Towards Computing Attributions for Dimensionality Reduction Techniques
Bioinformatics advances(2023)
摘要
We describe the problem of computing local feature attributions for dimensionality reduction methods. We use one such method that is well established within the context of supervised classification – using the gradients of target outputs with respect to the inputs – on the popular dimensionality reduction technique t-SNE, widely used in analyses of biological data. We provide an efficient implementation for the gradient computation for this dimensionality reduction technique. We show that our explanations identify significant features using novel validation methodology; using synthetic datasets and the popular MNIST benchmark dataset. We then demonstrate the practical utility of our algorithm by showing that it can produce explanations that agree with domain knowledge on a SARS-CoV-2 sequence dataset. Throughout, we provide a road map so that similar explanation methods could be applied to other dimensionality reduction techniques to rigorously analyze biological datasets.
### Competing Interest Statement
The authors have declared no competing interest.
更多查看译文
关键词
computing attributions
AI 理解论文
溯源树
样例
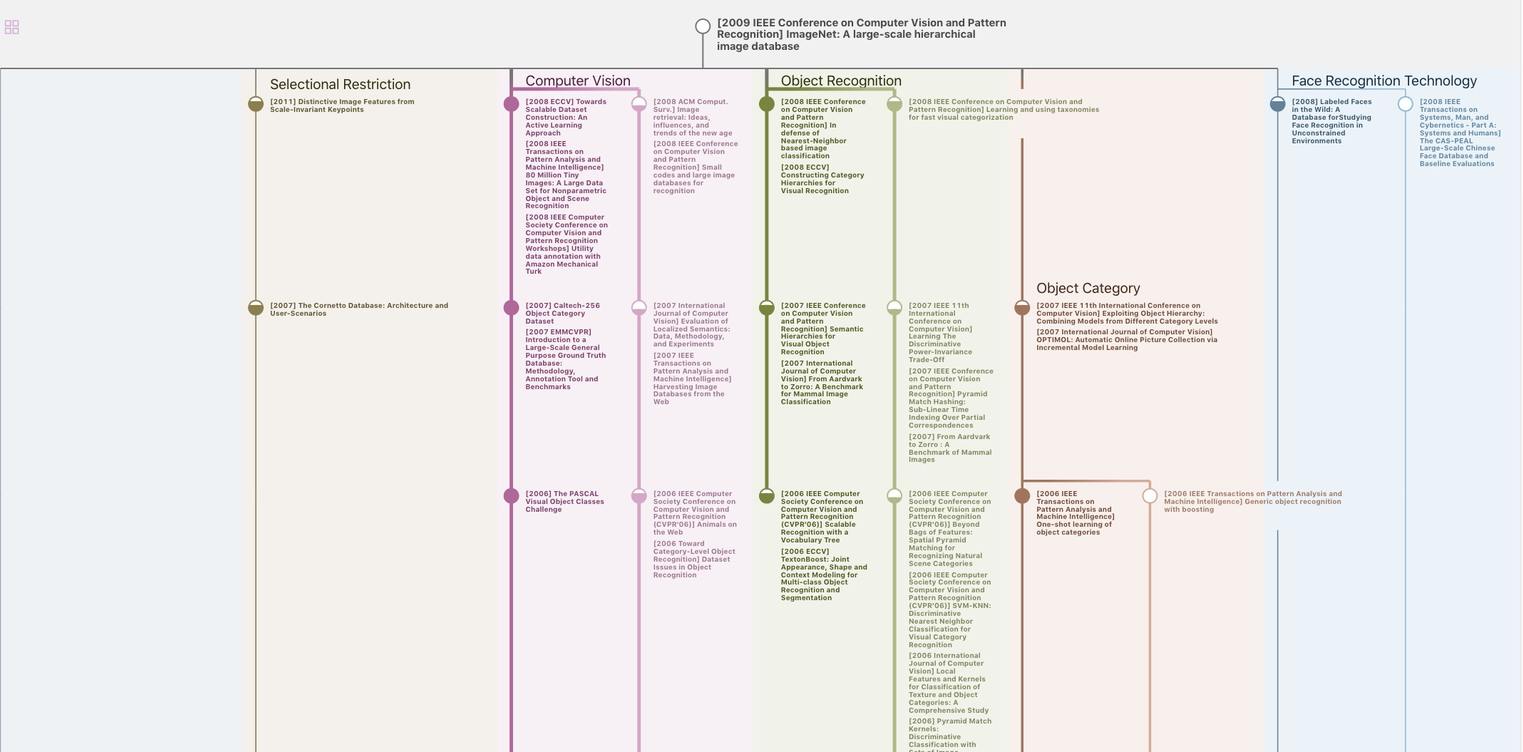
生成溯源树,研究论文发展脉络
Chat Paper
正在生成论文摘要