Scalable variable selection for two-view learning tasks with projection operators
Machine Learning(2023)
摘要
In this paper we propose a novel variable selection method for two-view settings, or for vector-valued supervised learning problems. Our framework is able to handle extremely large scale selection tasks, where number of data samples could be even millions. In a nutshell, our method performs variable selection by iteratively selecting variables that are highly correlated with the output variables, but which are not correlated with the previously chosen variables. To measure the correlation, our method uses the concept of projection operators and their algebra. With the projection operators the relationship, correlation, between sets of input and output variables can also be expressed by kernel functions, thus nonlinear correlation models can be exploited as well. We experimentally validate our approach, showing on both synthetic and real data its scalability and the relevance of the selected features.
更多查看译文
关键词
Supervised variable selection,Vector-valued learning,Projection-valued measure,Reproducing kernel Hilbert space
AI 理解论文
溯源树
样例
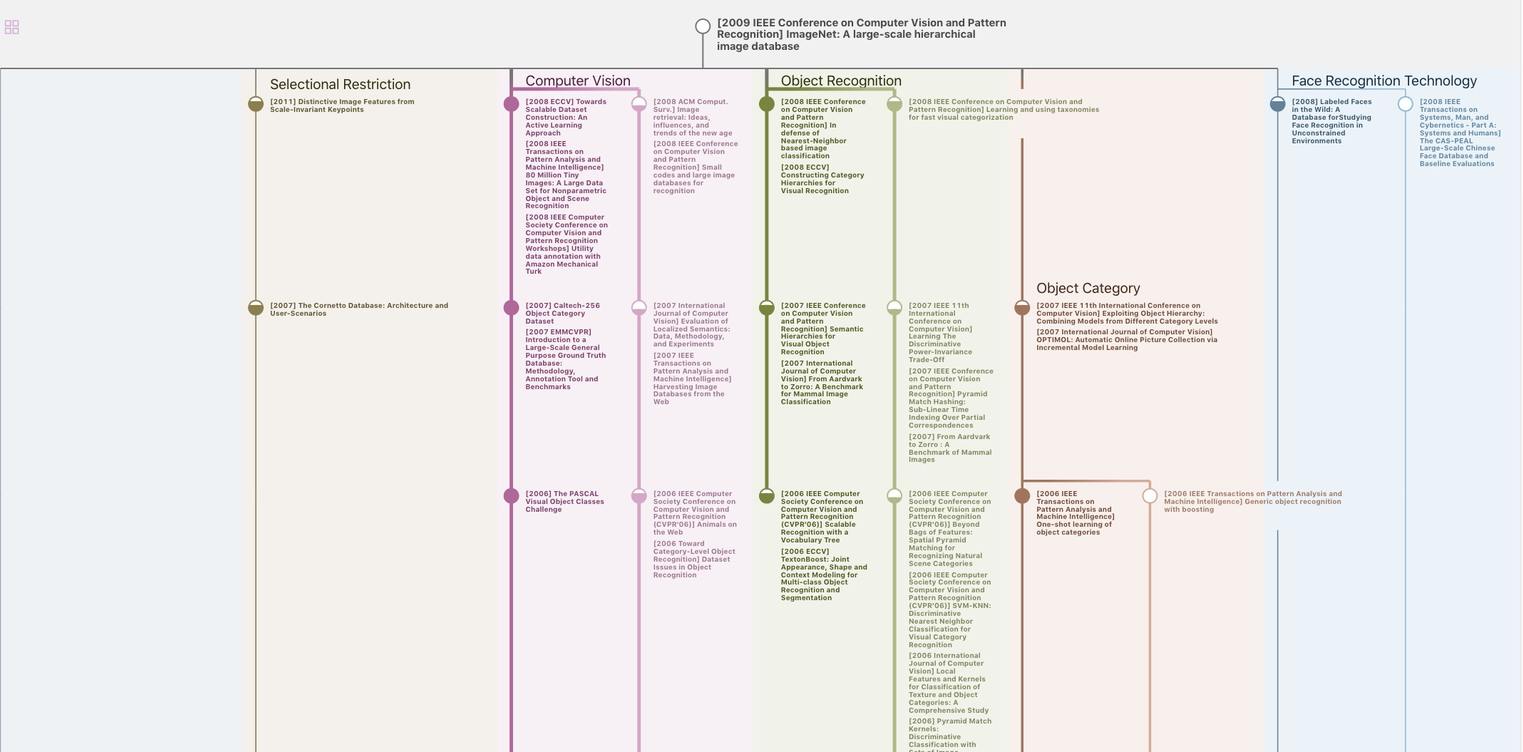
生成溯源树,研究论文发展脉络
Chat Paper
正在生成论文摘要