Speech recognition from MEG data using covariance filters
bioRxiv (Cold Spring Harbor Laboratory)(2023)
摘要
Speech recognition from EEG and MEG data is the first step in the development of BCI and AI systems for further use in the decoding of inner speech. Great achievements in this direction have been made with the use of ECoG and stereo-EEG. At the same time, there are few works on this topic on the analysis of data obtained by nonin-vasive methods of brain activity registration. Our approach is based on the evaluation of connections in the sensor space with the extraction of the MEG connectivity pattern specific to a given segment of speech. We tested our method on 7 subjects. In all cases, our processing pipeline was sufficiently reliable and worked either without recognition errors or with few errors. After ”training” the algorithm is able to recognize a fragment of spoken speech in a single presentation. For recognition, we used MEG recording segments of 50-1200 ms from the beginning of the word. A segment of at least 600 ms was required for high-quality recognition. Intervals longer than 1200 ms degraded the quality of recognition. Band-pass filtering of MEG showed that the quality of recognition is higher when using the gamma frequency range compared to the low-frequency range of the analyzed signal.
### Competing Interest Statement
The authors have declared no competing interest.
更多查看译文
关键词
meg data,covariance filters,speech recognition
AI 理解论文
溯源树
样例
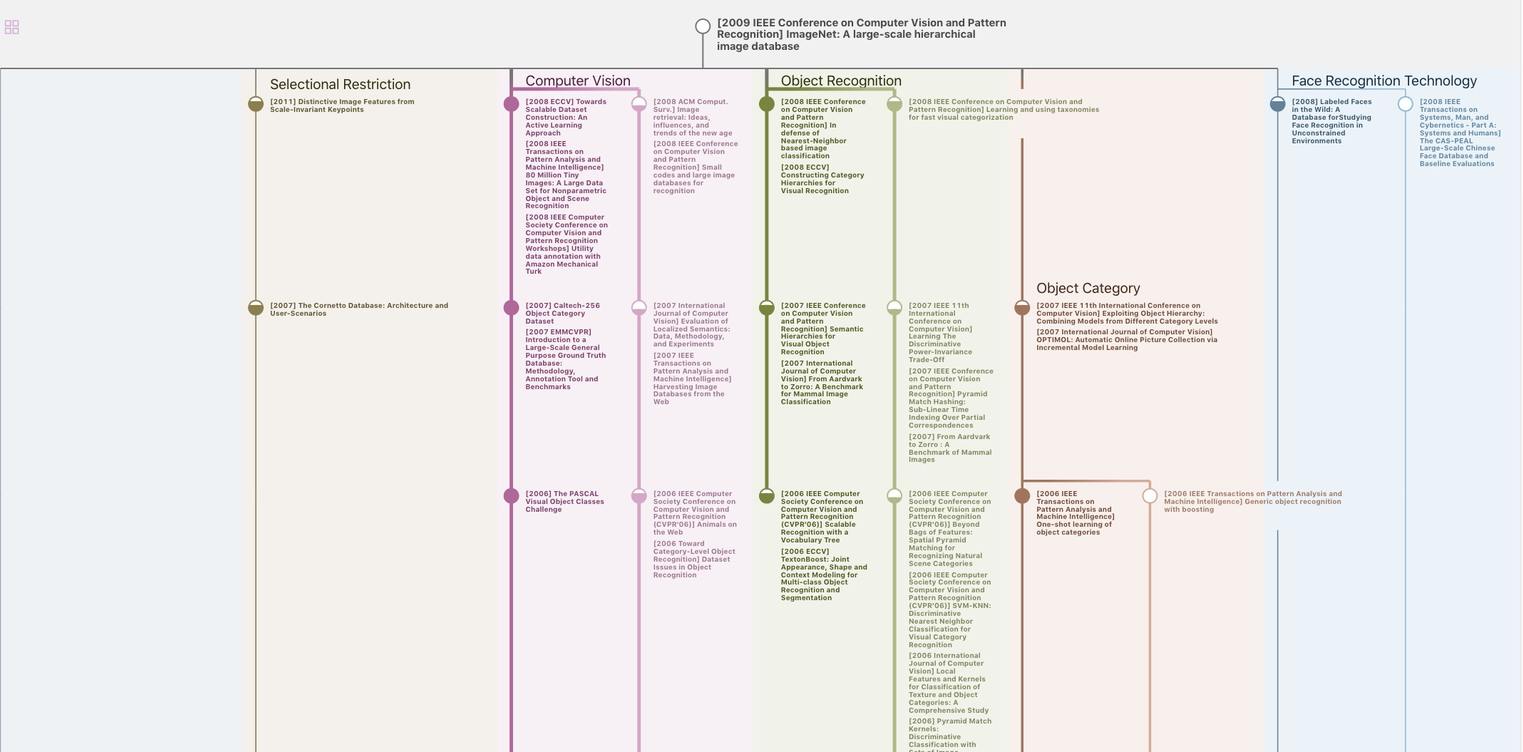
生成溯源树,研究论文发展脉络
Chat Paper
正在生成论文摘要