Comparison of chemometric models using Raman spectroscopy for offline biochemical monitoring throughout the VLP-making upstream process
BIOCHEMICAL ENGINEERING JOURNAL(2023)
Abstract
This work aimed to compare the predictive capacity of linear (Partial Least Squares; Principal Component Regression) and non-linear (Artificial Neural Network, ANN; Support Vector Machine, SVM) chemometric models from Raman spectroscopy spectra acquired offline with (unclarified samples) and without cells (clarified samples), and biochemical parameters (Viable cell density, cell viability, glucose, lactate, glutamine, glutamate, ammonium, and potassium) quantified by reference methods in a rabies VLP production bioprocess. The nonlinear models, ANN and SVM were the more suitable models with the lowest absolute errors. No effective differences in accuracy were detected for models from spectral data with and without cells. The mean absolute error of the best models within the assessed parameter ranges for viable cell density (0.01-8.83 x 106 cells/mL), cell viability (1.3-100.0%), glucose (5.22-10.93 g L-1), lactate (18.6-152.7 mg L-1), glutamine (158-1761 mg L-1), glutamate (807.6-2159.7 mg L-1), ammonium (62.8-117.8 mg L-1), and potassium (531.6-685. 3 mg L-1) were 0.17 & PLUSMN; 0.13 x 106 cells/mL, 6.8 & PLUSMN; 7.1%, 0.24 & PLUSMN; 0.14 gL- 1, 3.3 & PLUSMN; 3.5 mgL- 1, 61 & PLUSMN; 52 mgL- 1, 52 & PLUSMN; 51 mg L- 1, 2.1 & PLUSMN; 1.9 mg L- 1 and 8.6 & PLUSMN; 8.6 mg L- 1, respectively. The errors achieved were like those for bioprocesses using a highlighted animal cell host (CHO cell) in similar ranges.
MoreTranslated text
Key words
Bioprocess Monitoring,Virus-Like Particles,Partial Least Squares,Principal Component Regression,Artificial Neural Network,Support Vector Machine
AI Read Science
Must-Reading Tree
Example
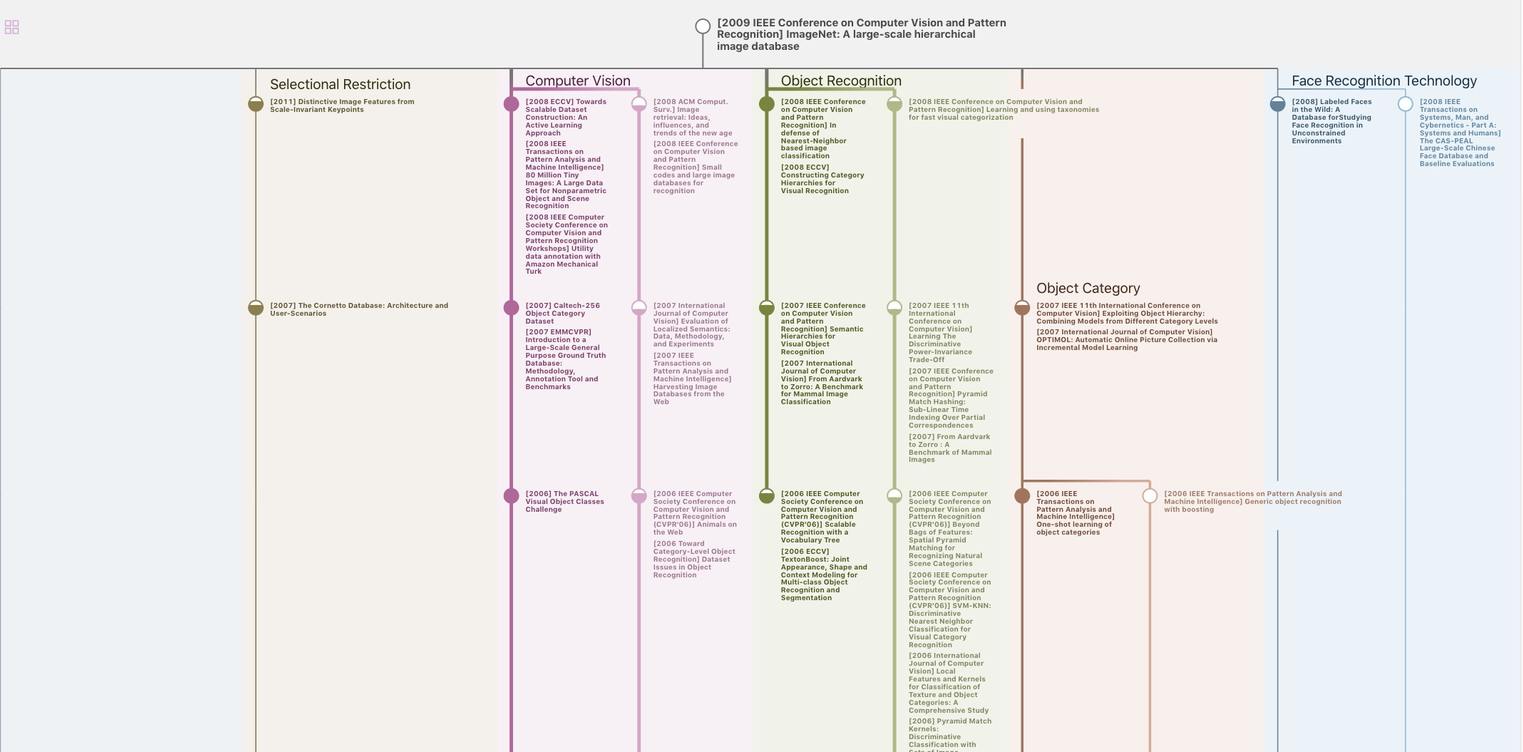
Generate MRT to find the research sequence of this paper
Chat Paper
Summary is being generated by the instructions you defined