An overview on Meta-learning approaches for Few-shot Weakly-supervised Segmentation
Computers & Graphics(2023)
摘要
Semantic segmentation is a difficult task in computer vision that have applications in many scenarios, often as a preprocessing step for a tool. Current solutions are based on Deep Neural Networks, which often require a large amount of data for learning a task. Aiming to alleviate the strenuous data-collecting/annotating labor, research fields have emerged in recent years. One of them is Meta-Learning, which tries to improve the generability of models to learn in a restricted amount of data. In this work, we extend a previous paper conducting a more extensive overview of the still under-explored problem of Few-Shot Weakly-supervised Semantic Segmentation. We refined the previous taxonomy and included the review of additional methods, including Few-Shot Segmentation methods that could be adapted to the weak supervision. The goal is to provide a simple organization of literature and highlight aspects observed in the current moment, and be a starting point to foment research on this problem with applications in areas like medical imaging, remote sensing, video segmentation, and others.
更多查看译文
关键词
segmentation,meta-learning,few-shot,weakly-supervised
AI 理解论文
溯源树
样例
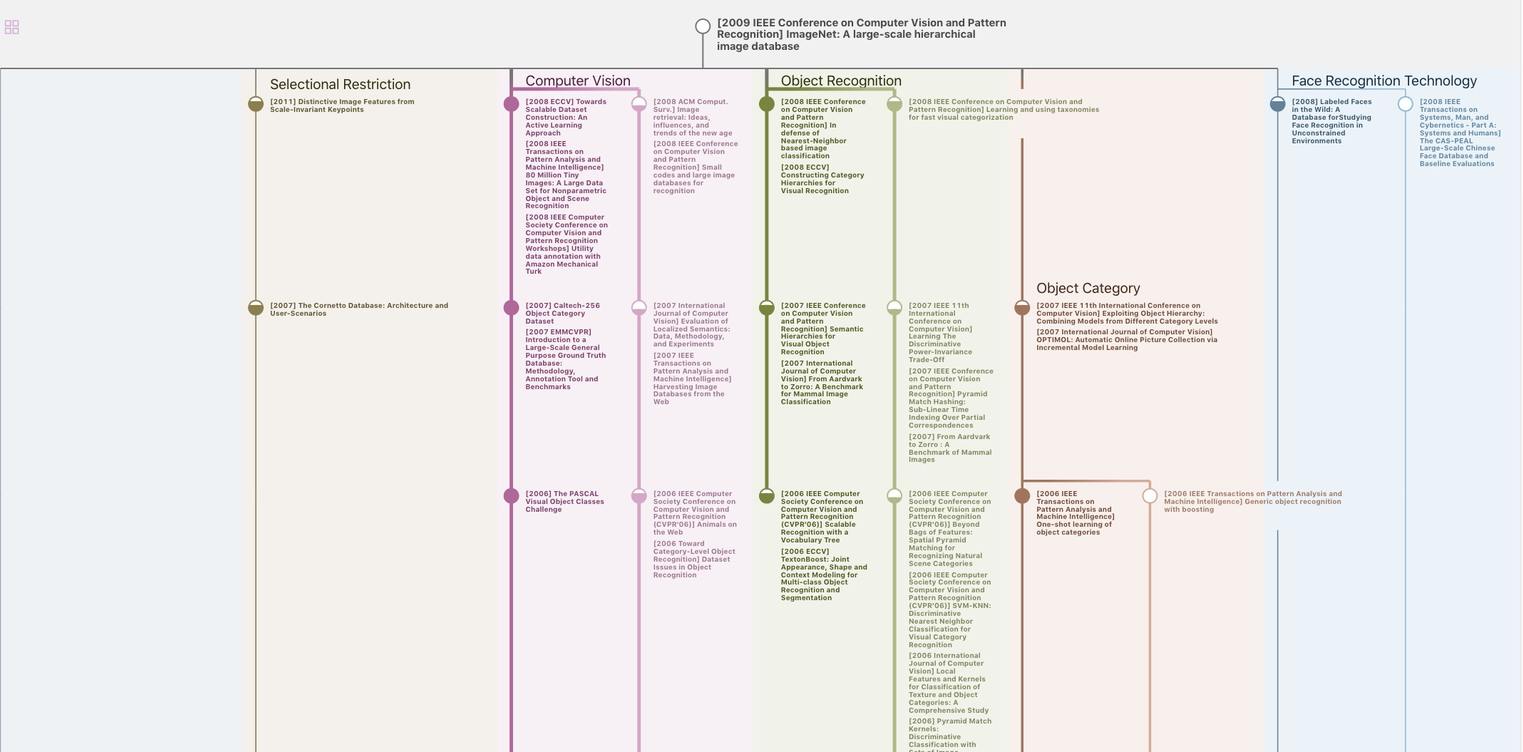
生成溯源树,研究论文发展脉络
Chat Paper
正在生成论文摘要