Local primordial non-Gaussianity from the large-scale clustering of photometric DESI luminous red galaxies
arxiv(2023)
Abstract
We use angular clustering of luminous red galaxies from the Dark Energy
Spectroscopic Instrument (DESI) imaging surveys to constrain the local
primordial non-Gaussianity parameter . Our sample comprises over 12
million targets, covering 14,000 square degrees of the sky, with redshifts in
the range 0.2< z < 1.35. We identify Galactic extinction, survey depth, and
astronomical seeing as the primary sources of systematic error, and employ
linear regression and artificial neural networks to alleviate non-cosmological
excess clustering on large scales. Our methods are tested against simulations
with and without and systematics, showing superior performance of the
neural network treatment. The neural network with a set of nine imaging
property maps passes our systematic null test criteria, and is chosen as the
fiducial treatment. Assuming the universality relation, we find =
34^+24(+50)_-44(-73) at 68%(95%) confidence. We apply a series of
robustness tests (e.g., cuts on imaging, declination, or scales used) that show
consistency in the obtained constraints. We study how the regression method
biases the measured angular power-spectrum and degrades the constraining
power. The use of the nine maps more than doubles the uncertainty compared to
using only the three primary maps in the regression. Our results thus motivate
the development of more efficient methods that avoid over-correction, protect
large-scale clustering information, and preserve constraining power.
Additionally, our results encourage further studies of with DESI
spectroscopic samples, where the inclusion of 3D clustering modes should help
separate imaging systematics and lessen the degradation in the
uncertainty.
MoreTranslated text
AI Read Science
Must-Reading Tree
Example
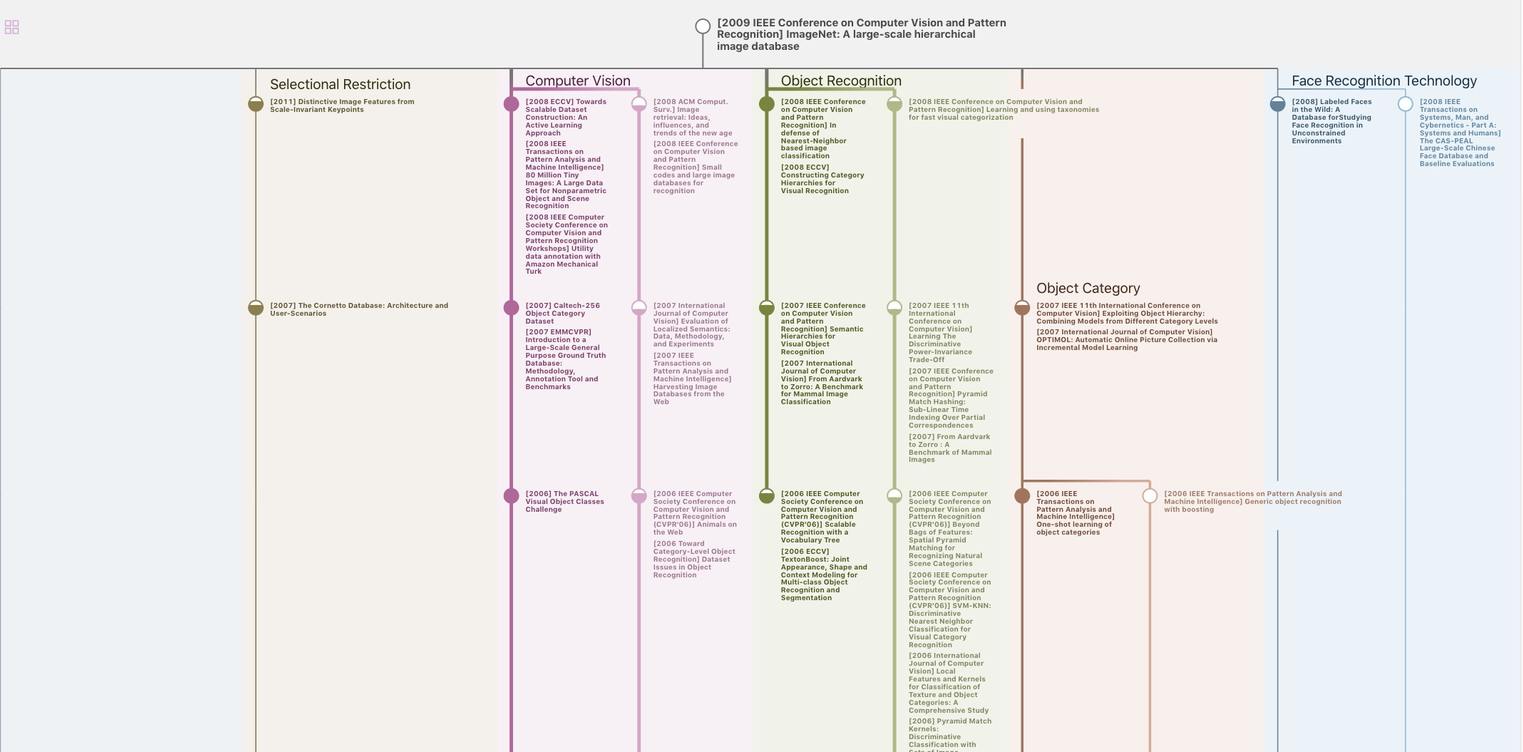
Generate MRT to find the research sequence of this paper
Chat Paper
Summary is being generated by the instructions you defined