A two-stage approach based on Bayesian deep learning for predicting remaining useful life of rolling element bearings
Computers and Electrical Engineering(2023)
摘要
Remaining useful life (RUL) prediction of rolling element bearings is critical to maintaining rotating machinery and lowering industrial costs. There are many RUL prediction techniques, but most of them ignore two factors that may have a significant impact on prediction accuracy. One is the detection of the first predicting time (FPT) while the other is the predictive uncertainty. This paper proposes a two-stage approach to incorporating both factors into the prediction process based on Bayesian deep learning (BDL). In stage one, the state change of the bearing is identified and the FPT is determined according to a proposed detection technique. In stage two, RUL prediction is performed according to a new BDL model, and the results provide RUL point estimates and quantification of predictive uncertainty. The proposed two-stage approach has been validated on two publicly available bearing datasets, and the experimental results have demonstrated the effectiveness of the proposed approach in detecting FPT and its superiority over competitive BDL models.
更多查看译文
关键词
Remaining useful life, Bayesian deep learning, First predicting time, Rolling element bearings
AI 理解论文
溯源树
样例
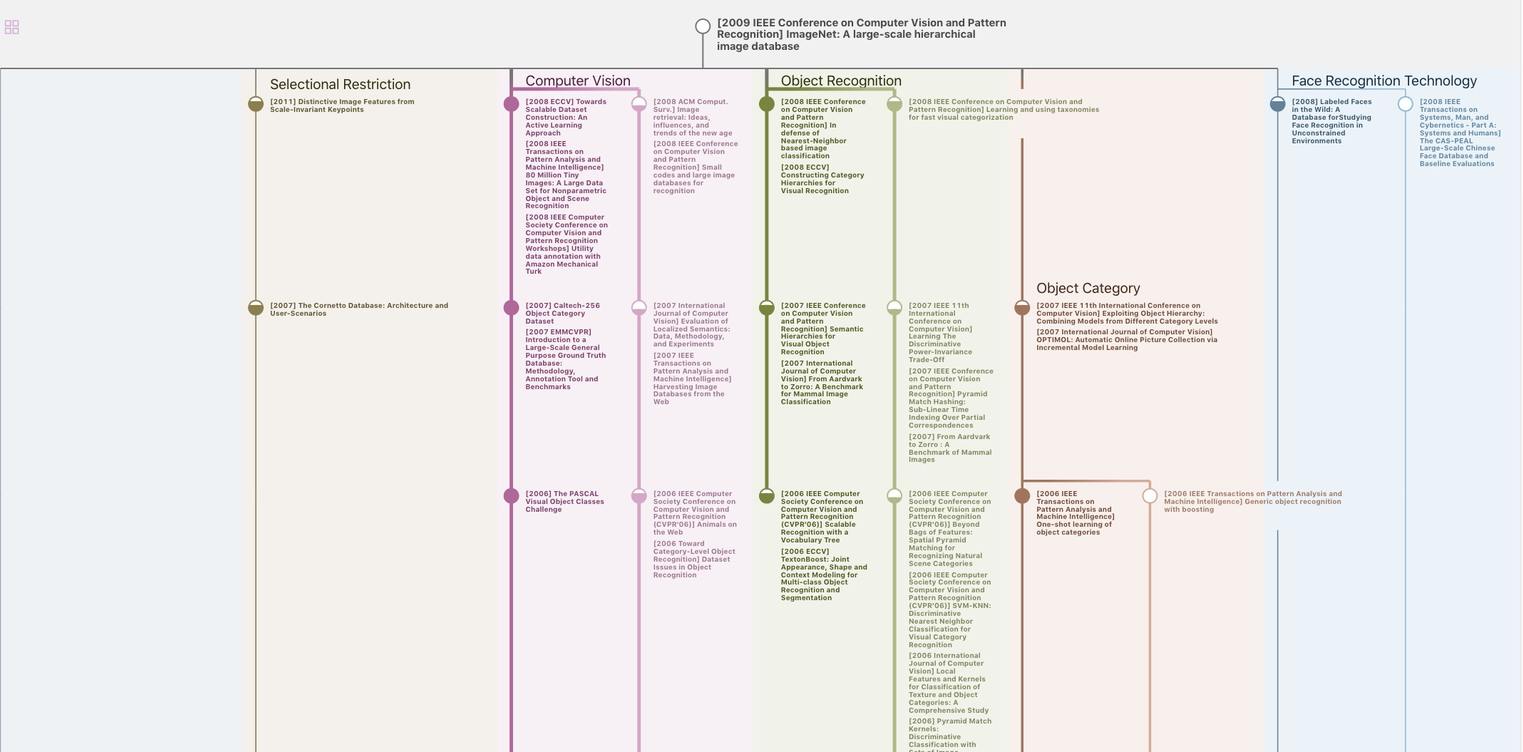
生成溯源树,研究论文发展脉络
Chat Paper
正在生成论文摘要