Statistically bias-corrected and downscaled climate models underestimate the severity of U.S. maize yield shocks
crossref(2021)
摘要
Abstract Efforts to understand and quantify how a changing climate can impact agriculture often rely on bias-corrected and downscaled climate information, making it important to quantify potential biases of this approach. Previous studies typically focus their uncertainty analyses on climatic variables and are silent on how these uncertainties propagate into human systems through their subsequent incorporation into econometric models. Here, we use a multi-model ensemble of statistically downscaled and bias-corrected climate models, as well as the corresponding CMIP5 parent models, to analyze uncertainty surrounding annual maize yield variability in the United States. We find that the CMIP5 models considerably overestimate historical yield variability while the bias-corrected and downscaled versions underestimate the largest historically observed yield shocks. We also find large differences in projected yields and other decision-relevant metrics throughout this century, leaving stakeholders with modeling choices that require navigating trade-offs in resolution, historical accuracy, and projection confidence.
更多查看译文
AI 理解论文
溯源树
样例
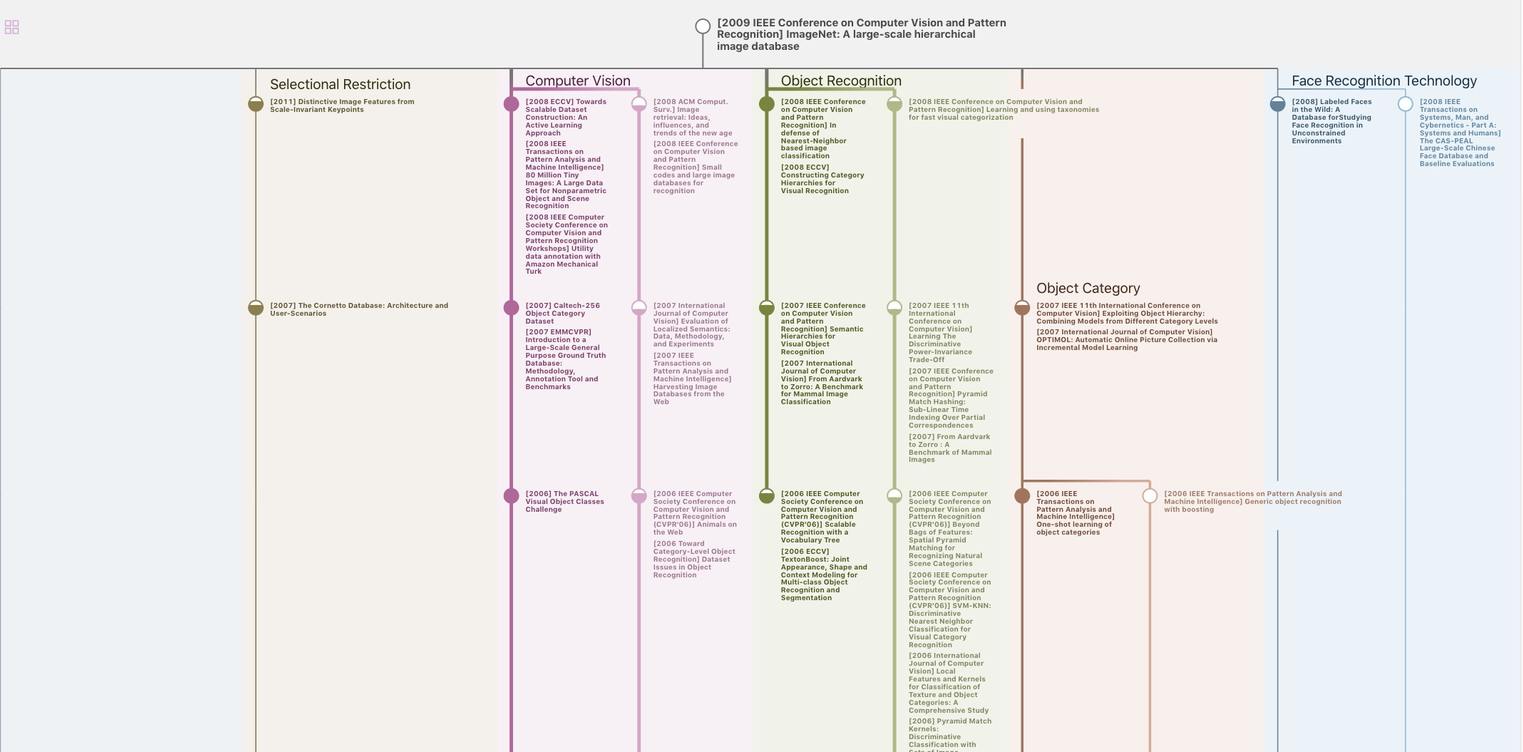
生成溯源树,研究论文发展脉络
Chat Paper
正在生成论文摘要