Survival Analysis on Rare Events Using Group-Regularized Multi-Response Cox Regression
bioRxiv (Cold Spring Harbor Laboratory)(2020)
摘要
We propose a Sparse-Group regularized Cox regression method to improve the prediction performance of large-scale and high-dimensional survival data with few observed events. Our approach is applicable when there is one or more other survival responses that 1. has a large number of observed events; 2. share a common set of associated predictors with the rare event response. This scenario is common in the UK Biobank ([Sudlow et al. 2015][1]) dataset where records for a large number of common and rare diseases of the same set of individuals are available. By analyzing these responses together, we hope to achieve higher prediction performance than when they are analyzed individually. To make this approach practical for large-scale data, we developed an accelerated proximal gradient optimization algorithm as well as a screening procedure inspired by [Qian et al. (2019)][2]. We provide a software implementation of the proposed method and demonstrate its efficacy through simulations and applications to UK Biobank data.
### Competing Interest Statement
The authors have declared no competing interest.
[1]: #ref-13
[2]: #ref-10
更多查看译文
关键词
cox regression,rare events,survival,group-regularized,multi-response
AI 理解论文
溯源树
样例
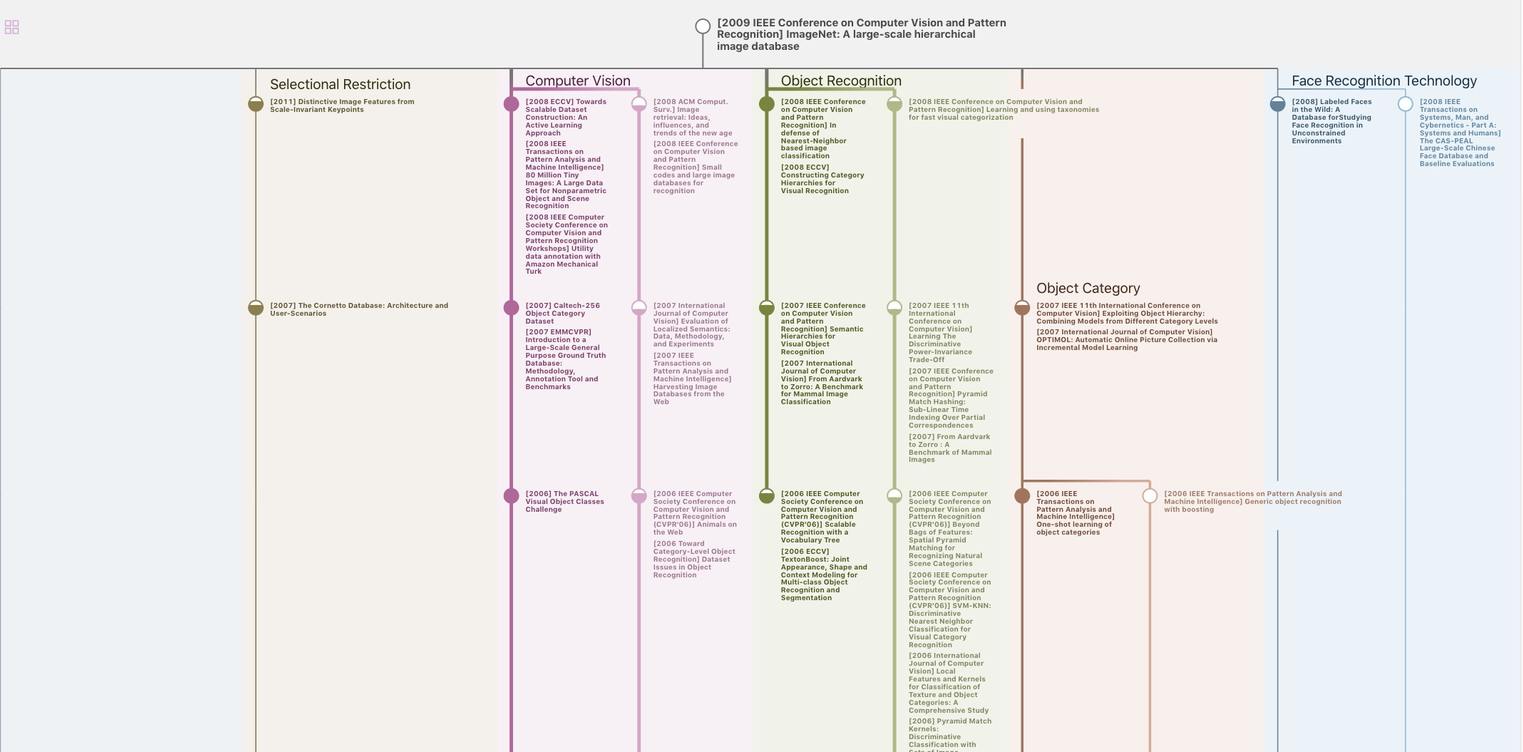
生成溯源树,研究论文发展脉络
Chat Paper
正在生成论文摘要