Pulmonary nodule detection based on Hierarchical-Split HRNet and feature pyramid network with atrous convolution
Biomedical Signal Processing and Control(2023)
摘要
Accurate pulmonary nodule detection is crucial to the diagnosis of lung diseases. In this work, we propose an end-to-end network for pulmonary nodule detection mainly consisting of pre-processing, detection modules for candidate prediction, and a discriminator to identify the existence of nodules. In the detection module, HS-HRNet is proposed to fulfill feature extraction on high-resolution input for pulmonary nodules that occupy tiny spaces of CT images. HS-HRNet incorporates plug-and-play Hierarchical-Split block into High-Resolution Network (HRNet) and modifies STEM with sandglass module. The main advantages of these modifications are that HS-HRNet can largely promote feature representation ability by split and concatenation operation with no significant increase in computation. In addition, a novel Feature Pyramid Network with Atrous Convolution (AC-FPN) is proposed for multi-scale feature fusion and multi-level prediction. This design allows context and spatial information in different feature levels to be leveraged and extracted under larger receptive fields. Besides, a discriminator replaces false positive reduction modules in most pulmonary detection methods. The discriminator judges the existence of nodules and back-propagates prediction proposals to detection modules through adversarial training. Experiments on publicly available datasets demonstrate competitive performance in pulmonary nodule detection.
更多查看译文
关键词
Pulmonary nodule detection,Hierarchical-Split Block,High-resolution network,Feature pyramid network,Adversarial training
AI 理解论文
溯源树
样例
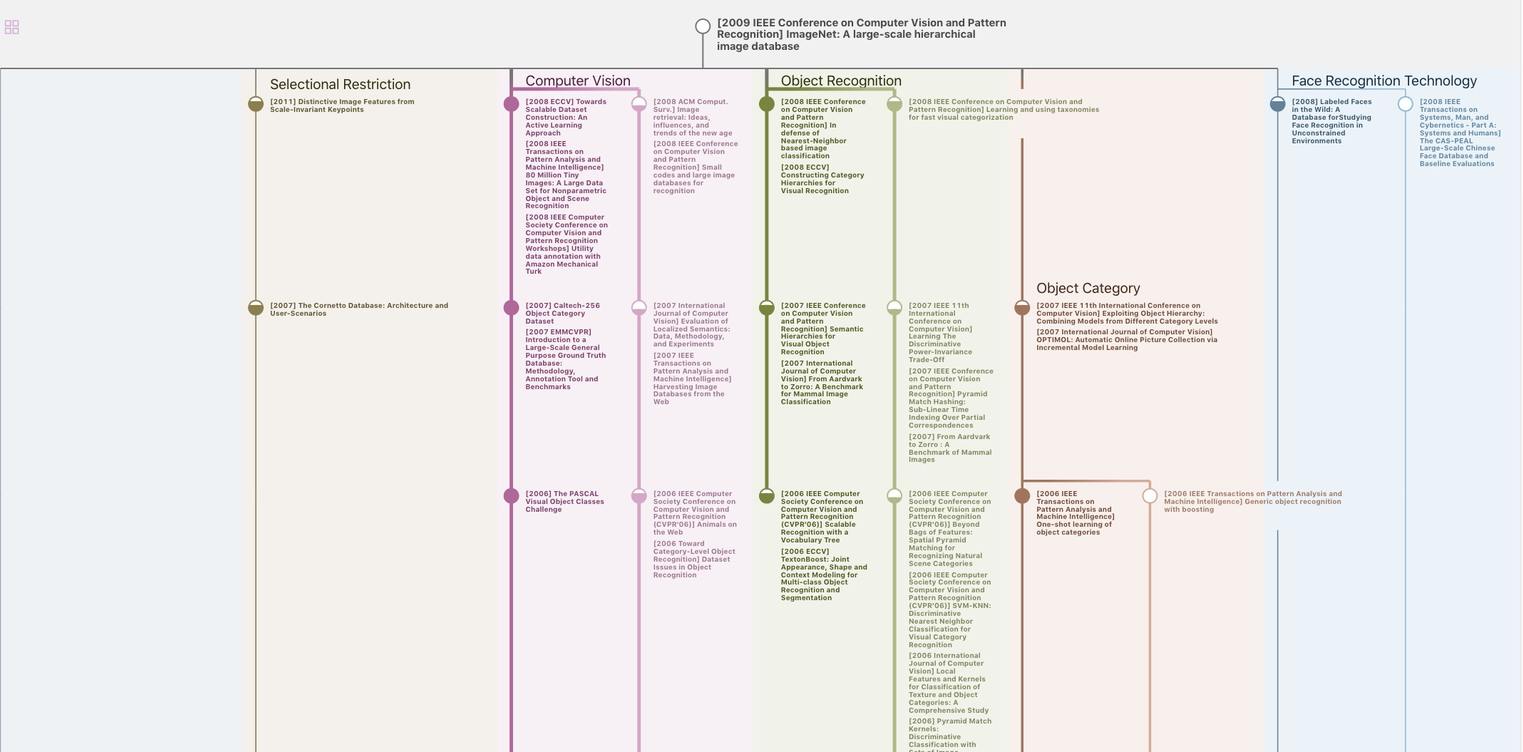
生成溯源树,研究论文发展脉络
Chat Paper
正在生成论文摘要