Deriving hydrological drought indicators based on a GRACE-assimilated global hydrological model
crossref(2020)
摘要
<p class="western" align="justify"><span><span lang="en-US">Detecting and quantifying hydrological drought in hindcast plays an important role for understanding its negative impacts on water supply and agricultural systems. Obser</span><span lang="en-US">vation of</span><span lang="en-US">, for example, streamflow or groundwater storage decline would be required for drought detection, but is often restricted </span><span lang="en-US">due to</span><span lang="en-US"> inaccessibility or irregular spatial and temporal coverage of in-situ data. At this point, hydrological models can help to provide information about surface and subsurface storages. However, models do not perfectly represent reality because they are subject to assumptions and very sensitive to uncertainties of input data.</span></span></p> <p class="western" align="justify"><span><span lang="en-US">At larger spatial scales, the gravity satellite mission GRACE (Gravity Recovery And Climate Experiment) offers a possibility to observe total water storage </span><span lang="en-US">anomalies</span><span lang="en-US"> (TWS</span><span lang="en-US">A</span><span lang="en-US">), which also contain surface and subsurface storages. A number of indicators for hydrological drought based on GRACE TWS</span><span lang="en-US">A</span><span lang="en-US"> have been developed and applied to detect drought events in different parts of the world. But the application of GRACE TWS</span><span lang="en-US">A</span><span lang="en-US"> data is severely hampered by its sparse spatial resolution of about 300 km and it does not allow to distinguish separately storage declines in different hydrological compartments like snow, soil, groundwater and surface water bodies.</span></span></p> <p class="western" align="justify"><span><span lang="en-US">To overcome these limitations of the model and observation data, we developed an assimilation framework that integrates GRACE TWS</span><span lang="en-US">A</span><span lang="en-US"> into the WaterG</span><span lang="en-US">AP</span><span lang="en-US"> Hydrological Model (WGHM). The ability of spatially downscaling and disaggregating GRACE data by data assimilation opens up new opportunities for drought detection. We compare and analyze different TWS</span><span lang="en-US">A</span><span lang="en-US">-based drought indicators in the period of 2003 to 2016 for South Africa using (i) the </span><span lang="en-US">WGHM</span><span lang="en-US"> model, (ii) </span><span lang="en-US">GRACE observations</span><span lang="en-US">, and (iii) GRACE TWS</span><span lang="en-US">A</span><span lang="en-US"> integrated into WGHM. Finally, we apply the same methodology to surface and groundwater storage variability from the GRACE data assimilation. We show that the 2016 drought event was mainly related to groundwater deficit, which is more pronounced in the assimilation as compared to the model.</span></span></p>
更多查看译文
AI 理解论文
溯源树
样例
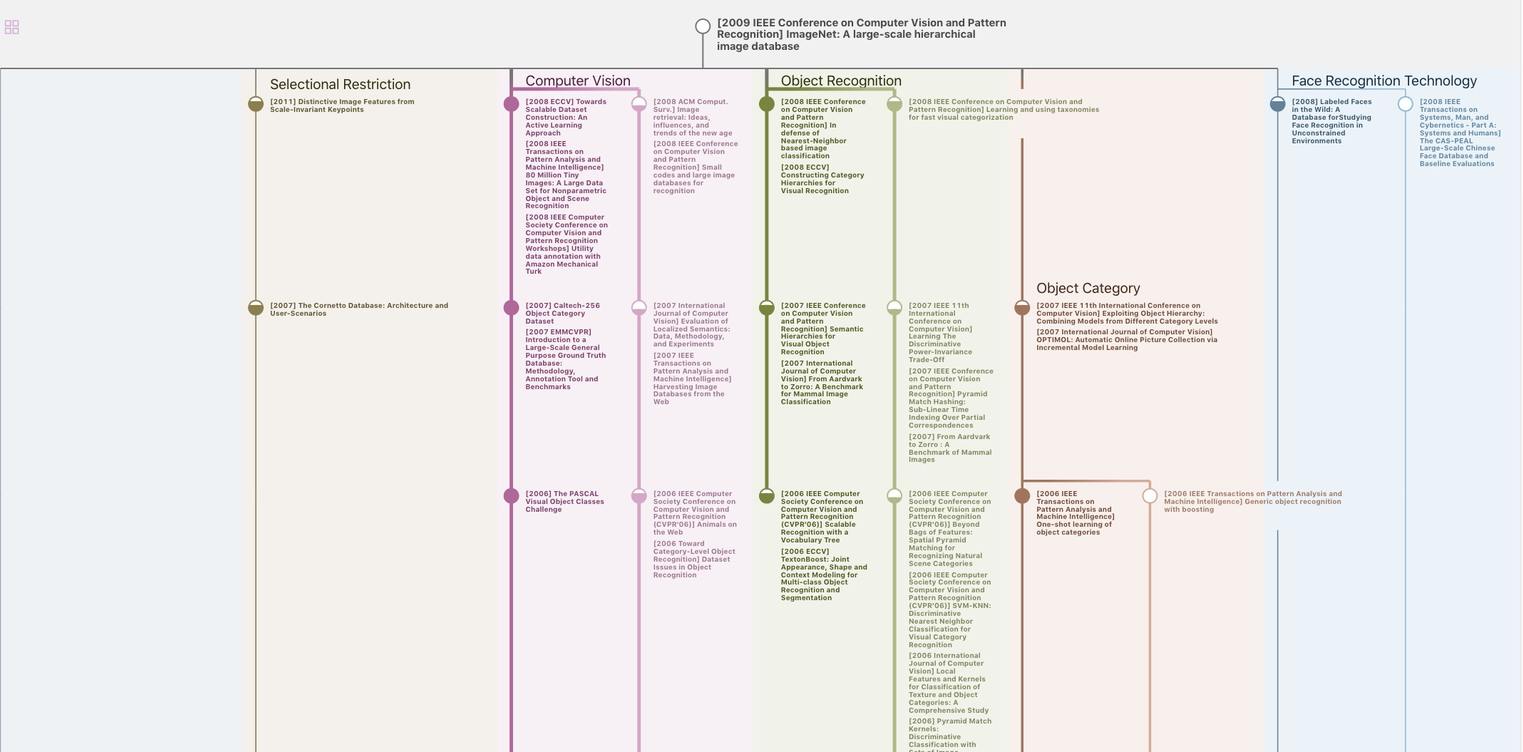
生成溯源树,研究论文发展脉络
Chat Paper
正在生成论文摘要