DrugGPT: A GPT-based Strategy for Designing Potential Ligands Targeting Specific Proteins
bioRxiv (Cold Spring Harbor Laboratory)(2023)
摘要
DrugGPT presents a ligand design strategy based on the autoregressive model, GPT, focusing on chemical space exploration and the discovery of ligands for specific proteins. Deep learning language models have shown significant potential in various domains including protein design and biomedical text analysis, providing strong support for the proposition of DrugGPT. In this study, we employ the DrugGPT model to learn a substantial amount of protein-ligand binding data, aiming to discover novel molecules that can bind with specific proteins. This strategy not only significantly improves the efficiency of ligand design but also offers a swift and effective avenue for the drug development process, bringing new possibilities to the pharmaceutical domain. In our research, we particularly optimized and trained the GPT-2 model to better adapt to the requirements of drug design. Given the characteristics of proteins and ligands, we redesigned the tokenizer using the BPE algorithm, abandoned the original tokenizer, and trained the GPT-2 model from scratch. This improvement enables DrugGPT to more accurately capture and understand the structural information and chemical rules of drug molecules. It also enhances its comprehension of binding information between proteins and ligands, thereby generating potentially active drug candidate molecules. Theoretically, DrugGPT has significant advantages. During the model training process, DrugGPT aims to maximize the conditional probability and employs the back-propagation algorithm for training, making the training process more stable and avoiding the Mode Collapse problem that may occur in Generative Adversarial Networks in drug design. Furthermore, the design philosophy of DrugGPT endows it with strong generalization capabilities, giving it the potential to adapt to different tasks. In conclusion, DrugGPT provides a forward-thinking and practical new approach to ligand design. By optimizing the tokenizer and retraining the GPT-2 model, the ligand design process becomes more direct and efficient. This not only reflects the theoretical advantages of DrugGPT but also reveals its potential applications in the drug development process, thereby opening new perspectives and possibilities in the pharmaceutical field.
### Competing Interest Statement
S.H., Y.X., Y.L., C.G., X.S. and X.W. have filed a patent application (202310711607.X) relating to the autoregressive model-based drug design method in the name of the First Affiliated Hospital of Xi'an Jiaotong University School of Medicine.
更多查看译文
关键词
ligands,proteins,gpt-based
AI 理解论文
溯源树
样例
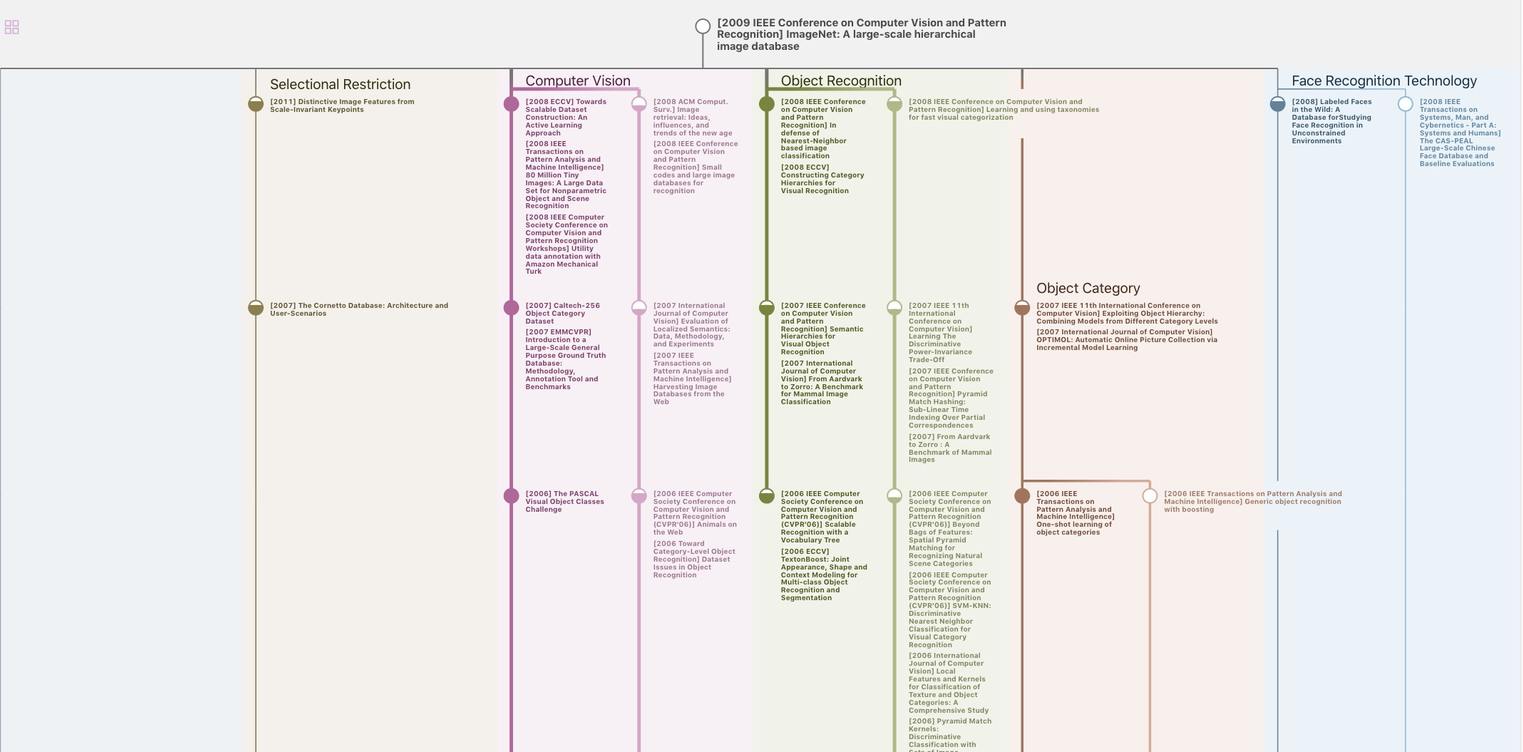
生成溯源树,研究论文发展脉络
Chat Paper
正在生成论文摘要