Improving in-text citation reason extraction and classification using supervised machine learning techniques
Computer Speech & Language(2023)
摘要
In the last decade, automatic extraction and classification of in-text citations have received immense popularity and have become one of the most frequently used techniques to evaluate research. Due to the large volume of in-text citations in various digital libraries such as Web of Science, Scopus, Google Scholar, Microsoft Academic, etc., machine learning models and natural language processing techniques are being used to extract, classify, and analyze them. Typical automatic in-text classification techniques use sentiment-based classes (Positive, Negative, and Neutral). However, there are cognitive-based schemes as well that classify in-text citations based on the author’s perspective. In such schemes, extracting citation reasons with high recall is challenging. To address this challenge, we have used eight citations’ context and reason classes defined by CCRO (Citation’s Context and Reasons Ontology) to develop a machine learning model to achieve high recall without compromising on precision. We have worked on Association for Computational Linguistics Corpus with over 7000 in-text citations, randomly annotated by experts in CCRO classes. Afterwards, an array of machine-learning models is implemented on the annotated dataset: Support Vector Machine (SVM), Naïve Bayesian (NB), and Random Forest (RF). We have used various part-of-speech (Nouns, Verbs, Adverbs, and Adjectives) as novel features. Our results show that we have outperformed the three comparative models by achieving 91% accuracy.
更多查看译文
关键词
Citation reason classification,Machine learning,Supervised learning
AI 理解论文
溯源树
样例
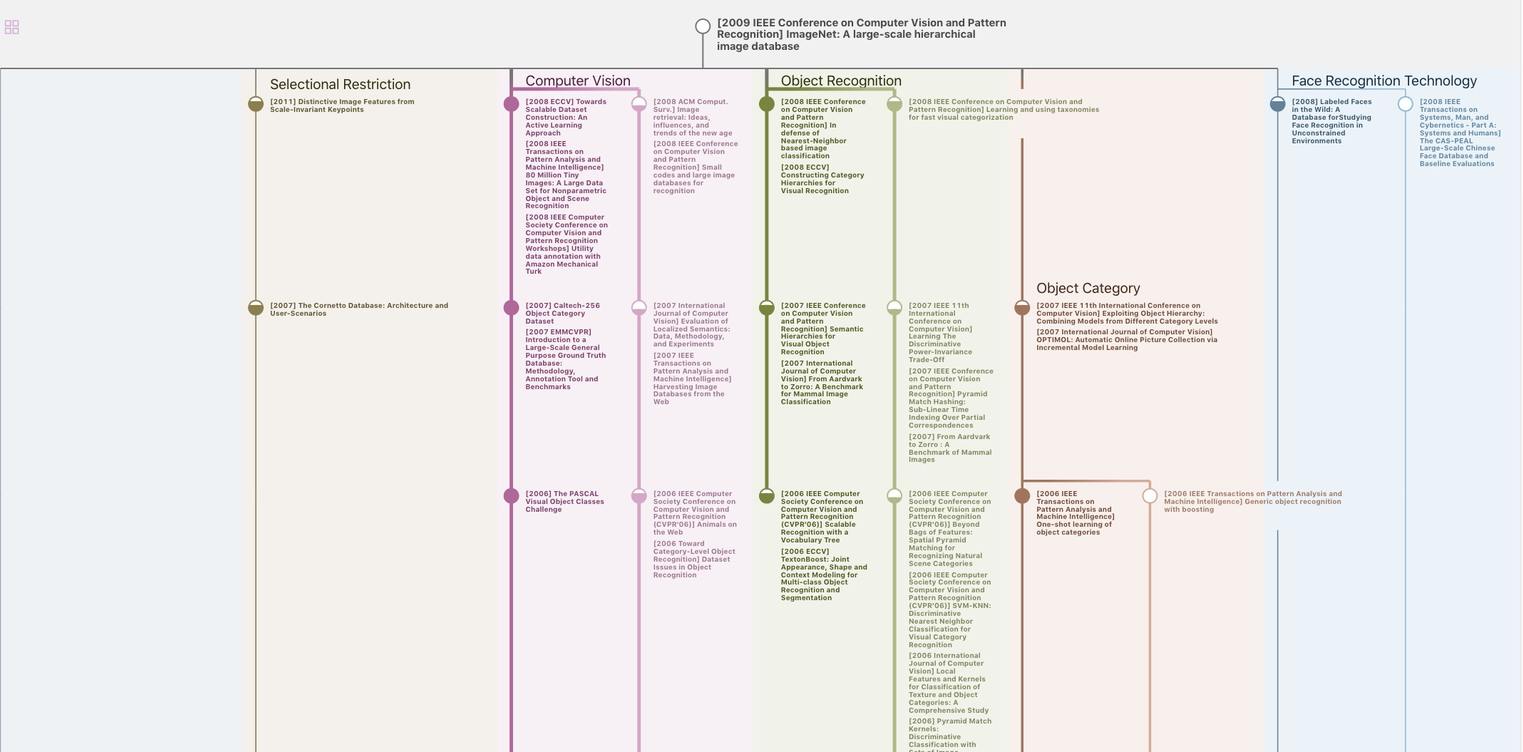
生成溯源树,研究论文发展脉络
Chat Paper
正在生成论文摘要