Pruning vs Quantization: Which is Better?
arXiv (Cornell University)(2023)
摘要
Neural network pruning and quantization techniques are almost as old as neural networks themselves. However, to date only ad-hoc comparisons between the two have been published. In this paper, we set out to answer the question on which is better: neural network quantization or pruning? By answering this question, we hope to inform design decisions made on neural network hardware going forward. We provide an extensive comparison between the two techniques for compressing deep neural networks. First, we give an analytical comparison of expected quantization and pruning error for general data distributions. Then, we provide lower bounds for the per-layer pruning and quantization error in trained networks, and compare these to empirical error after optimization. Finally, we provide an extensive experimental comparison for training 8 large-scale models on 3 tasks. Our results show that in most cases quantization outperforms pruning. Only in some scenarios with very high compression ratio, pruning might be beneficial from an accuracy standpoint.
更多查看译文
关键词
quantization
AI 理解论文
溯源树
样例
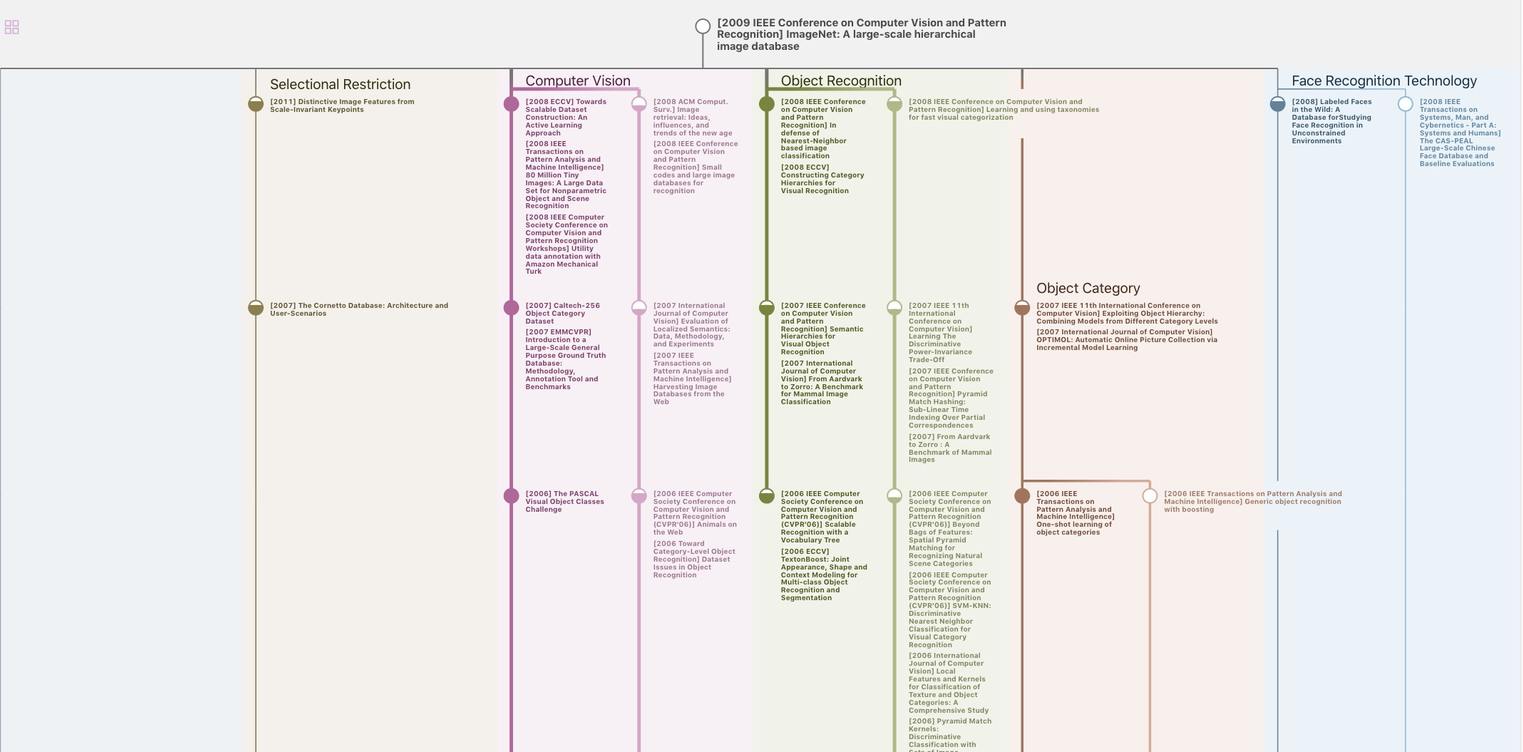
生成溯源树,研究论文发展脉络
Chat Paper
正在生成论文摘要