Optimal -k nearest neighbours based ensemble for classification and feature selection in chemometrics data
Chemometrics and Intelligent Laboratory Systems(2023)
摘要
There are various machine-learning techniques available for classification and regression tasks. The k-nearest neighbours (k-NN) method is a well-recognized algorithm that is used for both regression and classification problems. It identifies a group of knearest observations to a given test point, reducing the impact of outliers in the training dataset. For regression, the mean value is calculated, while for classification, the majority value is determined. This study proposes a novel ensemble approach that constructs k-NN models using bootstrap samples from the training data and a randomly selected subset of features. Stepwise logistic regression is then applied to the nearest neighbours identified by each k-NN model to estimate the test observations. The final estimation for the test point's response is made through a majority voting approach using the estimates from different k-NN models. The performance of the proposed method is compared to other methods using five benchmark datasets, using Brier score, sensitivity, and accuracy as performance metrics. The results indicate that the proposed ensemble method outperforms the other methods across most of the datasets. Additionally, the proposed ensemble method is used for feature selection and compared with four other feature selection methods using 9 benchmark datasets. The results demonstrate that the proposed method exhibits superior performance compared to the other methods.
更多查看译文
关键词
K-nearest neighbours (k-NN),Random k-NN,Classification,Stepwise model selection,Ensemble learning,Non-informative features
AI 理解论文
溯源树
样例
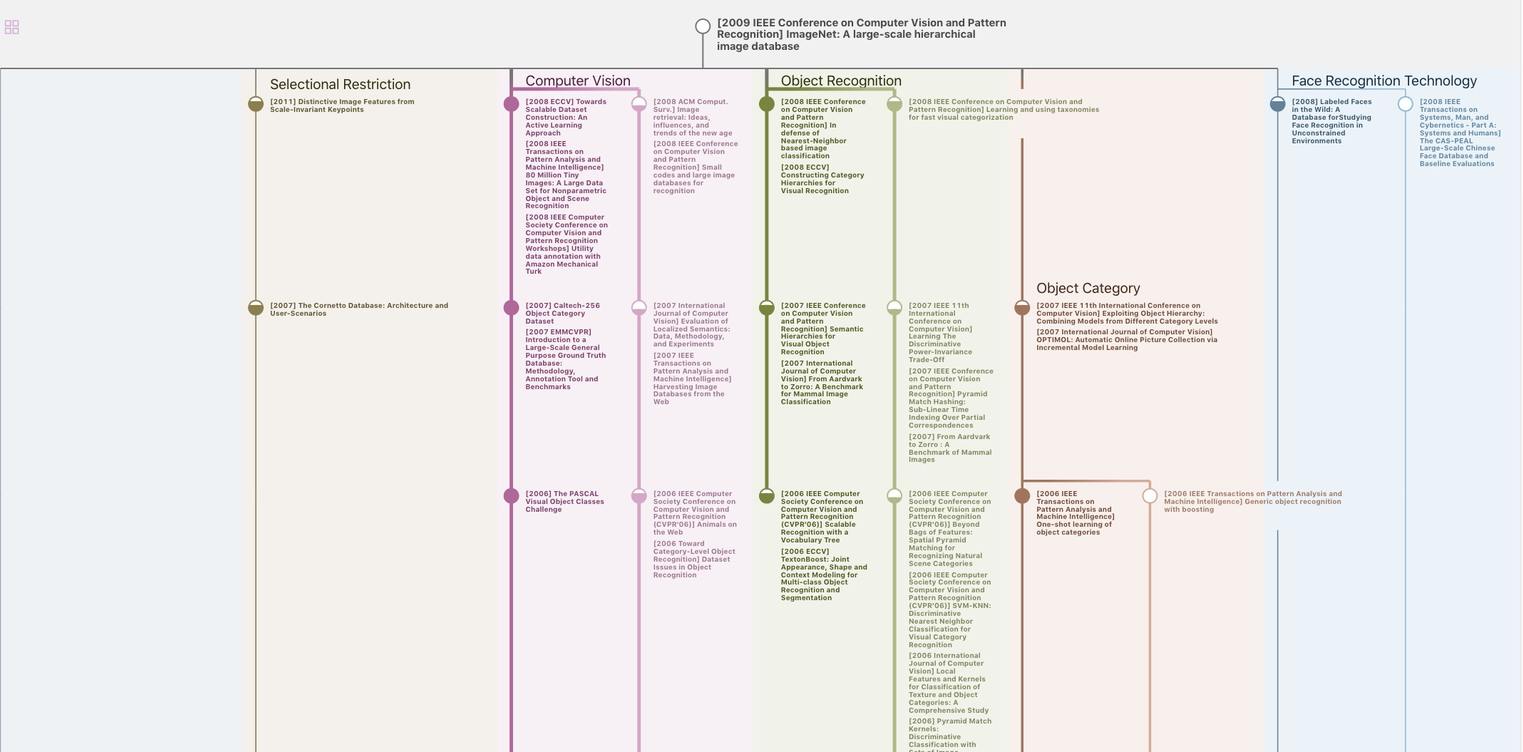
生成溯源树,研究论文发展脉络
Chat Paper
正在生成论文摘要