Real-time Workload Pattern Analysis for Large-scale Cloud Databases
VLDB 2023(2023)
摘要
Hosting database services on cloud systems has become a common practice. This has led to the increasing volume of database workloads, which provides the opportunity for pattern analysis. Discovering workload patterns from a business logic perspective is conducive to better understanding the trends and characteristics of the database system. However, existing workload pattern discovery systems are not suitable for large-scale cloud databases which are commonly employed by the industry. This is because the workload patterns of large-scale cloud databases are generally far more complicated than those of ordinary databases. In this paper, we propose Alibaba Workload Miner (AWM), a real-time system for discovering workload patterns in complicated large-scale workloads. AWM encodes and discovers the SQL query patterns logged from user requests and optimizes the querying processing based on the discovered patterns. First, Data Collection & Preprocessing Module collects streaming query logs and encodes them into high-dimensional feature embeddings with rich semantic contexts and execution features. Next, Online Workload Mining Module separates encoded queries by business groups and discovers the workload patterns for each group. Meanwhile, Offline Training Module collects labels and trains the classification model using the labels. Finally, Pattern-based Optimizing Module optimizes query processing in cloud databases by exploiting discovered patterns. Extensive experimental results on one synthetic dataset and two real-life datasets (extracted from Alibaba Cloud databases) show that AWM enhances the accuracy of pattern discovery by 66% and reduce the latency of online inference by 22%, compared with the state-of-the-arts.
更多查看译文
关键词
workload,pattern,real-time,large-scale
AI 理解论文
溯源树
样例
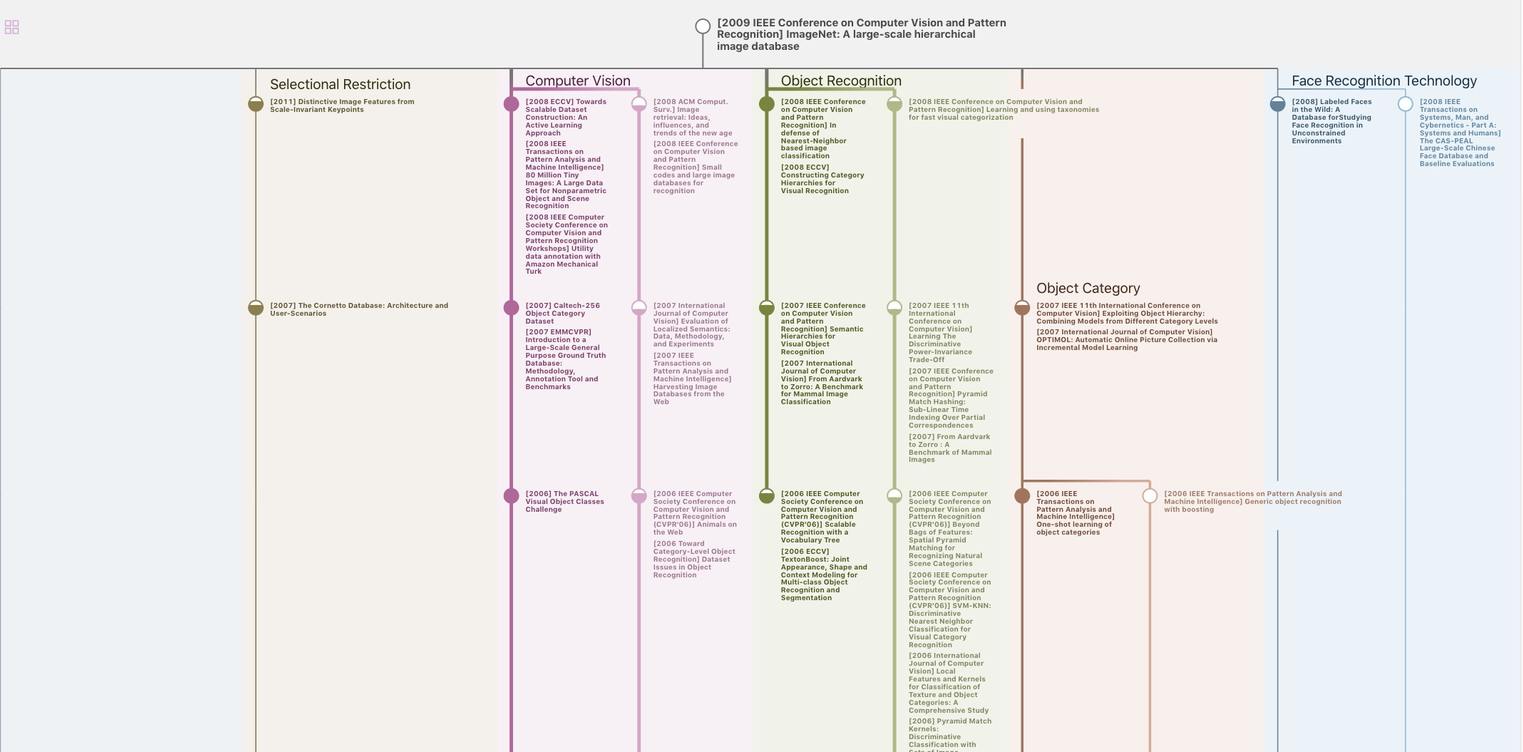
生成溯源树,研究论文发展脉络
Chat Paper
正在生成论文摘要