Development and clinical application of a rapid and sensitive loop-mediated isothermal amplification test for SARS-CoV-2 infection
crossref(2020)
摘要
BackgroundThe SARS-CoV-2 outbreak urgently requires sensitive and convenient COVID-19 diagnostics for the containment and timely treatment of patients. We aimed to develop and validate a novel reverse transcription loop-mediated isothermal amplification (RT-LAMP) assay to detect SARS-CoV-2 in qualified laboratories and point-of-care settings.MethodsPatients with suspected COVID-19 and close contacts were recruited from two hospitals between Jan 26 and April 8, 2020. Respiratory samples were collected and tested using the RT-LAMP assays, and the results were compared with those obtained by RT-qPCR. Samples yielding inconsistent results between these two methods were subjected to next-generation sequencing for confirmation. The RT-LAMP assay was also tested on an asymptomatic COVID-19 carrier and patients with other respiratory viral infections.ResultsSamples were collected from a cohort of 129 cases (329 nasopharyngeal swabs) and an independent cohort of 76 patients (152 nasopharyngeal swabs and sputum samples). The RT-LAMP assay was validated to be accurate (overall sensitivity and specificity: 88.89% and 99.00%; positive and negative predictive values: 94.74% and 97.78%, respectively) and diagnostically useful (positive and negative likelihood ratios: 88.89 and 0.11, respectively). RT-LAMP showed an increased sensitivity (88.89% vs 81.48%) and high consistency (kappa 0.92) compared with RT-qPCR for SARS-CoV-2 screening while requiring only constant temperature heating and visual inspection. The time required for RT-LAMP was less than 1 h from sample preparation to result. In addition, RT-LAMP was feasible for use with asymptomatic patients and did not cross-react with other respiratory pathogens.ConclusionThe developed RT-LAMP assay offers rapid, sensitive and straightforward detection of SARS-CoV-2 infection and could aid the expansion of COVID-19 testing in the public domain and hospitals.
更多查看译文
AI 理解论文
溯源树
样例
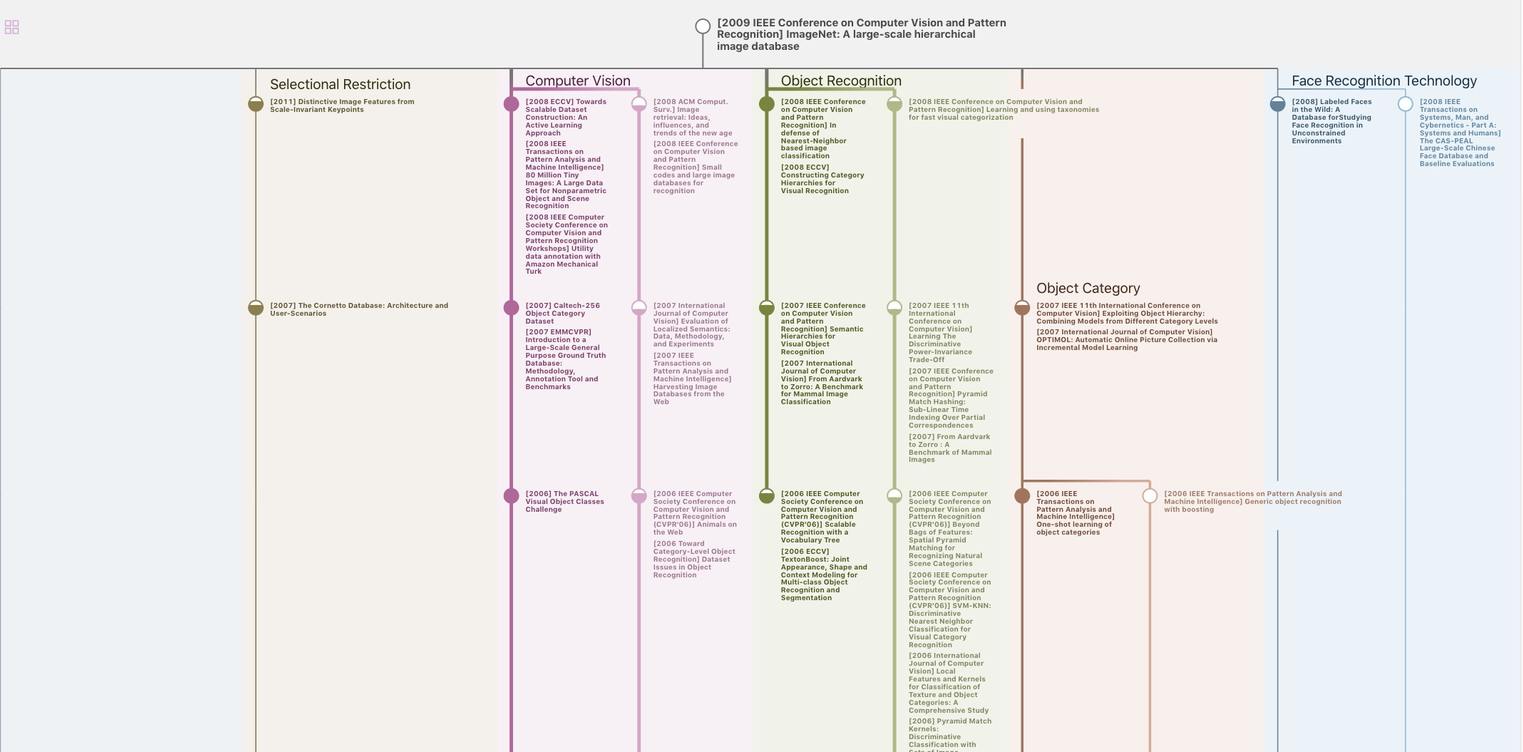
生成溯源树,研究论文发展脉络
Chat Paper
正在生成论文摘要