BertNDA: A Model Based on Graph-Bert and Multi-Scale Information Fusion for ncRNA-Disease Association Prediction
IEEE JOURNAL OF BIOMEDICAL AND HEALTH INFORMATICS(2023)
摘要
Non-coding RNAs (ncRNAs) are a class of RNA molecules that lack the ability to encode proteins in human cells, but play crucial roles in various biological process. Understanding the interactions between different ncRNAs and their impact on diseases can significantly contribute to diagnosis, prevention, and treatment of diseases. However, predicting tertiary interactions between ncRNAs and diseases based on structural information in multiple scales remains a challenging task. To address this challenge, we propose a method called BertNDA, aiming to predict potential relationships between miRNAs, lncRNAs, and diseases. The framework identifies the local information through connectionless subgraph, which aggregate neighbor nodes' feature. And global information is extracted by leveraging Laplace transform of graph structures and WL (Weisfeiler-Lehman) absolute role coding. Additionally, an EMLP (Element-wise MLP) structure is designed to fuse pairwise global information. The transformer-encoder is employed as the backbone of our approach, followed by a prediction-layer to output the final correlation score. Extensive experiments demonstrate that BertNDA outperforms state-of-the-art methods in prediction assignment and exhibits significant potential for various biological applications. Moreover, we develop an online prediction platform that incorporates the prediction model, providing users with an intuitive and interactive experience. Overall, our model offers an efficient, accurate, and comprehensive tool for predicting tertiary associations between ncRNAs and diseases.
更多查看译文
关键词
Transformer encoder,multi-scale feature extraction and fusion,ncRNA-disease tertiary interactions
AI 理解论文
溯源树
样例
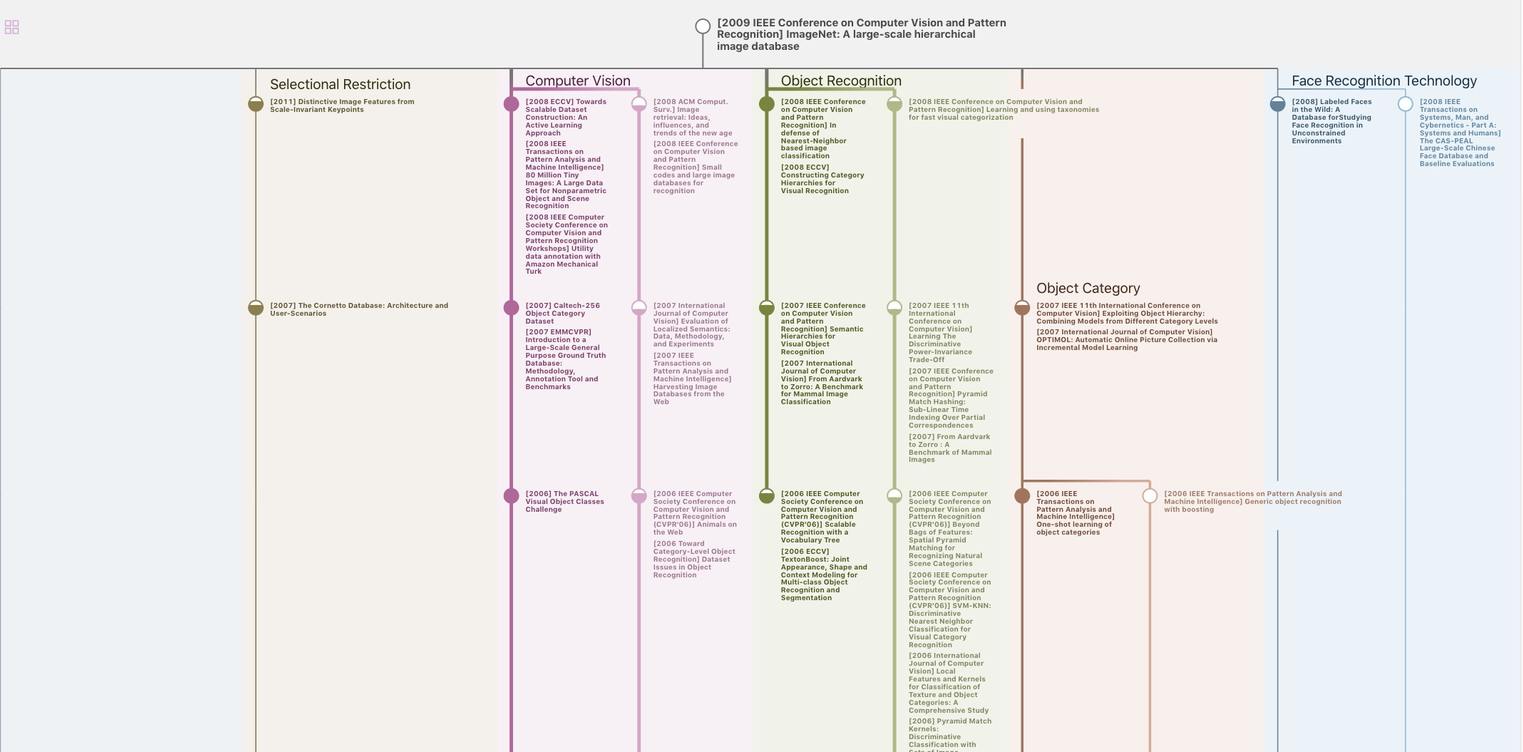
生成溯源树,研究论文发展脉络
Chat Paper
正在生成论文摘要