Deep learning analysis of drug-induced ECG changes to inform arrhythmia risk and improve diagnosis of congenital long QT syndrome
Research Square (Research Square)(2021)
摘要
Abstract Congenital or drug-induced long-QT syndromes can cause Torsade-de-Pointes (TdP), a life-threatening ventricular arrhythmia. The current strategy to identify individuals at high risk of TdP consists on measuring the QT duration on the electrocardiogram (ECG), shown to provide limited information. We propose an original method, including training deep neural networks to recognize ECG alterations induced by QT-prolonging drugs, as a comprehensive evaluation of TdP risk. These models accurately detected patients taking QT prolonging drugs during ECGs, while discriminating for the presence and type of congenital long-QT. Moreover, they enhanced prediction of drug-induced TdP events in addition to QT measurement. Analyses of these models revealed footprints of the torsadogenic risk and clinically relevant patient stratification.
更多查看译文
关键词
congenital long qt syndrome,arrhythmia,ecg changes,deep learning,drug-induced
AI 理解论文
溯源树
样例
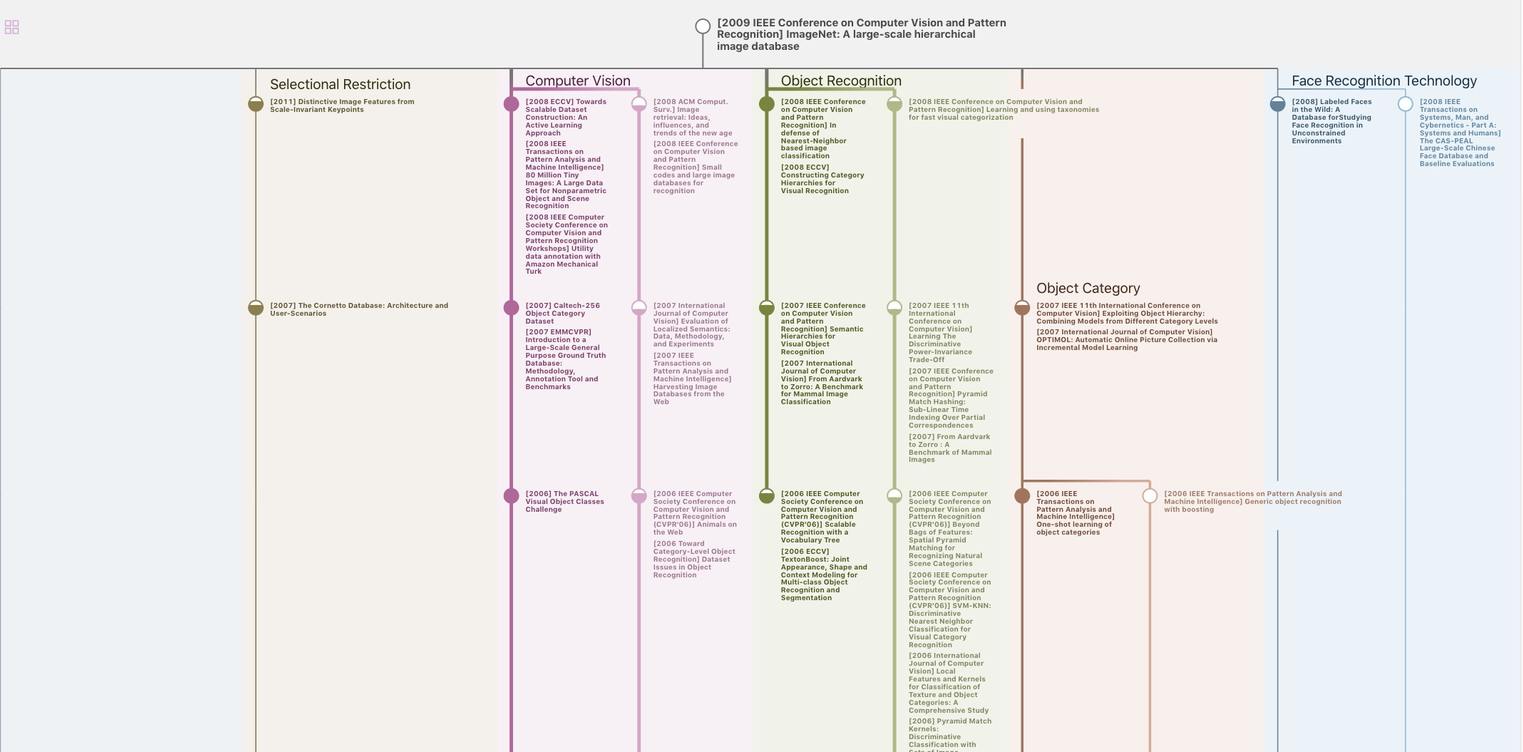
生成溯源树,研究论文发展脉络
Chat Paper
正在生成论文摘要