Airfield concrete pavement joint detection network based on dual-modal feature fusion
Automation in Construction(2023)
摘要
To solve the problem of inaccurate positioning of airfield pavement concrete joint and misalignment quantification, this study proposes an airfield concrete pavement joint detection network based on dual-modal feature fusion (ACJD-DFF) and a misalignment quantification method. First, outlier cleaning and row-level distortion correction are proposed to improve the quality of 3D data. Subsequently, data from two modalities are fused to create a dual-modal feature fusion matrix dataset. An ACJD-DFF is proposed to accomplish the positioning of the concrete joint by integrating positioning coordinate optimization. Finally, by mapping the accurate joint positioning coordinate by ACJD-DFF, the misalignment quantification of the concrete joint is constructed. The results show that the ACJD-DFF performs better than the state-of-the-art methods. The mAP@0.5 and Recall reached 96.38%, 91.4%. The average error of misalignment quantification is 4.333%. These methods have been applied in routine airfield pavement monitoring and are important for later detection of concrete joint distress.
更多查看译文
关键词
Airfield pavement, Concrete joint detection, Dual -modal, Feature fusion, Positioning coordinate optimization, Misalignment quantification
AI 理解论文
溯源树
样例
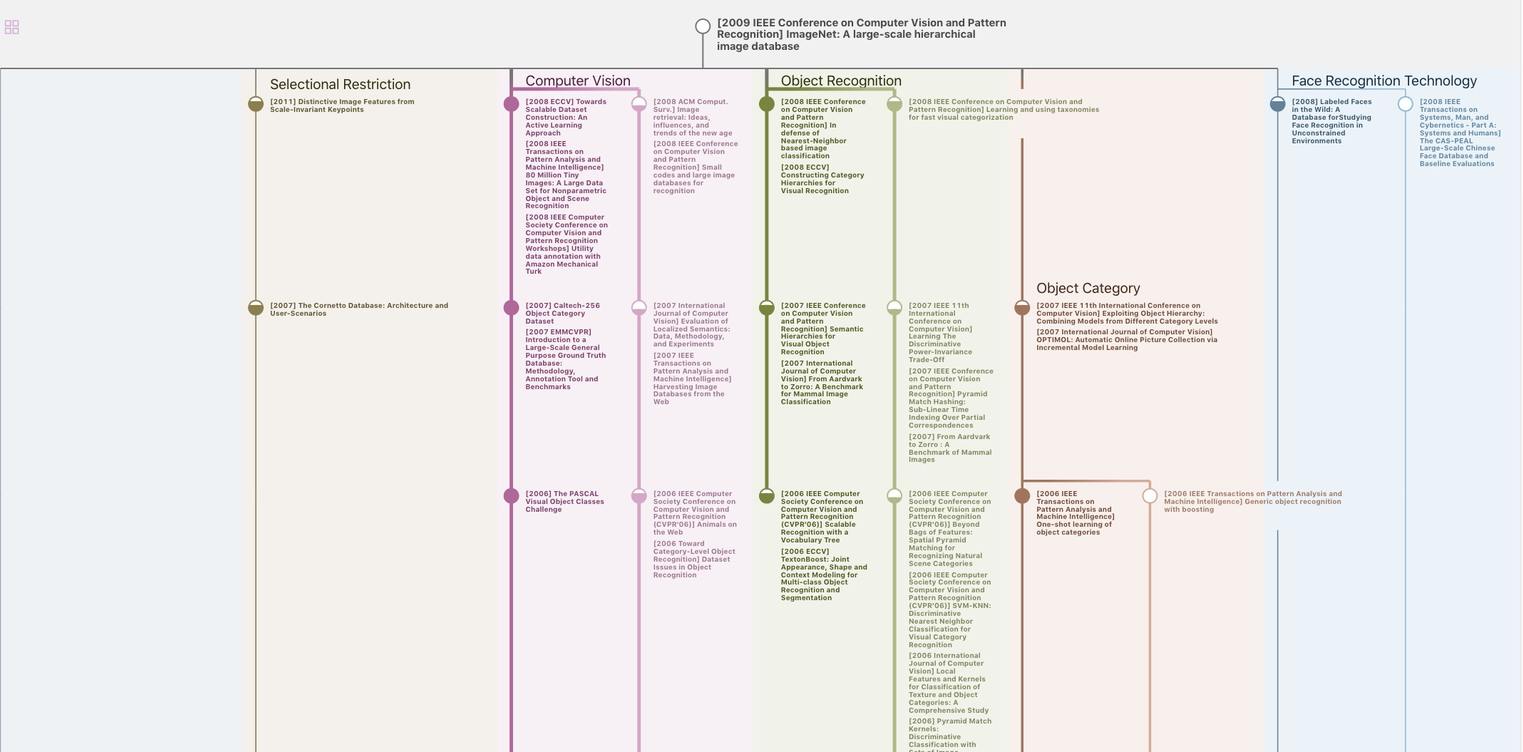
生成溯源树,研究论文发展脉络
Chat Paper
正在生成论文摘要