Variance Analysis of LC-MS Experimental Factors and Their Impact on Machine Learning
biorxiv(2023)
摘要
Background Machine learning (ML) technologies, especially deep learning (DL), have gained increasing attention in predictive mass spectrometry (MS) for enhancing the data processing pipeline from raw data analysis to end-user predictions and re-scoring. ML models need large-scale datasets for training and re-purposing, which can be obtained from a range of public data repositories. However, applying ML to public MS datasets on larger scales is challenging, as they vary widely in terms of data acquisition methods, biological systems, and experimental designs.
Results We aim to facilitate ML efforts in MS data by conducting a systematic analysis of the potential sources of variance in public MS repositories. We also examine how these factors affect ML performance and perform a comprehensive transfer learning to evaluate the benefits of current best practice methods in the field for transfer learning.
Conclusions Our findings show significantly higher levels of homogeneity within a project than between projects, which indicates that it’s important to construct datasets most closely resembling future test cases, as transferability is severely limited for unseen datasets. We also found that transfer learning, although it did increase model performance, did not increase model performance compared to a non-pre-trained model.
### Competing Interest Statement
The authors have declared no competing interest.
* ML
: Machine Learning
DL
: Deep Learning
MS
: Mass Spectrometry
LC-MS or MS1
: Liquid Chromatography-Mass Spectrometry
LC-MS/MS, MS/MS or MS2
: Tandem mass-spectrometry
m/z
: Mass to charge ratio
NCE
: Normalized Collision Energy
PTM
: Post-translational modification
CID
: Collision induced dissociation
HCD
: high-energy C-trap dissociation
ETD
: electron-transfer dissociation
ETciD
: electron-transfer and collision-induced dissociation
EThcD
: electron-transfer and higher-energy collision dissociation
PX
: ProteomeXchange
更多查看译文
AI 理解论文
溯源树
样例
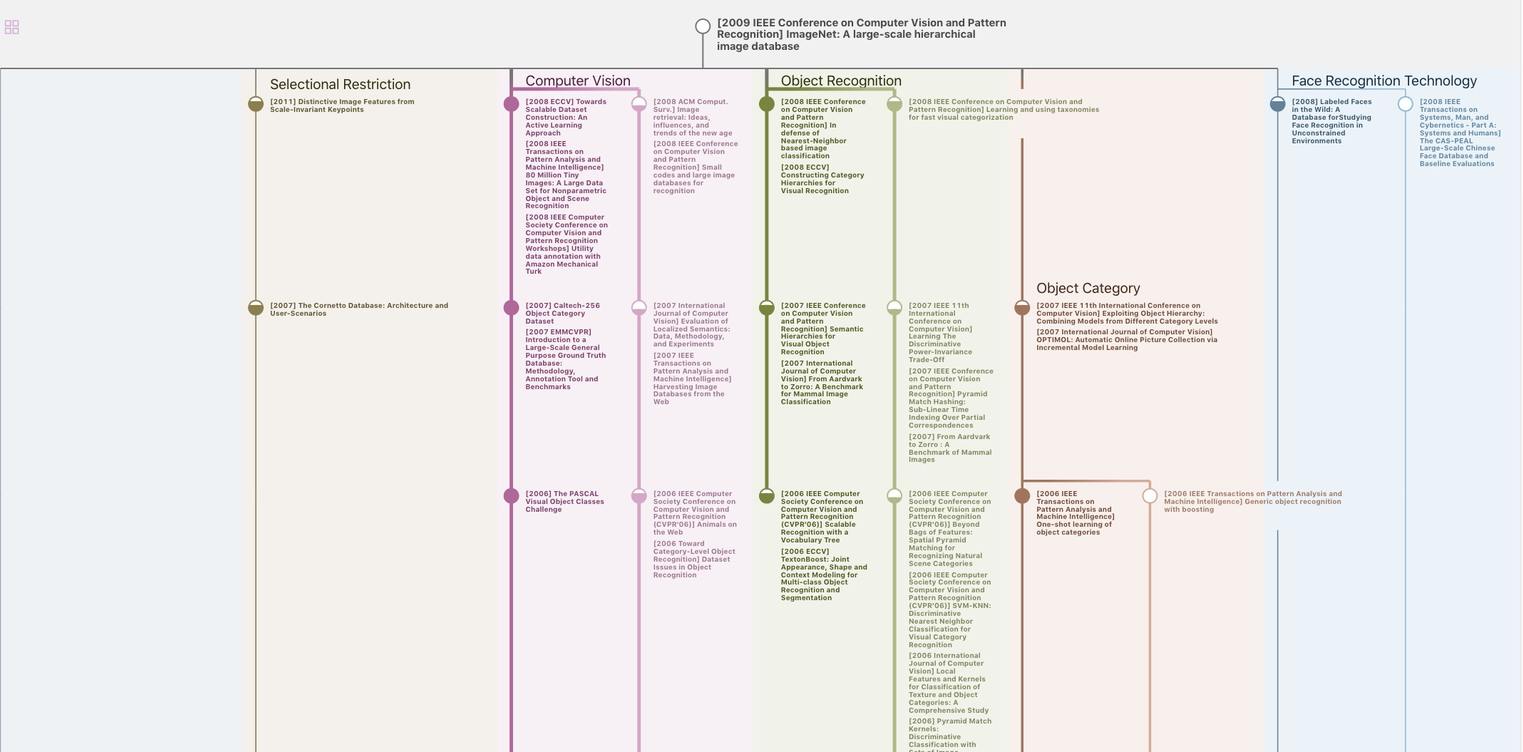
生成溯源树,研究论文发展脉络
Chat Paper
正在生成论文摘要