Identification of tumor-specific MHC ligands through improved biochemical isolation and incorporation of machine learning
bioRxiv (Cold Spring Harbor Laboratory)(2023)
摘要
Isolation of MHC ligands and subsequent analysis by mass spectrometry is considered the gold standard for defining targets for TCR-T immunotherapies. However, as many targets of high tumor-specificity are only presented at low abundance on the cell surface of tumor cells, the efficient isolation of these peptides is crucial for their successful detection. Here, we demonstrate how different isolation strategies, which consider hydrophobicity and post-translational modifications, can improve the detection of MHC ligands, including cysteinylated MHC ligands from cancer germline antigens or point-mutated neoepitopes. Furthermore, we developed a novel MHC class I ligand prediction algorithm (ARDisplay-I) that outperforms the current state-of-the-art and facilitates the assignment of peptides to the correct MHC allele. The model has other applications, such as the identification of additional MHC ligands not detected from mass spectrometry or determining whether the MHC ligands can be presented on the cell surface via MHC alleles not included in the study. The implementation of these strategies can augment the development of T cell receptor-based therapies (i.a. TIL[1][1]-derived T cells, genetically engineered T cells expressing tumor recognizing receptors or TCR-mimic antibodies) by facilitating the identification of novel immunotherapy targets and by enriching the resources available in the field of computational immunology.
Significance: This study demonstrates how the isolation of different tumor-specific MHC ligands can be optimized when considering their hydrophobicity and post-translational modification status. Additionally, we developed a novel machine-learning model for the probability prediction of the MHC ligands’ presentation on the cell surface. The algorithm can assign these MHC ligands to their respective MHC alleles which is essential for the design of TCR-T immunotherapies.
### Competing Interest Statement
MGK is a consultant to Ardigen.
[1]: #fn-3
更多查看译文
关键词
biochemical isolation,machine learning,tumor-specific
AI 理解论文
溯源树
样例
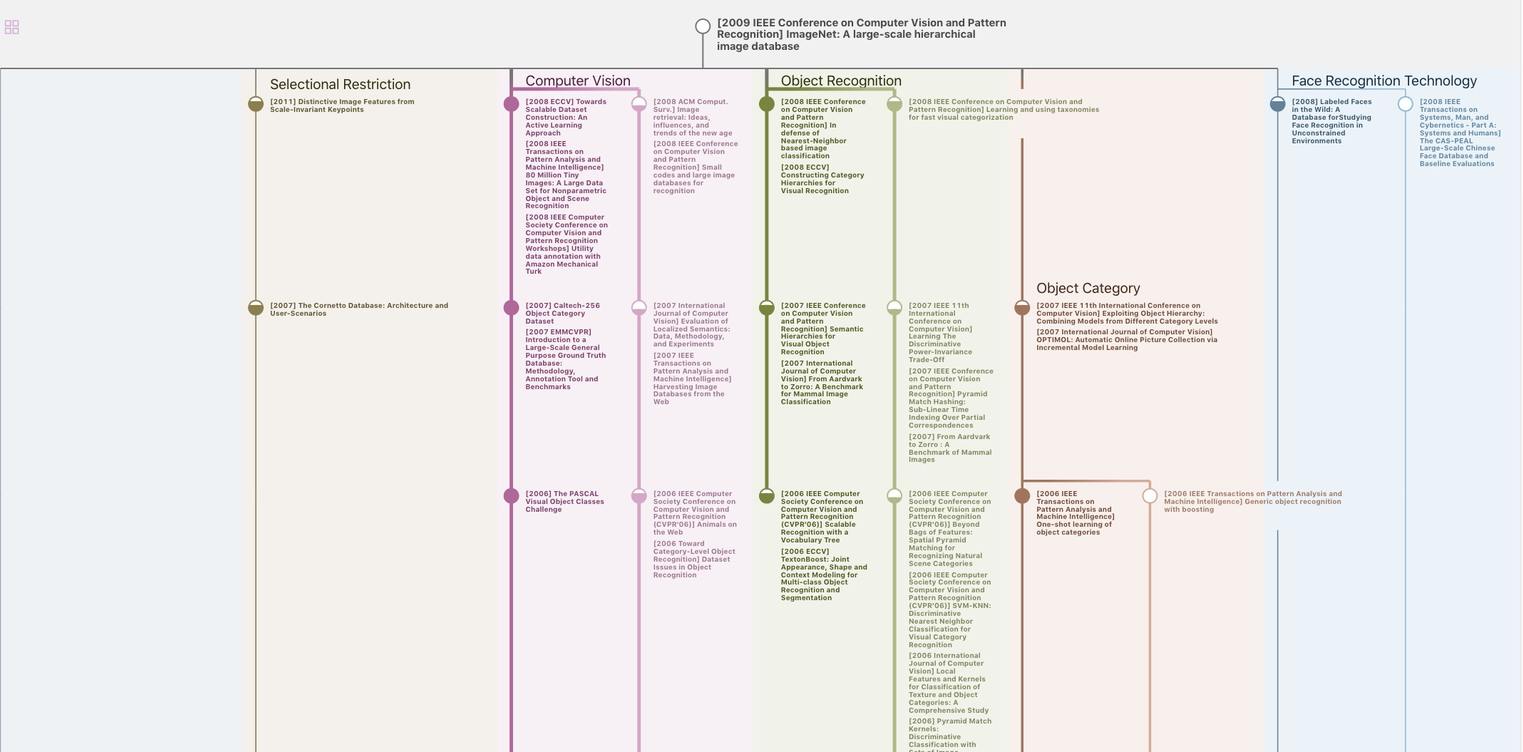
生成溯源树,研究论文发展脉络
Chat Paper
正在生成论文摘要