BLEURT Has Universal Translations: An Analysis of Automatic Metrics by Minimum Risk Training
conf_acl(2023)
摘要
Automatic metrics play a crucial role in machine translation. Despite the widespread use of n-gram-based metrics, there has been a recent surge in the development of pre-trained model-based metrics that focus on measuring sentence semantics. However, these neural metrics, while achieving higher correlations with human evaluations, are often considered to be black boxes with potential biases that are difficult to detect. In this study, we systematically analyze and compare various mainstream and cutting-edge automatic metrics from the perspective of their guidance for training machine translation systems. Through Minimum Risk Training (MRT), we find that certain metrics exhibit robustness defects, such as the presence of universal adversarial translations in BLEURT and BARTScore. In-depth analysis suggests two main causes of these robustness deficits: distribution biases in the training datasets, and the tendency of the metric paradigm. By incorporating token-level constraints, we enhance the robustness of evaluation metrics, which in turn leads to an improvement in the performance of machine translation systems. Codes are available at \url{https://github.com/powerpuffpomelo/fairseq_mrt}.
更多查看译文
关键词
automatic metrics,universal translations,risk
AI 理解论文
溯源树
样例
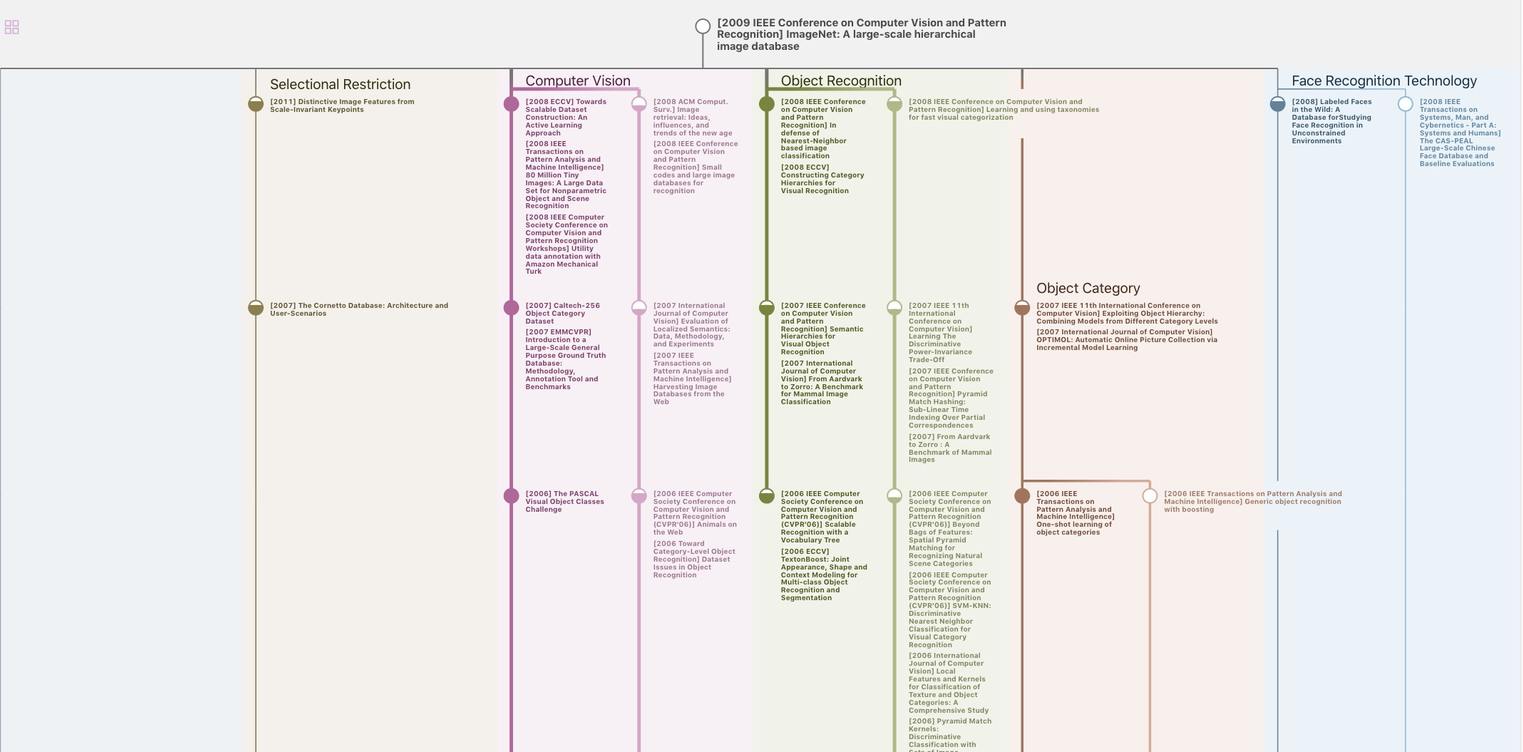
生成溯源树,研究论文发展脉络
Chat Paper
正在生成论文摘要