Covering Hierarchical Dirichlet Mixture Models on binary data to enhance genomic stratifications in Onco-Hematology
PLOS Computational Biology(2023)
摘要
Onco-hematological studies are increasingly adopting statistical mixture models to support the advancement of the genetically-driven classification systems for blood cancer. Targeting enhanced patients stratification based on the sole role of molecular biology attracted much interest and contributes to bring personalized medicine closer to reality. In particular, Dirichlet processes have become the preferred method to approach the fit of mixture models. Usually, the multinomial distribution is at the core of such models. However, despite their advanced statistical formalism, these processes are not to be considered black box techniques and a better understanding of their working mechanisms enables to improve their employment and explainability. Focused on genomic data in Acute Myeloid Leukemia, this work unfolds the driving factors and rationale of the Hierarchical Dirichlet Mixture Models of multinomials on binary data. In addition, we introduce a novel approach to perform accurate patients clustering via multinomials based on statistical considerations. The newly reported adoption of the Multivariate Fisher's Non-Central Hypergeometric distributions reveals promising results and outperformed the multinomials in clustering both on simulated and real onco-hematological data.
### Competing Interest Statement
The authors have declared no competing interest.
更多查看译文
AI 理解论文
溯源树
样例
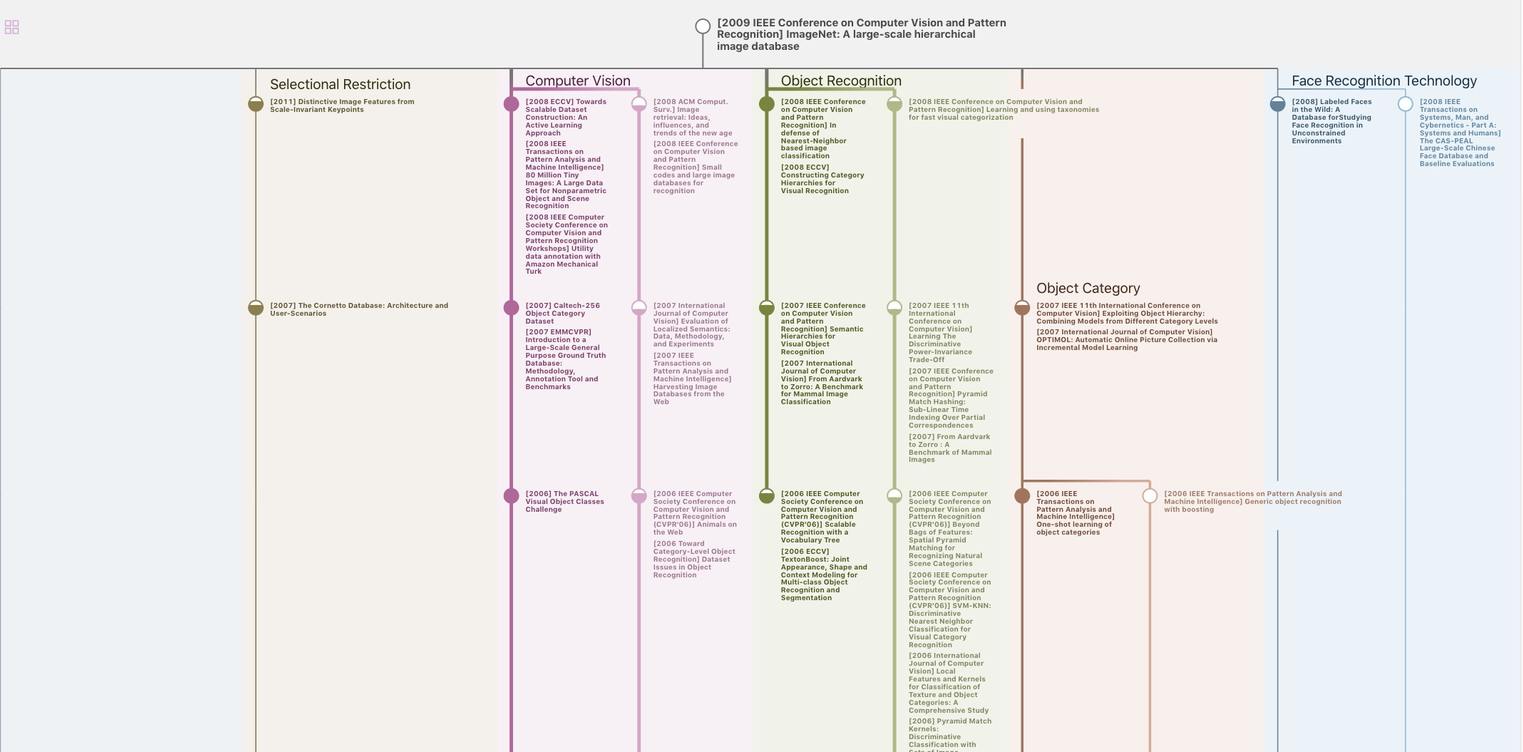
生成溯源树,研究论文发展脉络
Chat Paper
正在生成论文摘要