Comprehensive analysis of DNA methylation and gene expression reveals specific predictive biomarkers for renal cell carcinoma
Research Square (Research Square)(2020)
Abstract
Abstract Background We aimed to identify methylation-driven genes (MDGs) and predict the prognosis of clear cell renal cell carcinoma (ccRCC) based on several cohorts with high-throughput data.Methods The transcriptome profiles, 450K methylated data and corresponding clinical information were extracted from the cancer genome atlas (TCGA) database. MethylMix package was used to screen aberrant methylation events. Next, the Cox regression models were applied via survival package. Functional analysis was mainly performed based on ConsensusPathDB database. Besides, “mimifi” R package were adopted to analyze methylated alterations from three Gene Expression Omnibus (GEO) datasets. The predictive efficiency of constructed MDGs signature was assessed and validated in TCGA-KIRC and ICGC-RCC cohort, respectively. Unsupervised clustering analysis was conducted via ConsensusClusterPlus package. Moreover, MDGs-nomogram for OS prediction was conducted via glm and survival packages. Results Totally, we collected We finally identified 761 samples from TCGA-KIRC, ICGC-RCC, GSE61441, GSE105260 and GSE105261. We combined the expression data and 450K methylated data to find 5 hub prognostic MDGs (TAGLN2, PDK2, HHLA2, HOXA2, XAF1). We used the TCGA-KIRC cohort as the training dataset to verify the superior predictive significance of the 5 MDGs signature (AUC = 0.713), and validated it in ICGC-RCC cohort with AUC = 0.769. Kaplan-Meier analysis suggested that patients with high MDGs levels suffered from worse suvival outcomes. Besides, we further conducted the unsupervised clustering analysis in the whole ccRCC patients and identified the sub-cluster with the worst prognosis, indicating the MDGs could be used as efficient molecular classifier for ccRCC. We also identified 32 prognostic risk loci associated with hub MDGs in KIRC. Superior predictive efficiency was found in the MDGs-nomogram [Area Under Curve (AUC) of 3-year: 0.842, AUC of 5-year: 0.862], compared with traditional independent feature such as TNM stage (AUC of 3-year: 0.759, AUC of 5-year: 0.717). The 5 MDGs signature were mainly associated with oxygen metabolism, glycometabolism, even the HIF-1 signaling pathway. Conclusions Collectively, this study indicated several hub-MDGs and explored the prognostic value in ccRCC, which would provide new insights on the exploration of epigenetic pathogenesis and therapeutic targets for ccRCC.
MoreTranslated text
Key words
renal cell carcinoma,dna methylation,specific predictive biomarkers,gene expression
AI Read Science
Must-Reading Tree
Example
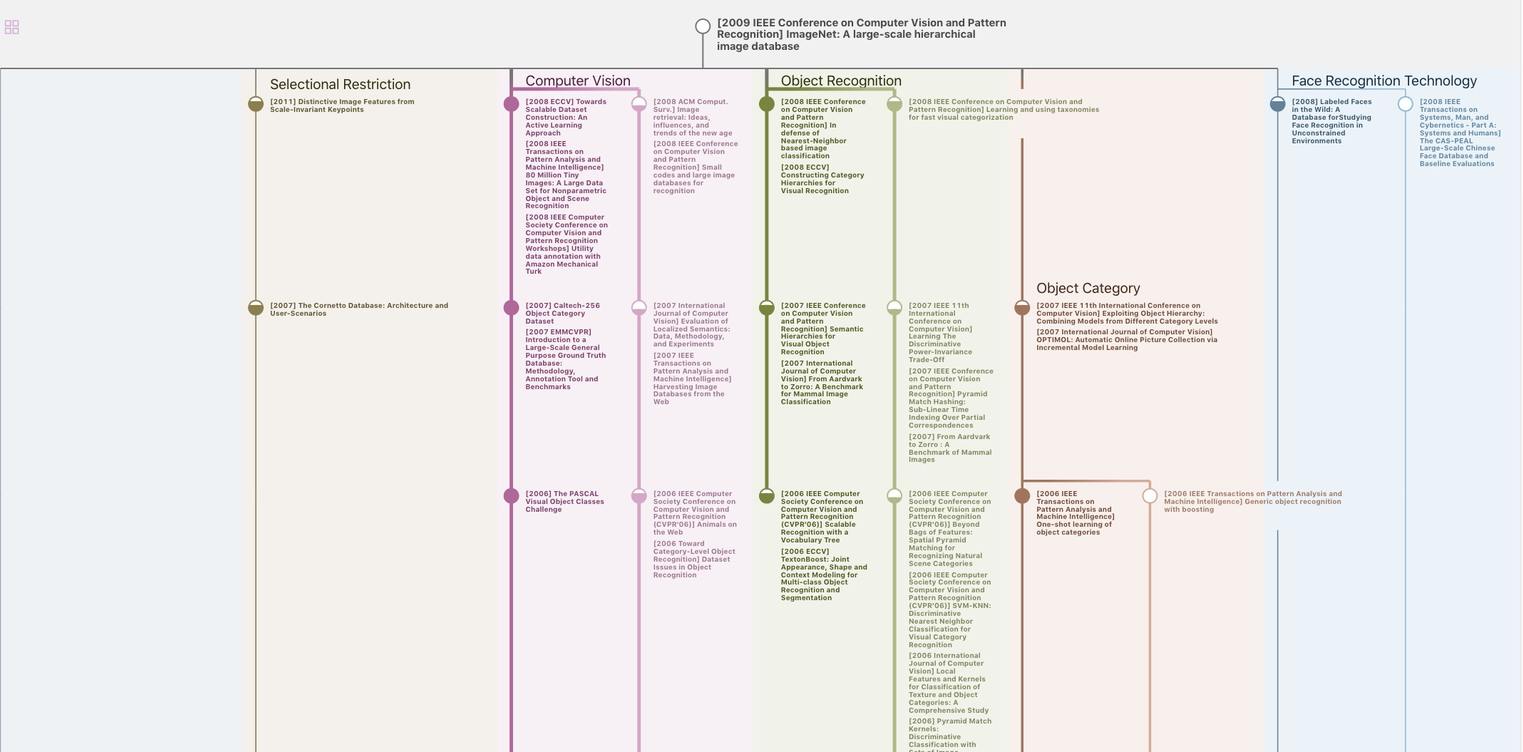
Generate MRT to find the research sequence of this paper
Chat Paper
Summary is being generated by the instructions you defined