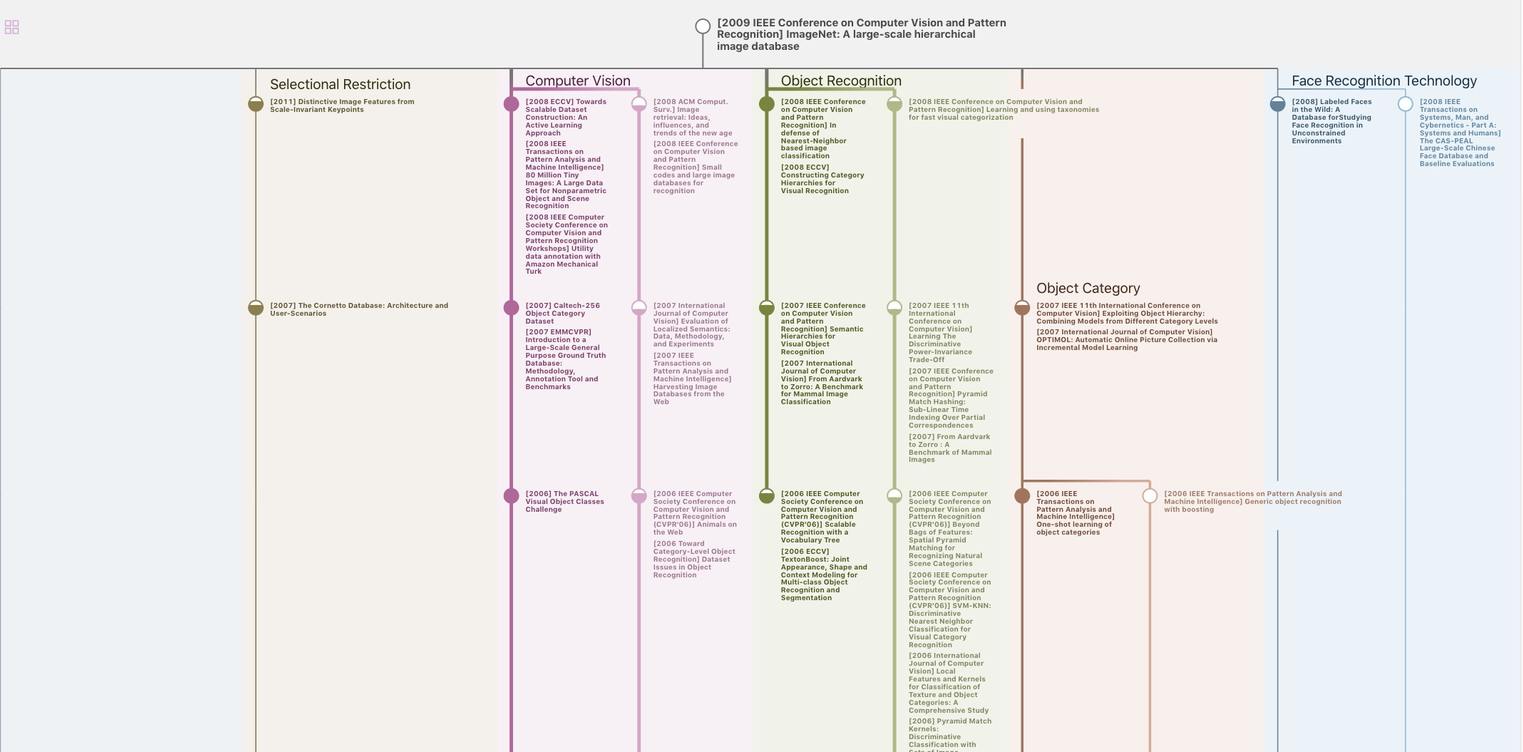
生成溯源树,研究论文发展脉络
Chat Paper
正在生成论文摘要