ERMDS: A obfuscation dataset for evaluating robustness of learning-based malware detection system
BenchCouncil Transactions on Benchmarks, Standards and Evaluations(2023)
摘要
Learning-based malware detection systems (LB-MDS) play a crucial role in defending computer systems from malicious attacks. Nevertheless, these systems can be vulnerable to various attacks, which can have significant consequences. Software obfuscation techniques can be used to modify the features of malware, thereby avoiding its classification as malicious by LB-MDS. However, existing portable executable (PE) malware datasets primarily use a single obfuscation technique, which LB-MDS has already learned, leading to a loss of their robustness evaluation ability. Therefore, creating a dataset with diverse features that were not observed during LB-MDS training has become the main challenge in evaluating the robustness of LB-MDS.
更多查看译文
关键词
Dataset,Malware detection system,Security,Machine learning,Adversarial malware
AI 理解论文
溯源树
样例
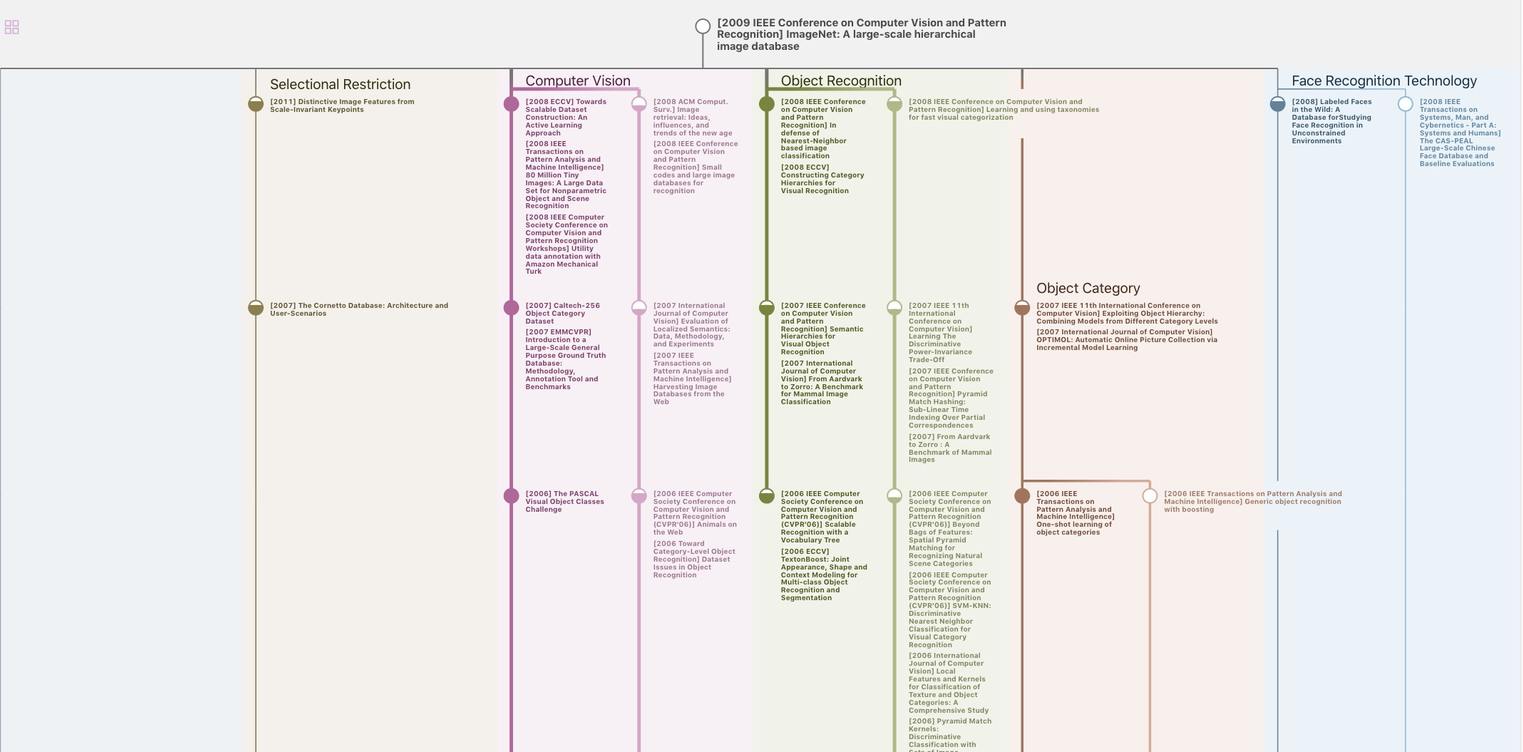
生成溯源树,研究论文发展脉络
Chat Paper
正在生成论文摘要