Few-shot multi-modal registration with mono-modal knowledge transfer
Biomedical Signal Processing and Control(2023)
摘要
Multi-modal registration is a key problem in many medical image analysis applications. Recent learning-based deformable image registration methods become attractive alternatives to traditional methods because of their great performance and fast run time. However, their success relies on large training datasets that are rarely available in multi-modal registration scenarios. To address this, we propose a novel knowledge transfer-based network (KT-Net) for few-shot multi-modal registration, which focuses on transferring knowledge of the mono-modal registration model to multi-modal registration. The contributions can be two-fold: (1) we propose model decoupling to disentangle the registration model into a feature learning network and an alignment learning network. The two networks are trained on large mono-modal datasets, making preparations for knowledge transfer. (2) a reverse teaching strategy is further designed to align the features of multi-modal images with a few samples, enabling the knowledge from mono-modal registration to transfer to multi-modal registration. Experimental results on multi-contrast brain MRI datasets demonstrate that our proposed method yields accurate and robust registration performance under the constraint of a few multi-modal samples. Compared with state-of-the-art registration methods, our proposed method achieves better registration performance with a high average Dice score of up to 83.5% and an average 95% percentile of Hausdorff distance as low as 1.26mm in various anatomical structures, showing great potential for mono-modal knowledge transfer to be applied in few-shot multi-modal registration.
更多查看译文
关键词
registration,knowledge,few-shot,multi-modal,mono-modal
AI 理解论文
溯源树
样例
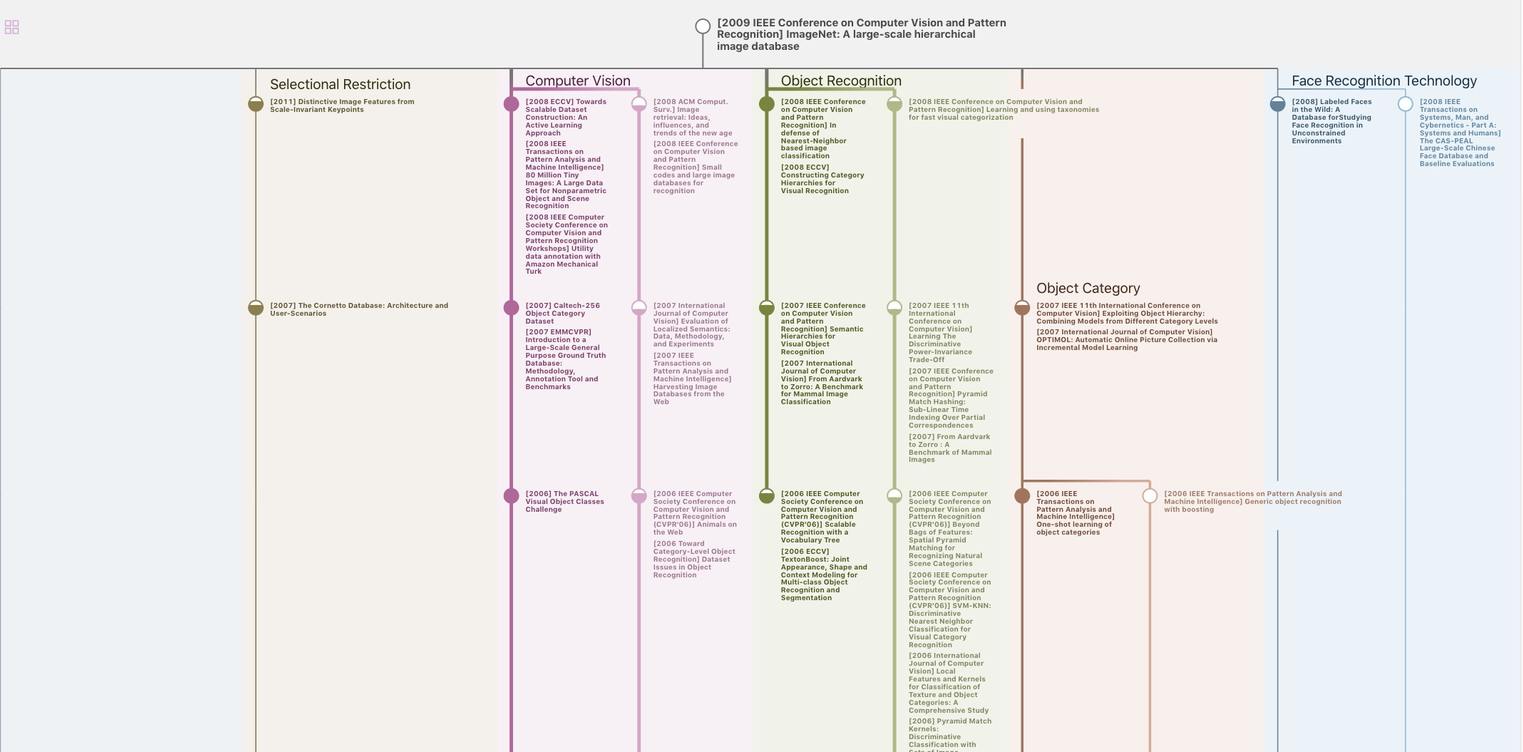
生成溯源树,研究论文发展脉络
Chat Paper
正在生成论文摘要