The Role of Subgroup Separability in Group-Fair Medical Image Classification
MEDICAL IMAGE COMPUTING AND COMPUTER ASSISTED INTERVENTION, MICCAI 2023, PT III(2023)
摘要
We investigate performance disparities in deep classifiers. We find that the ability of classifiers to separate individuals into subgroups varies substantially across medical imaging modalities and protected characteristics; crucially, we show that this property is predictive of algorithmic bias. Through theoretical analysis and extensive empirical evaluation (Code is available at https://github.com/biomedia-mira/subgroup-separability), we find a relationship between subgroup separability, subgroup disparities, and performance degradation when models are trained on data with systematic bias such as underdiagnosis. Our findings shed new light on the question of how models become biased, providing important insights for the development of fair medical imaging AI.
更多查看译文
关键词
subgroup separability,classification,group-fair
AI 理解论文
溯源树
样例
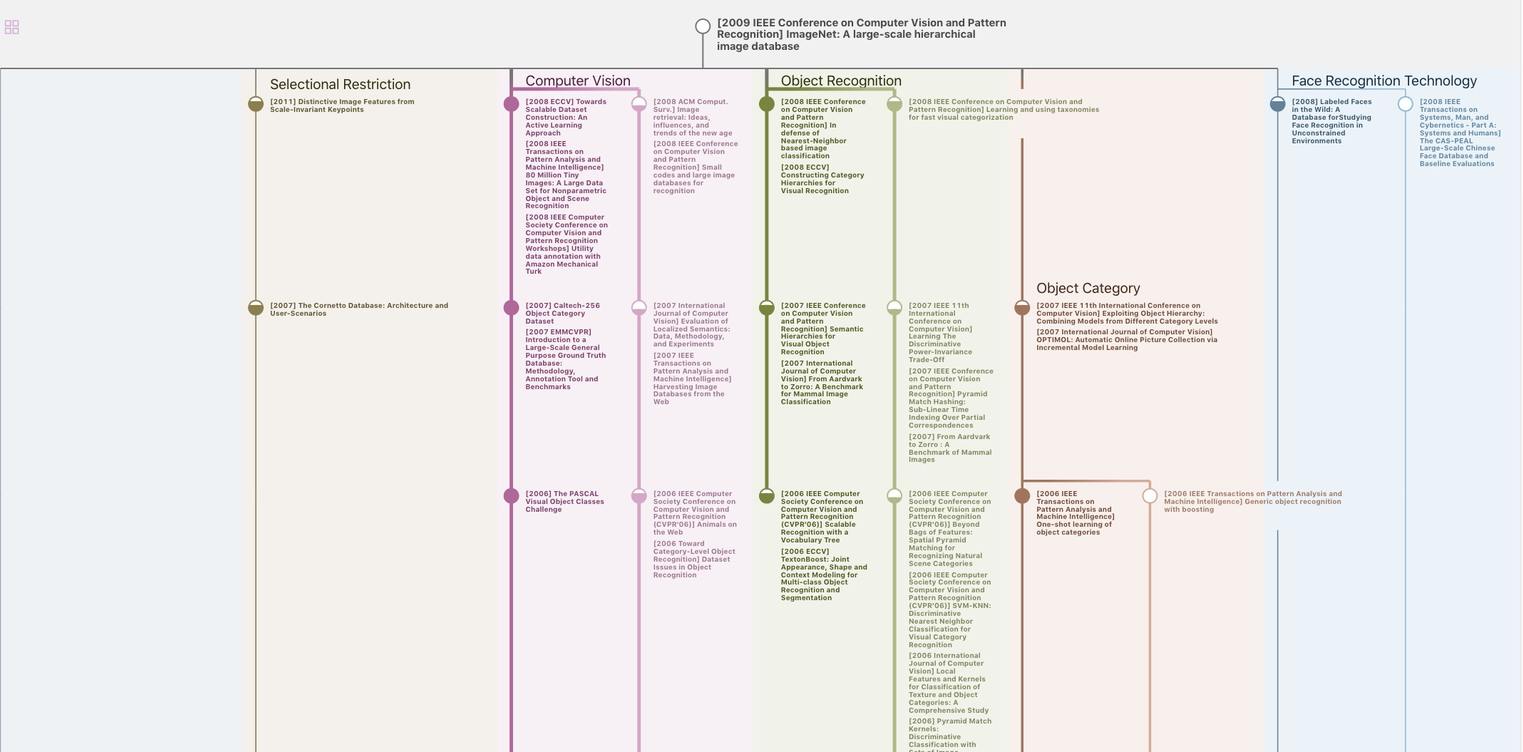
生成溯源树,研究论文发展脉络
Chat Paper
正在生成论文摘要