A novel approach for brain tissue segmentation and classification in infants' MRI images based on seeded region growing, foster corner detection theory, and sparse autoencoder
Alexandria Engineering Journal(2023)
Abstract
Brain tissue segmentation and classification in infant MRI images play a crucial role in early diagnosis of neurological disorders. In this paper, we propose a novel approach based on Seeded Region Growing, Foster Corner Detection Theory, and Sparse Autoencoder. Proposed method incorporates advantages of these techniques to improve segmentation and classification accuracy of infant brain MRI. An unsupervised segmentation method proposed in this study has four stages. First stage involves pre-processing input image by removing noise using a non-local means method and rectifying intensity inhomogeneity field employing Contrast-limited adaptive histogram equalization. In second stage, modified and optimized approach of region growth is utilized, where Forstner Corner Identification principle is used to choose and position seeds to a producer. Third stage involves applying the region growth technique to image, where similarity criterion is optimized using Predator Prey algorithm. In fourth stage, tissues are classified using weighted KNN and Sparse Auto Encoder classifiers. Result shows high Dice factor of 93%, outperforming existing works. This method improves accuracy of brain tissue segmentation and classification, leading to better clinical decision-making. Applications of proposed method include early detection and diagnosis of neurological disorders, monitoring of disease progression, and assessment of treatment effectiveness. (c) 2023 THE AUTHORS. Published by Elsevier BV on behalf of Faculty of Engineering, Alexandria University. This is an open access article under the CC BY-NC-ND license (http://creativecommons.org/ licenses/by-nc-nd/4.0/).
MoreTranslated text
Key words
Contrast limited adaptive histogram equalization,Seeded region grow algo-rithm,Forstner corner theory,PP optimization,Weighted KNN,SAE
AI Read Science
Must-Reading Tree
Example
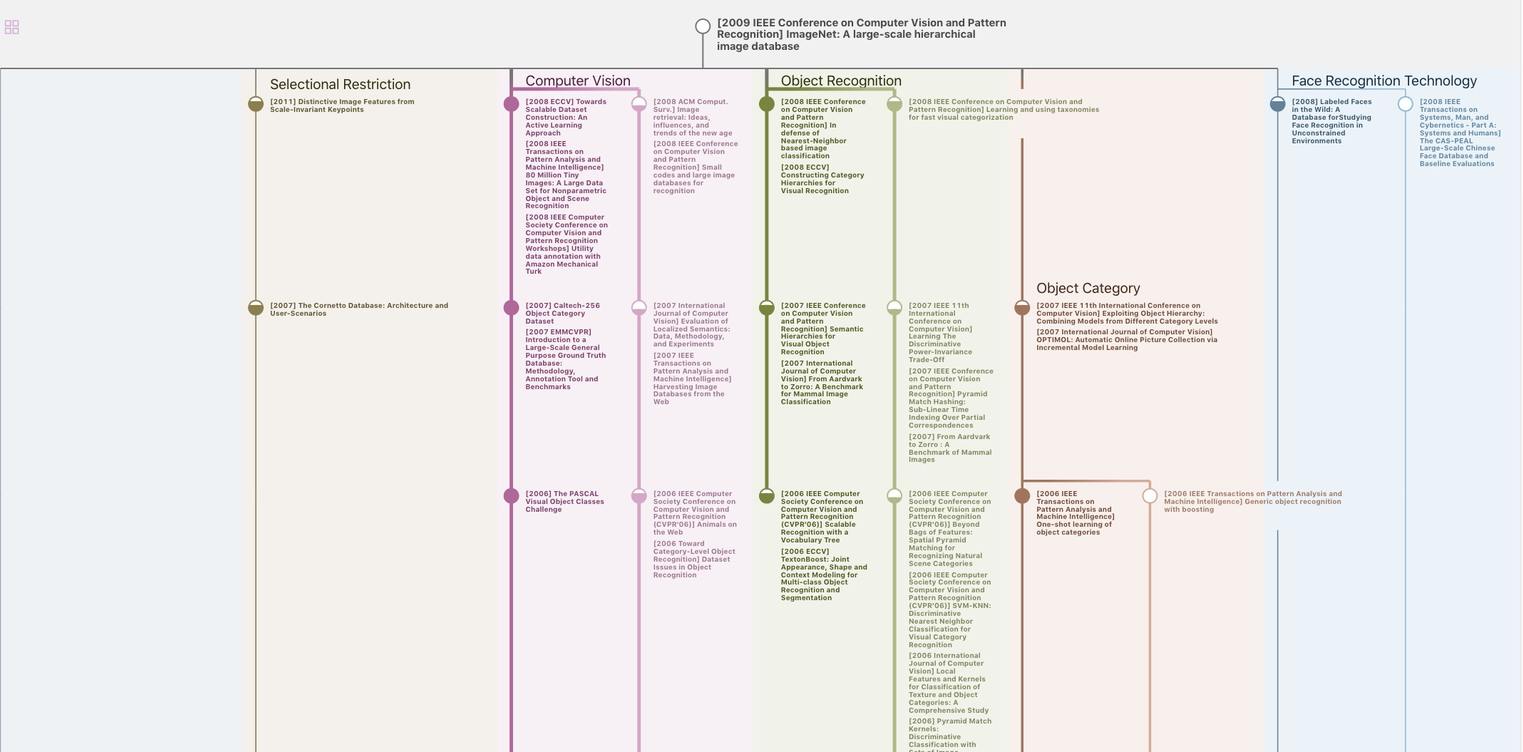
Generate MRT to find the research sequence of this paper
Chat Paper
Summary is being generated by the instructions you defined