Mitigating the choice of the duration in DDMS models through a parametric link
arXiv (Cornell University)(2023)
摘要
One of the most important hyper-parameters in duration-dependent Markov-switching (DDMS) models is the duration of the hidden states. Because there is currently no procedure for estimating this duration or testing whether a given duration is appropriate for a given data set, an ad hoc duration choice must be heuristically justified. This is typically a difficult task and is likely the most delicate point of the modeling procedure, allowing for criticism and ultimately hindering the use of DDMS models. In this paper, we propose and examine a methodology that mitigates the choice of duration in DDMS models when forecasting is the goal. The idea is to use a parametric link instead of the usual fixed link when calculating transition probabilities. As a result, the model becomes more flexible and any potentially incorrect duration choice (i.e., misspecification) is compensated by the parameter in the link, yielding a likelihood and transition probabilities very close to the true ones while, at the same time, improving forecasting accuracy under misspecification. We evaluate the proposed approach in Monte Carlo simulations and using real data applications. Results indicate that the parametric link model outperforms the benchmark logit model, both in terms of in-sample estimation and out-of-sample forecasting, for both well-specified and misspecified duration values.
更多查看译文
关键词
ddms models,duration,parametric link
AI 理解论文
溯源树
样例
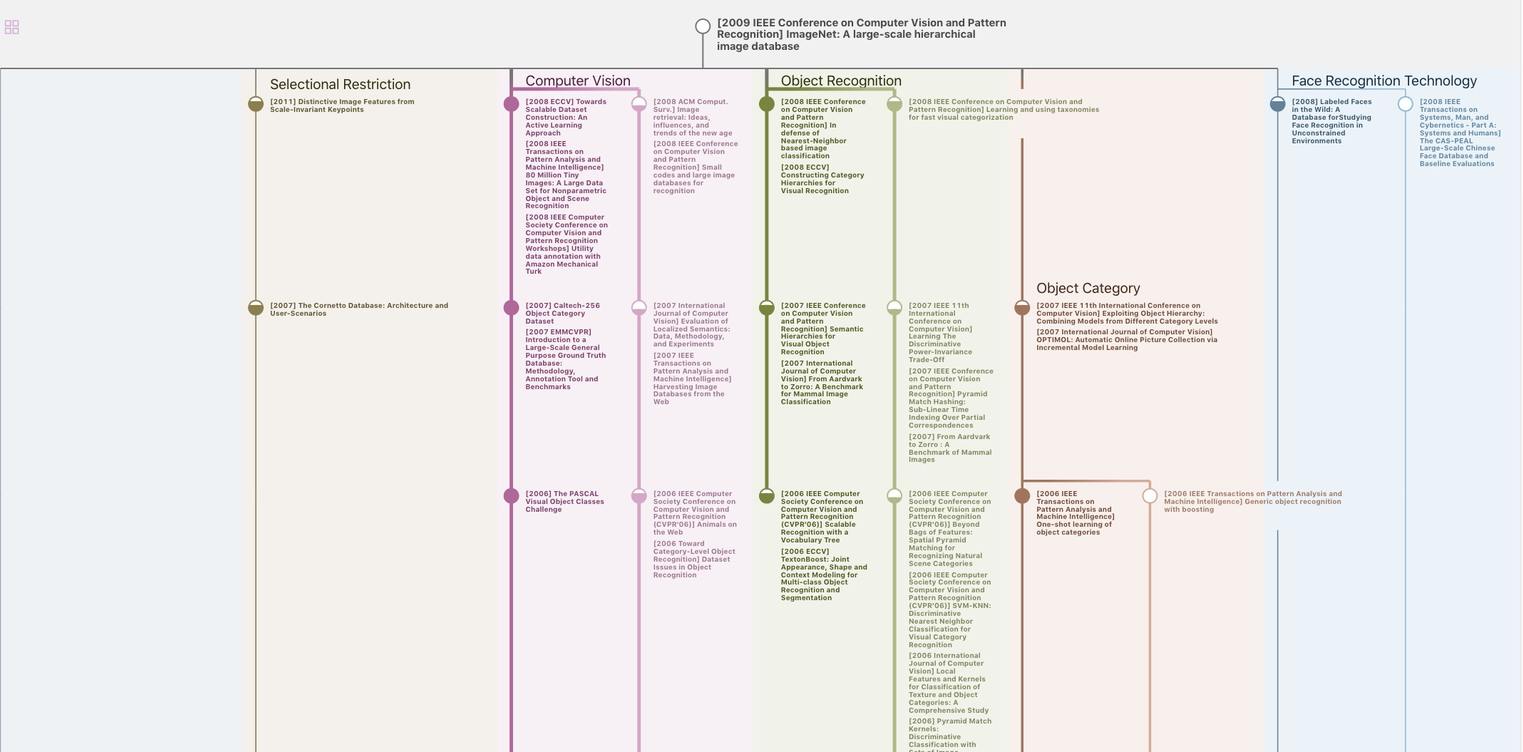
生成溯源树,研究论文发展脉络
Chat Paper
正在生成论文摘要