Improving latency performance trade-off in keyword spotting applications at the edge
2023 9th International Workshop on Advances in Sensors and Interfaces (IWASI)(2023)
摘要
Keyword Spotting (KWS) is handy in many innovative ambient intelligence applications, such as smart cities and home automation. While solving KWS on GP/GPUs has become a trivial task in recent years, many benefits arise when KWS applications run at the edge (e.g., privacy by design and infrastructure sustainability), where resources are limited. Hardware-aware scaling (HAS) is a novel paradigm that brings neural architectures to low-resource platforms. With HAS, it is possible to optimize neural architectures to fit on embedded platforms (e.g., microcontrollers) while maximizing the performance-complexity tradeoff and the performance-latency tradeoff. This paper shows how HAS, coupled with a neural network with appropriate scaling capabilities, can outperform architectures designed with neural architecture search techniques, such as MCUNet. Our method achieves 94.5% accuracy when classifying the 35 keywords in Google Speech Commands v2, with only 70 ms of latency and overall power consumption of less than 10 mJ.
更多查看译文
关键词
keyword spotting, tinyML, scalable backbone, IoT
AI 理解论文
溯源树
样例
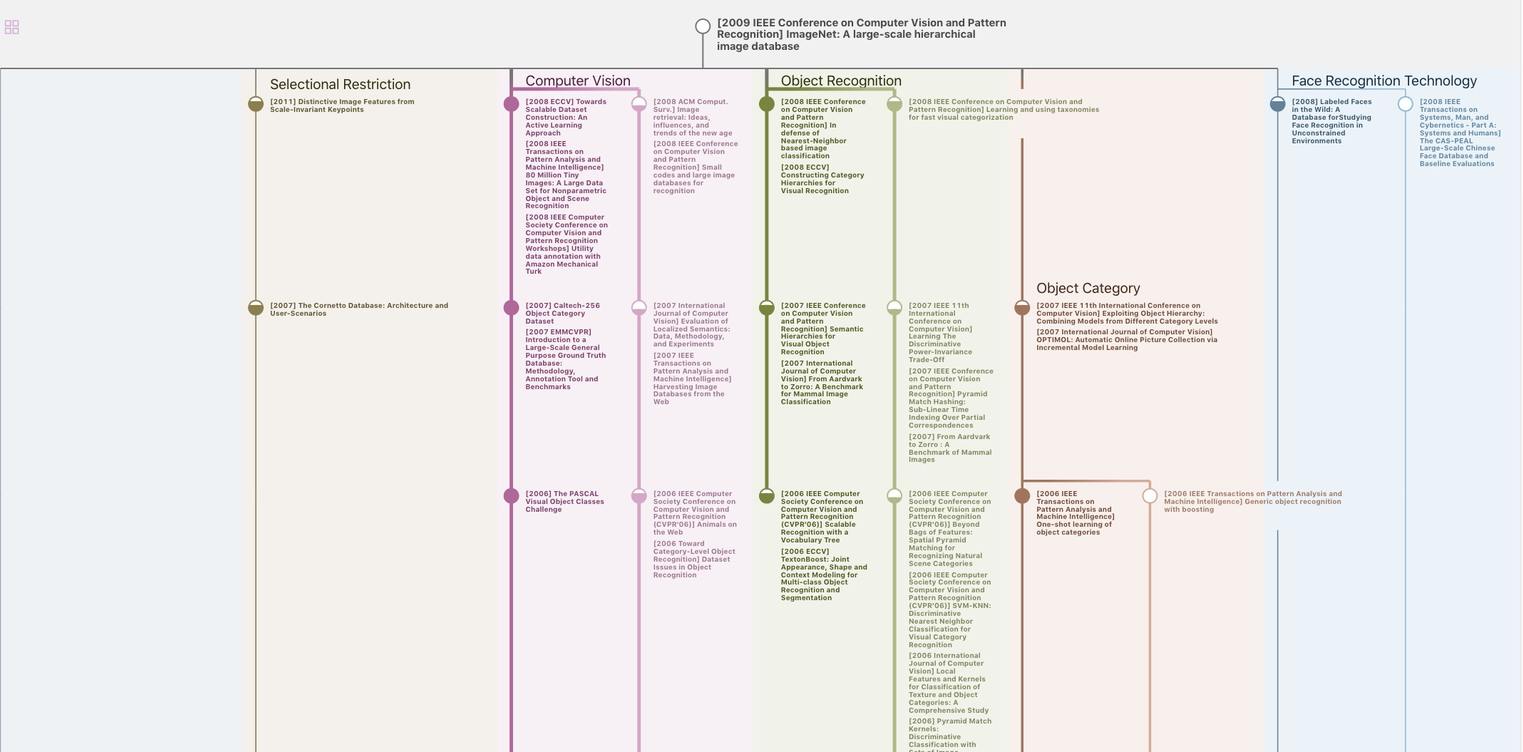
生成溯源树,研究论文发展脉络
Chat Paper
正在生成论文摘要